Data-Enabled Policy Optimization for Direct Adaptive Learning of the LQR
CoRR(2024)
摘要
Direct data-driven design methods for the linear quadratic regulator (LQR)
mainly use offline or episodic data batches, and their online adaptation has
been acknowledged as an open problem. In this paper, we propose a direct
adaptive method to learn the LQR from online closed-loop data. First, we
propose a new policy parameterization based on the sample covariance to
formulate a direct data-driven LQR problem, which is shown to be equivalent to
the certainty-equivalence LQR with optimal non-asymptotic guarantees. Second,
we design a novel data-enabled policy optimization (DeePO) method to directly
update the policy, where the gradient is explicitly computed using only a batch
of persistently exciting (PE) data. Third, we establish its global convergence
via a projected gradient dominance property. Importantly, we efficiently use
DeePO to adaptively learn the LQR by performing only one-step projected
gradient descent per sample of the closed-loop system, which also leads to an
explicit recursive update of the policy. Under PE inputs and for bounded noise,
we show that the average regret of the LQR cost is upper-bounded by two terms
signifying a sublinear decrease in time 𝒪(1/√(T)) plus a bias
scaling inversely with signal-to-noise ratio (SNR), which are independent of
the noise statistics. Finally, we perform simulations to validate the
theoretical results and demonstrate the computational and sample efficiency of
our method.
更多查看译文
AI 理解论文
溯源树
样例
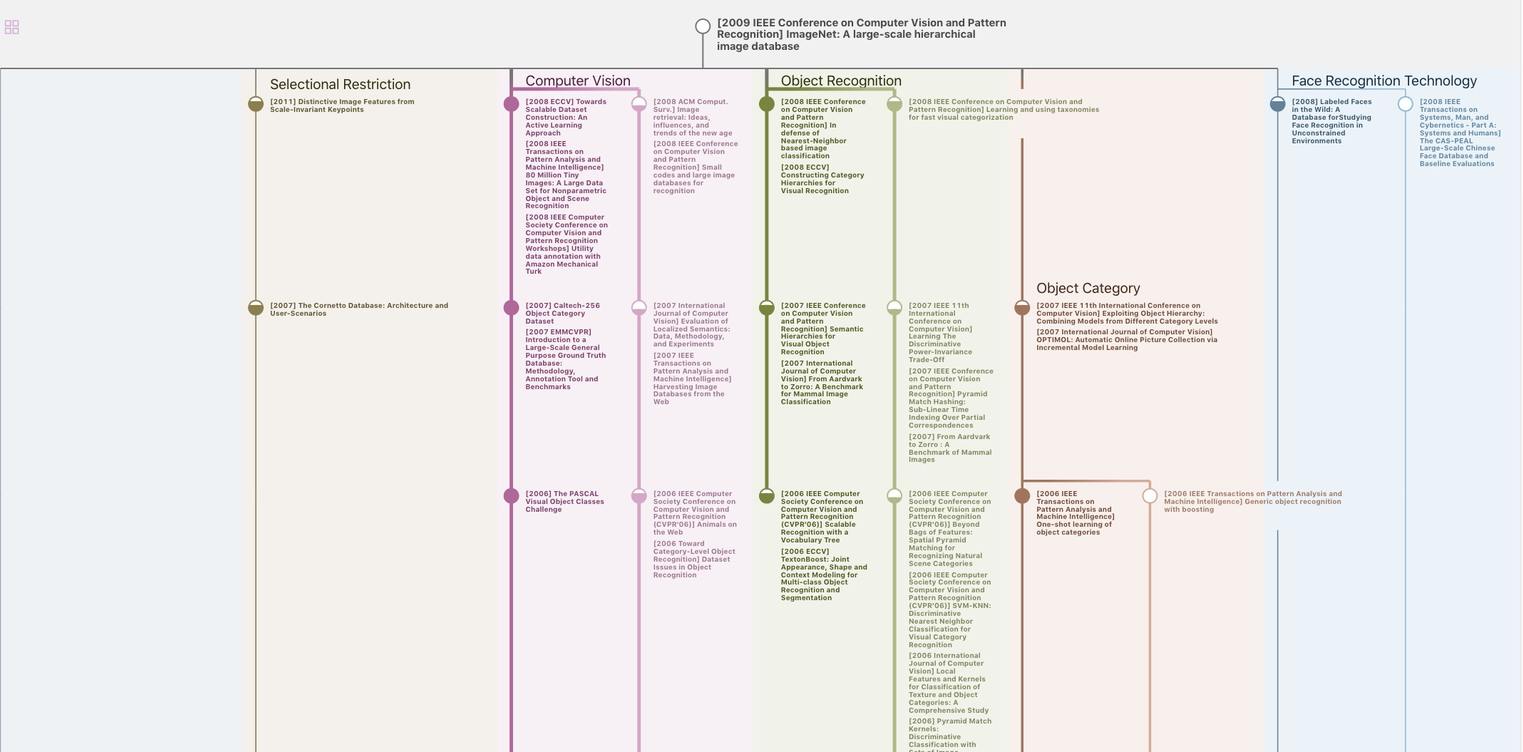
生成溯源树,研究论文发展脉络
Chat Paper
正在生成论文摘要