C ^2 Net: content-dependent and -independent cross-attention network for anomaly detection in videos
Applied Intelligence(2024)
摘要
nomaly detection in videos is a challenging issue that identifies unexpected occurrences if normal training examples are provided. Most approaches focus on designing elaborate models to mine normal patterns using content-dependent information. Nevertheless, content-dependent information, which includes various details, would have both positive and negative effects. The abundant details around abnormal events are conducive to anomaly detection by producing obvious errors, while complex details around normal events may lead to the erroneous detection of anomalies in challenging normal samples. To alleviate the problem of challenging normal samples, we propose a content-independent image without complex details for all normal samples during the training phase. It represents the pseudo label of regular patterns as a normal supporter. Accordingly, a content-dependent and -independent cross-attention network, termed C ^2 Net, is introduced by jointly considering the advantage of content-dependent information and content-independent pseudo-label simultaneously. C ^2 Net employs a fusion-first-then-separation strategy, where it injects the content-independent pseudo-label supporter into content-dependent frames using an auto-encoder network. It then reconstructs the content-independent pseudo-label supporter and the content-dependent frame respectively using siamese sub-networks. Additionally, a novel cross-attention module is designed between the siamese sub-networks to separate the information of the content-independent pseudo-label supporter and the content-dependent frame. The experimental results on three publicly outdoor datasets and a publicly indoor dataset about cognitive disorder rehabilitation assessment verify the effectiveness of C ^2 Net.
更多查看译文
关键词
Anomaly detection,Cross-attention,Pseudo label,Mutual learning
AI 理解论文
溯源树
样例
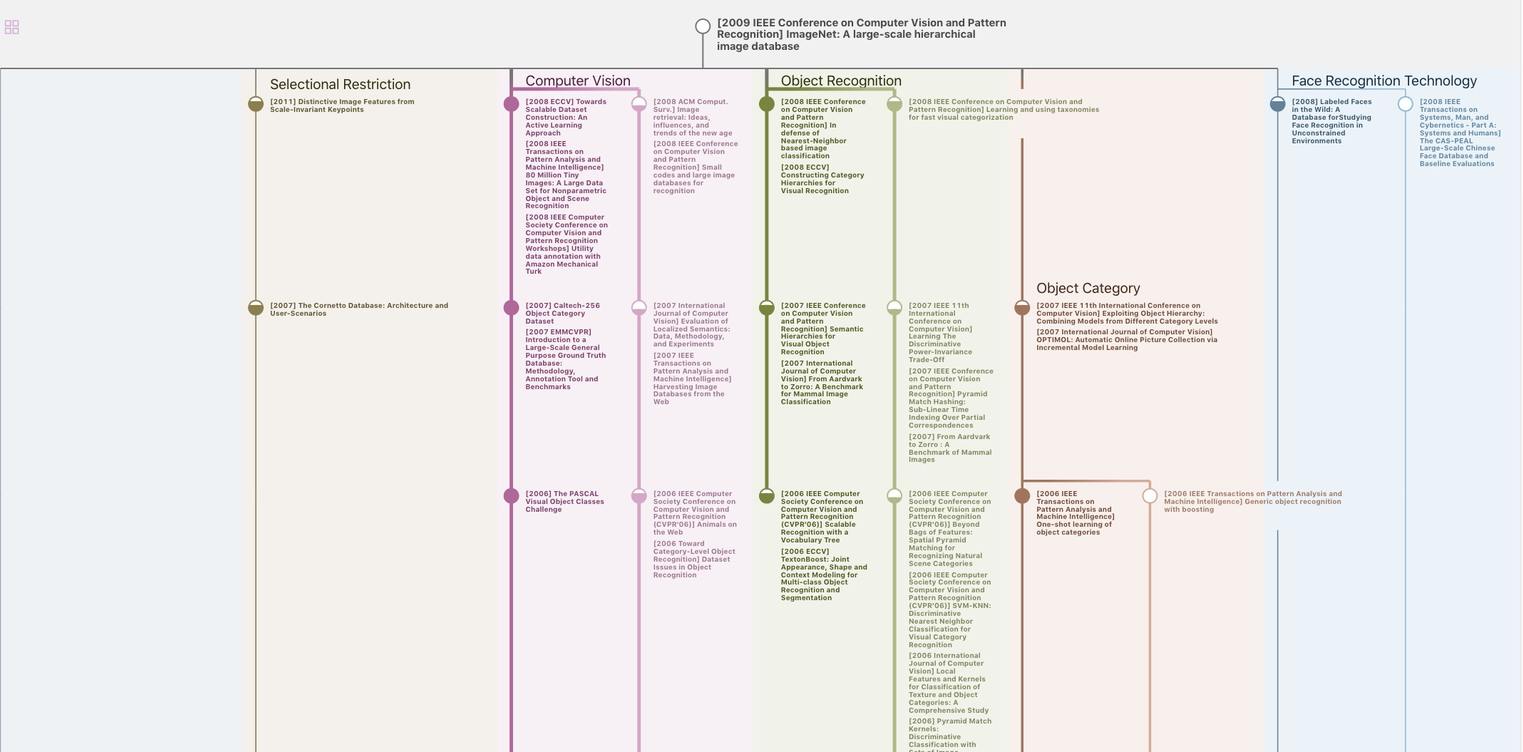
生成溯源树,研究论文发展脉络
Chat Paper
正在生成论文摘要