Neural-Net Artificial Pancreas: A Randomized Crossover Trial of a First-in-Class Automated Insulin Delivery Algorithm.
Diabetes technology & therapeutics(2024)
摘要
BACKGROUND:Automated Insulin Delivery (AID) is now integral to the clinical practice of Type 1 diabetes (T1D). The objective of this pilot-feasibility study was to introduce a new regulatory and clinical paradigm - a Neural-Net Artificial Pancreas (NAP) - an encoding of an AID algorithm into a neural network that approximates its action, and assess NAP vs the original AID algorithm.
METHODS:The UVA model-predictive control (UMPC) algorithm was encoded into a neural network, creating its NAP approximation. Seventeen AID users with T1D were recruited and 15 participated in two consecutive 20-hour hotel sessions, receiving in random order either NAP or UMPC. Their demographic characteristics were: ages 22-68 years old, duration of diabetes 7-58 years, gender 10/5 female/male, White Non-Hispanic/Black 13/2, and baseline HbA1c 5.4-8.1%.
RESULTS:The time-in-range (TIR) difference between NAP and UMPC, adjusted for entry glucose level, was 1 percentage point, with absolute TIR values of 86% (NAP) and 87% (UMPC). The two algorithms achieved similar times <70 mg/dL of 2.0% vs 1.8% and coefficients of variation of 29.3% (NAP) vs 29.1 (UMPC)%. Under identical inputs, the average absolute insulin-recommendation difference was 0.031 units/hour. There were no serious adverse events on either controller. NAP had 6-fold lower computational demands than UMPC.
CONCLUSION:In a randomized crossover study, a neural-network encoding of a complex model-predictive control algorithm demonstrated similar performance, at a fraction of the computational demands. Regulatory and clinical doors are therefore open for contemporary machine learning methods to enter the AID field.
更多查看译文
AI 理解论文
溯源树
样例
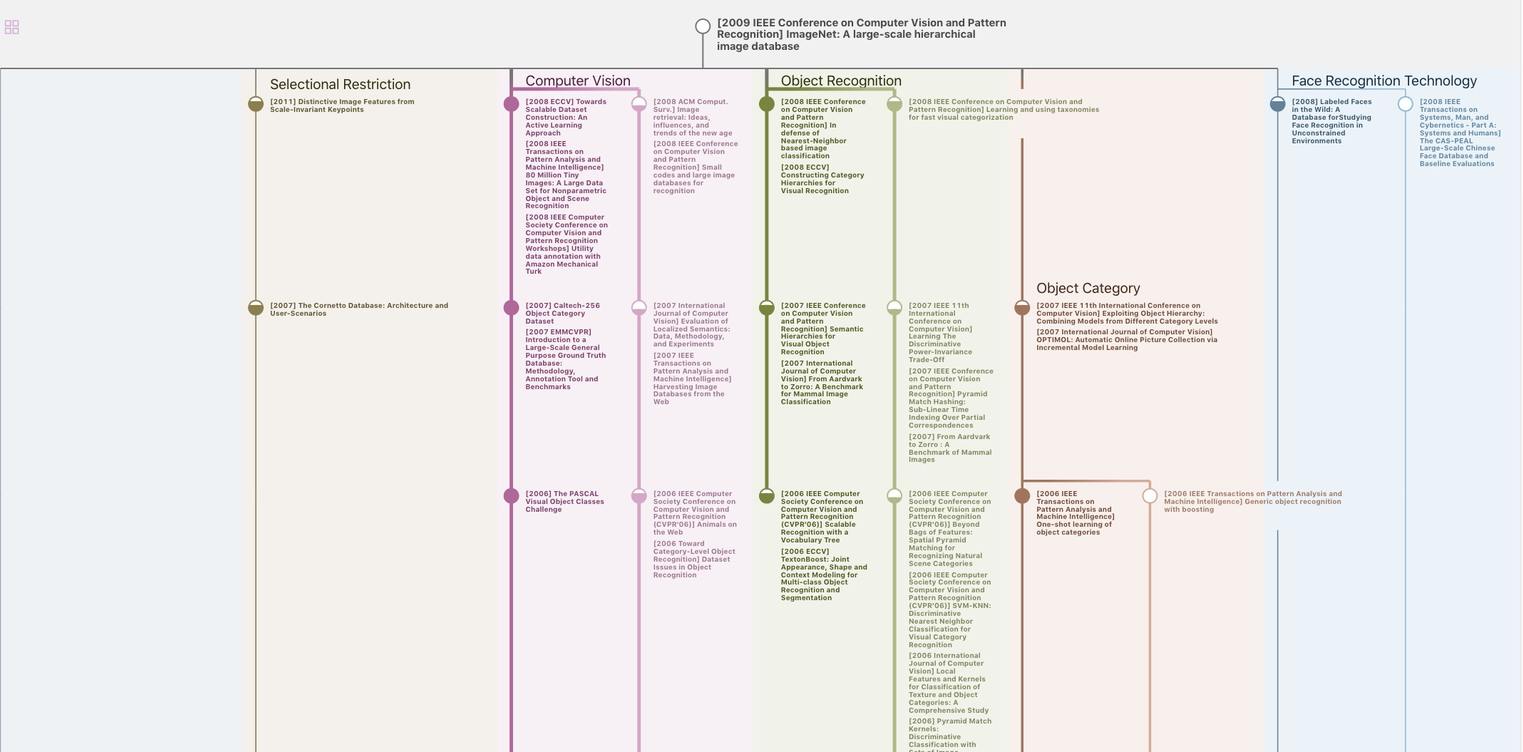
生成溯源树,研究论文发展脉络
Chat Paper
正在生成论文摘要