Data Aggregation Can Lead to Biased Inferences in Bayesian Linear Mixed Models and Bayesian Analysis of Variance
PSYCHOLOGICAL METHODS(2024)
摘要
Bayesian linear mixed-effects models (LMMs) and Bayesian analysis of variance (ANOVA) are increasingly being used in the cognitive sciences to perform null hypothesis tests, where a null hypothesis that an effect is zero is compared with an alternative hypothesis that the effect exists and is different from zero. While software tools for Bayes factor null hypothesis tests are easily accessible, how to specify the data and the model correctly is often not clear. In Bayesian approaches, many authors use data aggregation at the by-subject level and estimate Bayes factors on aggregated data. Here, we use simulation-based calibration for model inference applied to several example experimental designs to demonstrate that, as with frequentist analysis, such null hypothesis tests on aggregated data can be problematic in Bayesian analysis. Specifically, when random slope variances differ (i.e., violated sphericity assumption), Bayes factors are too conservative for contrasts where the variance is small and they are too liberal for contrasts where the variance is large. Running Bayesian ANOVA on aggregated data can-if the sphericity assumption is violated-likewise lead to biased Bayes factor results. Moreover, Bayes factors for by-subject aggregated data are biased (too liberal) when random item slope variance is present but ignored in the analysis. These problems can be circumvented or reduced by running Bayesian LMMs on nonaggregated data such as on individual trials, and by explicitly modeling the full random effects structure. Reproducible code is available from https://osf.io/mjf47/.
更多查看译文
关键词
Bayes factors,Bayesian model comparison,simulation-based calibration,sphericity assumption,items
AI 理解论文
溯源树
样例
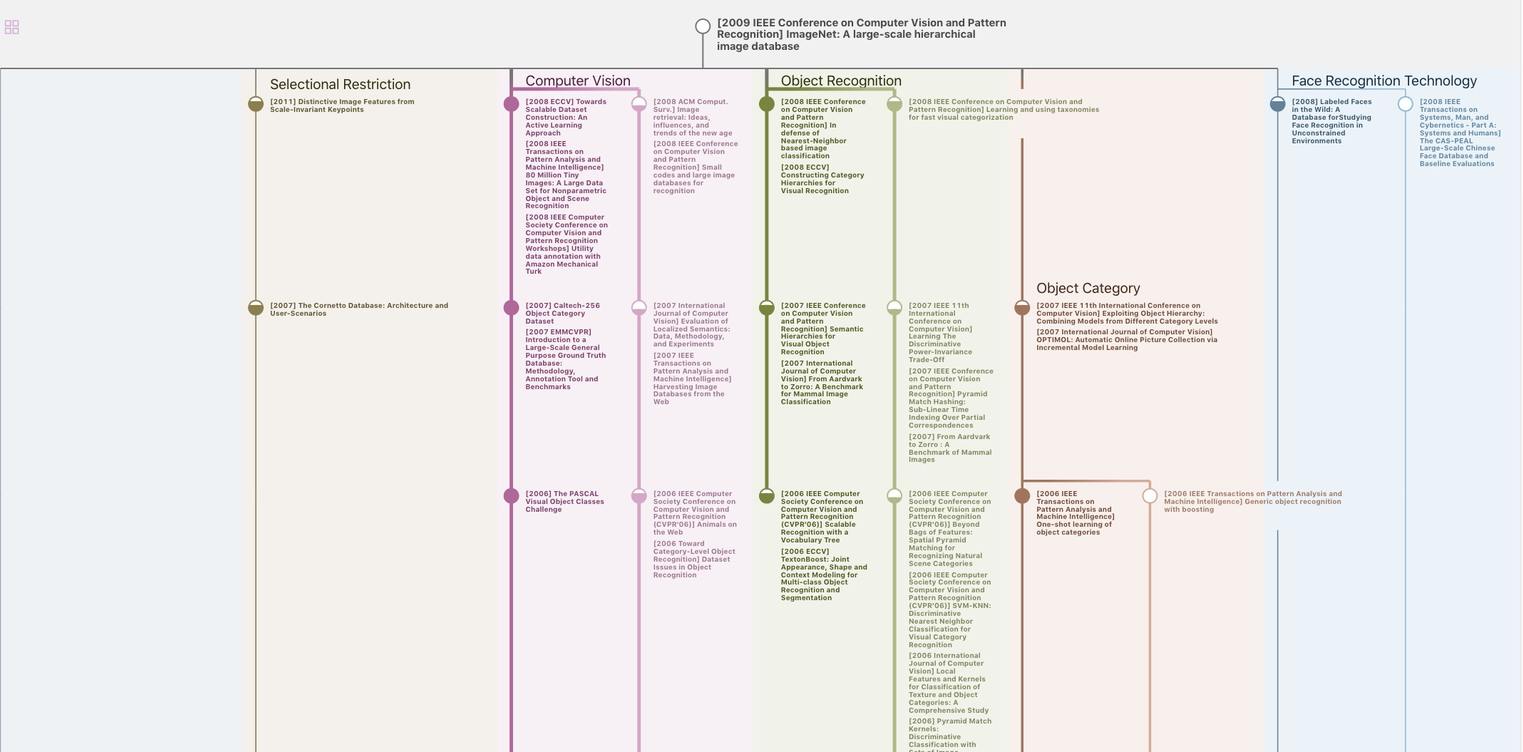
生成溯源树,研究论文发展脉络
Chat Paper
正在生成论文摘要