Functional Electrostimulation System for a Prototype of a Human Hand Prosthesis Using Electromyography Signal Classification by Machine Learning Techniques
MACHINES(2024)
摘要
Functional electrical stimulation (FES) has been proven to be a reliable rehabilitation technique that increases muscle strength, reduces spasms, and enhances neuroplasticity in the long term. However, the available electrical stimulation systems on the market produce stimulation signals with no personalized voltage-current amplitudes, which could lead to muscle fatigue or incomplete enforced therapeutic motion. This work proposes an FES system aided by machine learning strategies that could adjust the stimulating signal based on electromyography (EMG) information. The regulation of the stimulated signal according to the patient's therapeutic requirements is proposed. The EMG signals were classified using Long Short-Term Memory (LSTM) and a least-squares boosting ensemble model with an accuracy of 91.87% and 84.7%, respectively, when a set of 1200 signals from six different patients were used. The classification outcomes were used as input to a second regression machine learning algorithm that produced the adjusted electrostimulation signal required by the user according to their own electrophysiological conditions. The output of the second network served as input to a digitally processed electrostimulator that generated the necessary signal to be injected into the extremity to be treated. The results were evaluated in both simulated and robotized human hand scenarios. These evaluations demonstrated a two percent error when replicating the required movement enforced by the collected EMG information.
更多查看译文
关键词
functional electrostimulation,LSTM classifier,human hand prosthesis
AI 理解论文
溯源树
样例
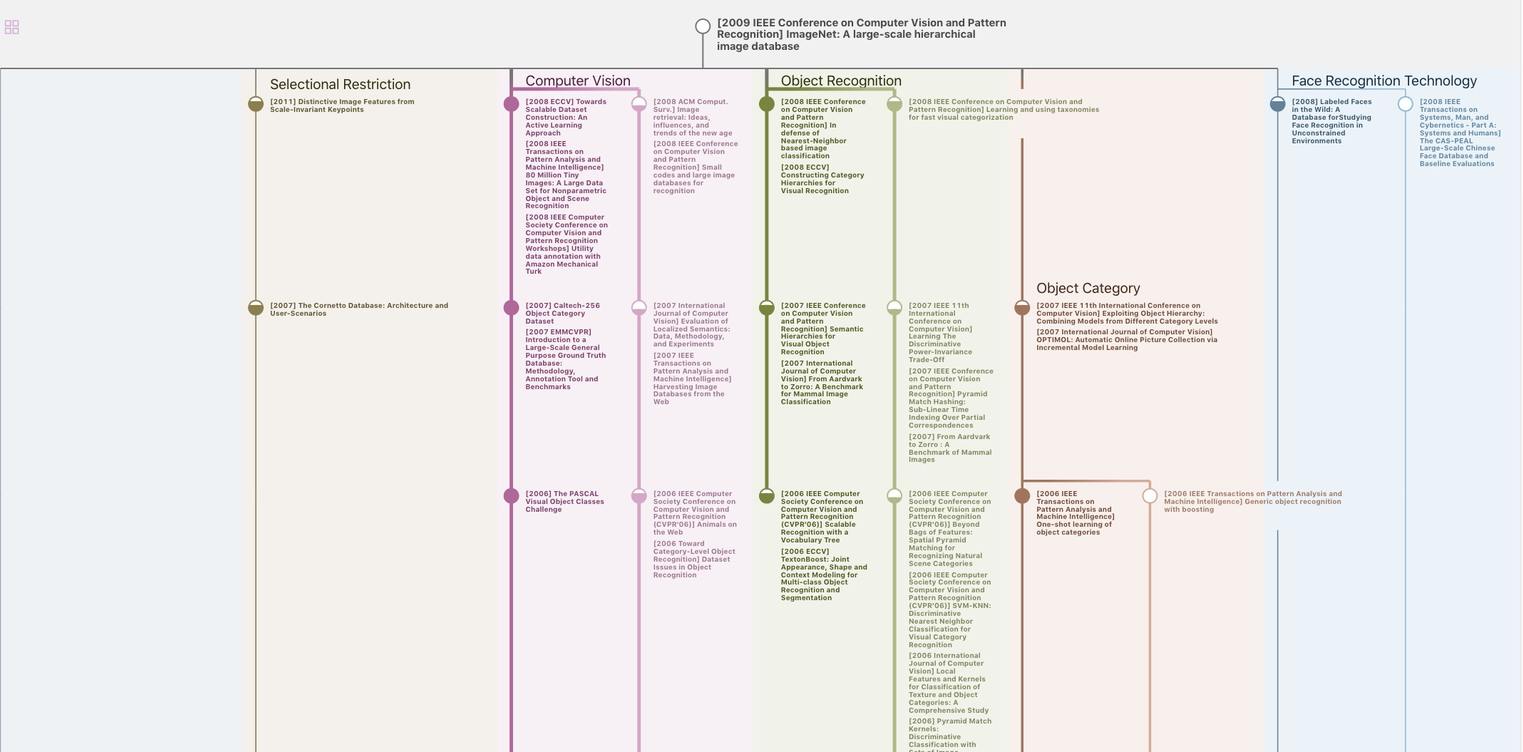
生成溯源树,研究论文发展脉络
Chat Paper
正在生成论文摘要