Automatic Detection of Phytophthora pluvialis Outbreaks in Radiata Pine Plantations Using Multi-Scene, Multi-Temporal Satellite Imagery
REMOTE SENSING(2024)
摘要
This study demonstrates a framework for using high-resolution satellite imagery to automatically map and monitor outbreaks of red needle cast (Phytophthora pluvialis) in planted pine forests. This methodology was tested on five WorldView satellite scenes collected over two sites in the Gisborne Region of New Zealand's North Island. All scenes were acquired in September: four scenes were acquired yearly (2018-2020 and 2022) for Wharerata, while one more was obtained in 2019 for Tauwhareparae. Training areas were selected for each scene using manual delineation combined with pixel-level thresholding rules based on band reflectance values and vegetation indices (selected empirically) to produce 'pure' training pixels for the different classes. A leave-one-scene-out, pixel-based random forest classification approach was then used to classify all images into (i) healthy pine forest, (ii) unhealthy pine forest or (iii) background. The overall accuracy of the models on the internal validation dataset ranged between 92.1% and 93.6%. Overall accuracies calculated for the left-out scenes ranged between 76.3% and 91.1% (mean overall accuracy of 83.8%), while user's and producer's accuracies across the three classes were 60.2-99.0% (71.4-91.8% for unhealthy pine forest) and 54.4-100% (71.9-97.2% for unhealthy pine forest), respectively. This work demonstrates the possibility of using a random forest classifier trained on a set of satellite scenes for the classification of healthy and unhealthy pine forest in new and completely independent scenes. This paves the way for a scalable and largely autonomous forest health monitoring system based on annual acquisitions of high-resolution satellite imagery at the time of peak disease expression, while greatly reducing the need for manual interpretation and delineation.
更多查看译文
关键词
random forest,WorldView,forest disease,forest decline,forest monitoring,machine learning
AI 理解论文
溯源树
样例
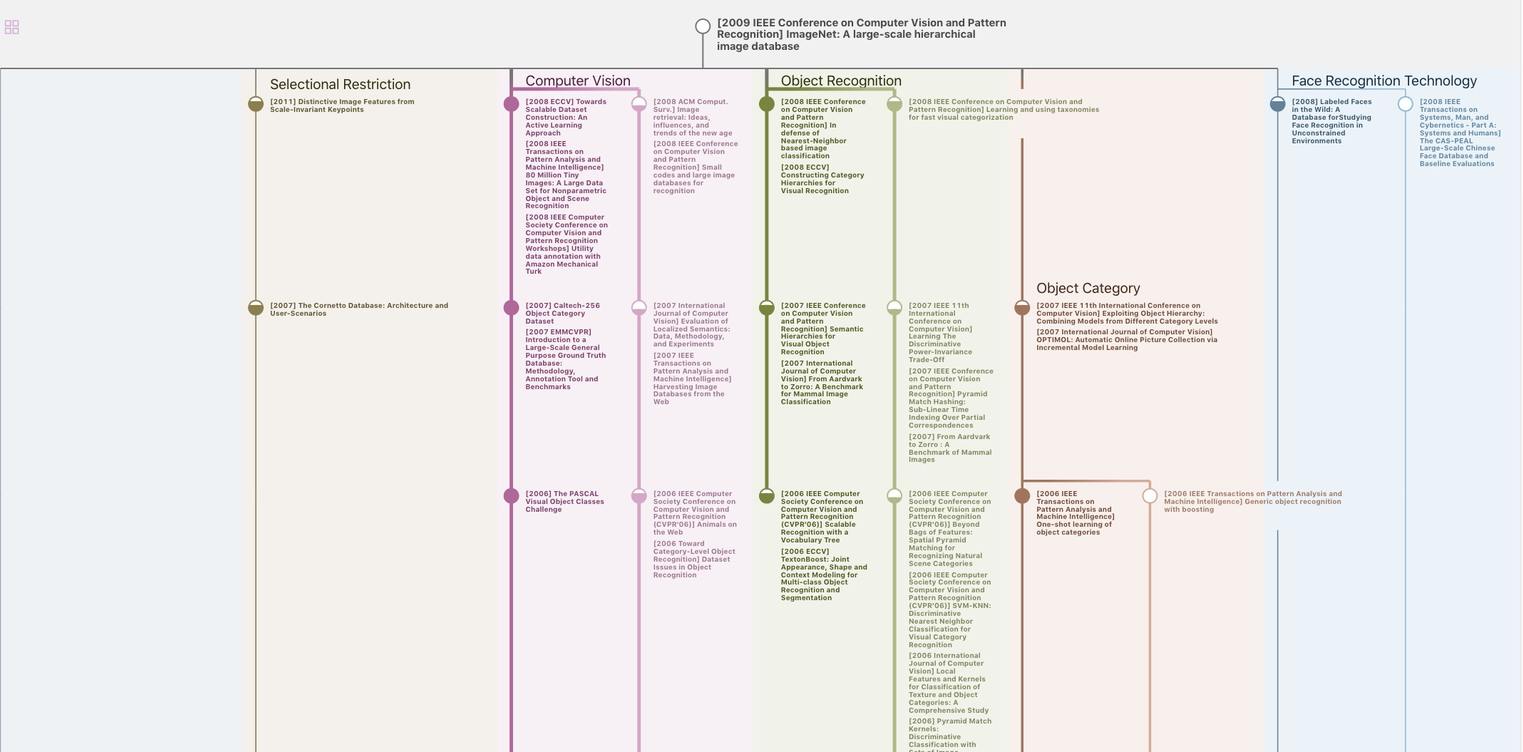
生成溯源树,研究论文发展脉络
Chat Paper
正在生成论文摘要