GanNoise: Defending against black-box membership inference attacks by countering noise generation
2023 International Conference on Data Security and Privacy Protection (DSPP)(2023)
摘要
In recent years, data privacy in deep learning has seen a notable surge of interest. Pretrained large-scale data-driven models are potential to be attacked risky with membership inference attacks. However, the current corresponding defenses to prevent the leak of data may reduce the performance of pre-trained models. In this paper, we propose a novel training framework called GanNoise that preserves privacy by maintaining the accuracy of classification tasks. Through utilizing adversarial regularization to train a noise generation model, we generate noise that adds randomness to private data during model training, effectively preventing excessive memorization of the actual training data. Our experimental results illustrate the efficacy of the framework against existing attack schemes on various datasets while outperforming advanced MIA defense solutions in terms of efficiency.
更多查看译文
关键词
data privacy,deep learning,MIA defense
AI 理解论文
溯源树
样例
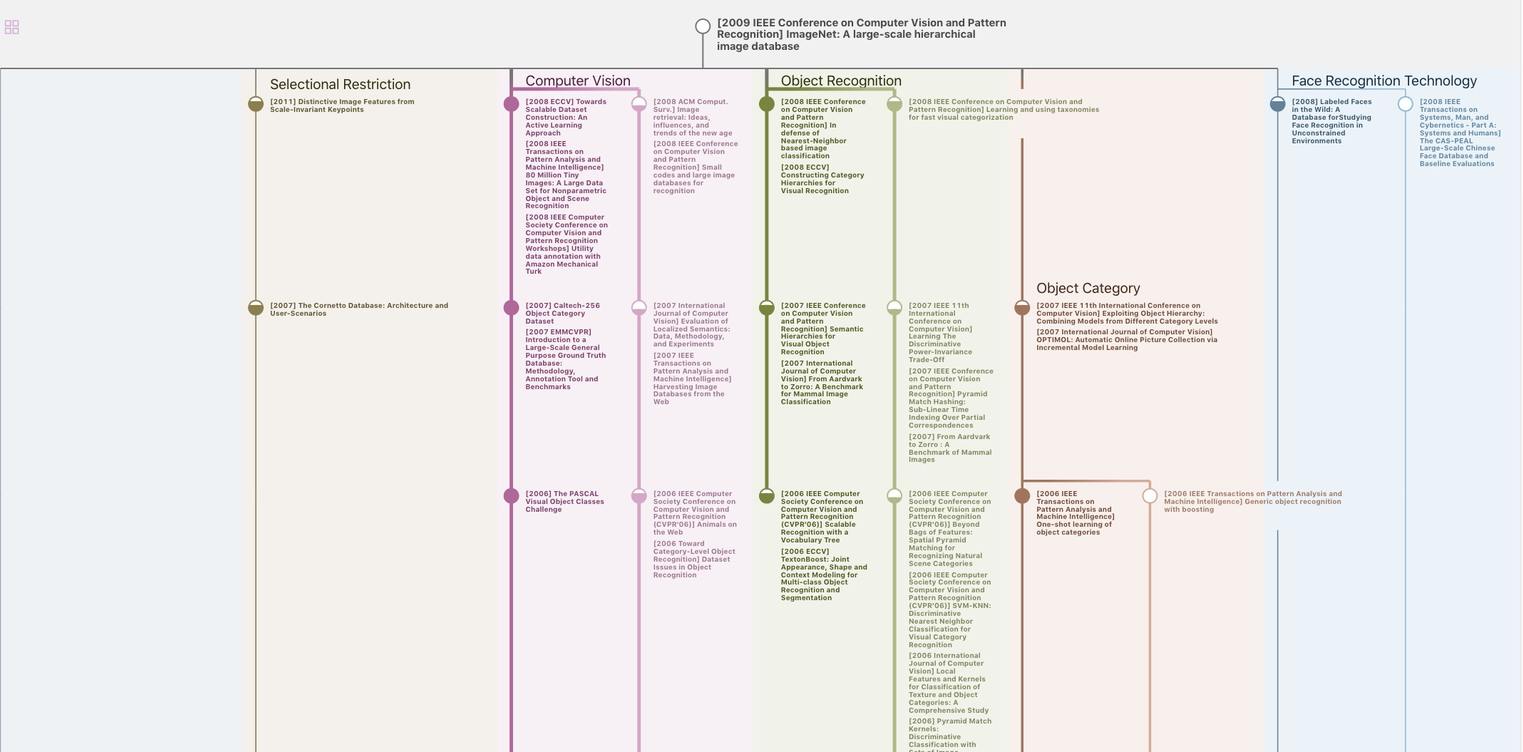
生成溯源树,研究论文发展脉络
Chat Paper
正在生成论文摘要