RS-DRL-based offloading policy and UAV trajectory design in F-MEC systems
Digital Communications and Networks(2024)
摘要
For better flexibility and greater coverage areas, Unmanned Aerial Vehicles (UAVs) have been applied in Flying Mobile Edge Computing (F-MEC) systems to offer offloading services for the User Equipment (UEs). This paper considers a disaster-affected scenario where UAVs undertake the role of MEC servers to provide computing resources for Disaster Relief Devices (DRDs). Considering the fairness of DRDs, a max-min problem is formulated to optimize the saved time by jointly designing the trajectory of the UAVs, the offloading policy and serving time under the constraint of the UAVs' energy capacity. To solve the above non-convex problem, we first model the service process as a Markov Decision Process (MDP) with the Reward Shaping (RS) technique, and then propose a Deep Reinforcement Learning (DRL) based algorithm to find the optimal solution for the MDP. Simulations show that the proposed RS-DRL algorithm is valid and effective, and has better performance than the baseline algorithms.
更多查看译文
关键词
Flying mobile edge computing,Task offloading,Reward shaping,Deep reinforcement learning
AI 理解论文
溯源树
样例
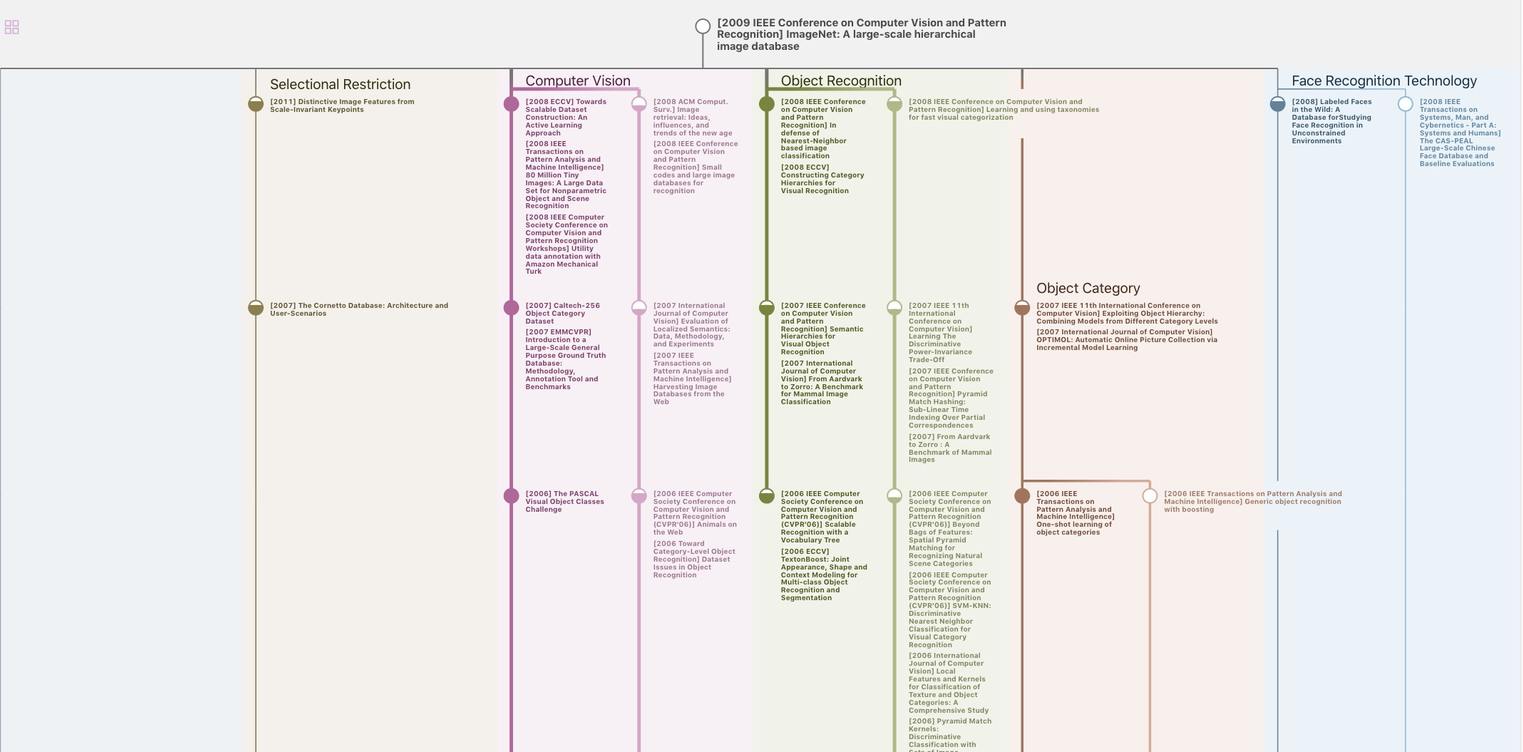
生成溯源树,研究论文发展脉络
Chat Paper
正在生成论文摘要