Wrist Motion Classification Using Flexible sEMG Sensors in Different Feature Conditions Based on Machine Learning
2023 IEEE 16th International Conference on Nano/Molecular Medicine & Engineering (NANOMED)(2023)
摘要
Electromyography (EMG) is a bioelectrical signal to reflect human intention before actual motion occurs. EMG has been widely used in human-machine interaction such as robot control, rehabilitation and health monitoring. In this work, we have designed an intelligent approach for wrist motion classification based on EMG signals. Since commercial electrodes cannot maintain good contact with skin during deformation, we have utilized a new type of fabricated flexible electrodes. With these electrodes, high-quality signals can be acquired. And the machine learning methods have been utilized to classify the extracted feature sets. Four different feature conditions have been compared. In the condition of six EMG features, we have identified four wrist gestures including wrist flexion, extension, radial deviation, and ulnar deviation with the best accuracy of 92.26%.
更多查看译文
关键词
Machine Learning,Flexible Sensors,Wrist Motion,Health Monitoring,Robot Control,Electromyography Signals,Bioelectric,Human-machine Interaction,Flexible Electrodes,Wrist Extension,High-quality Signals,Commercial Electrodes,Radial Deviation,Support Vector Machine,Linear Discriminant Analysis,Polydimethylsiloxane,Identification Of Features,Inertial Measurement Unit,Muscle Fatigue,Markov Random Field,Mean Absolute Value,Flexor Carpi Radialis,sEMG Signals,Linear Discriminant
AI 理解论文
溯源树
样例
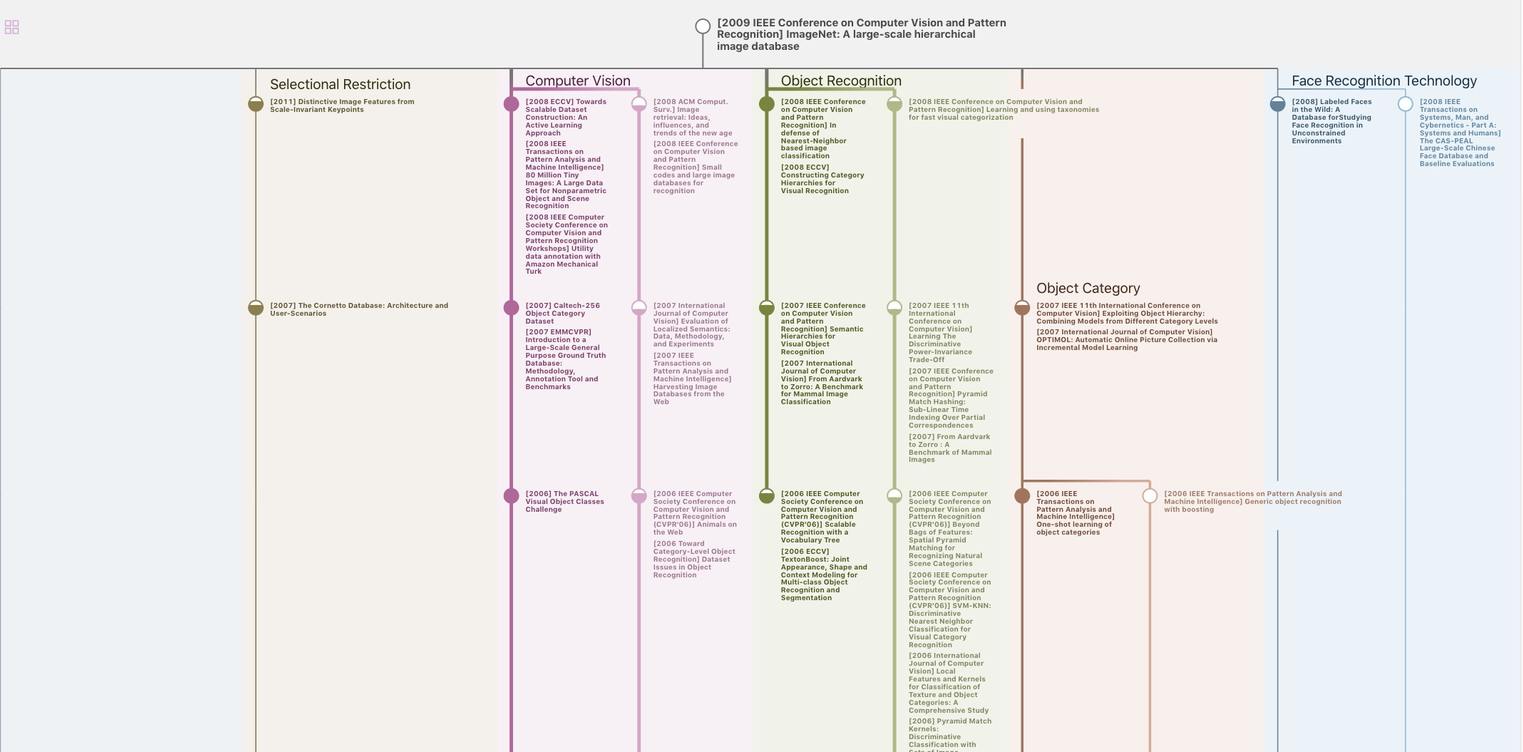
生成溯源树,研究论文发展脉络
Chat Paper
正在生成论文摘要