Exploiting Unlabeled RSSI Fingerprints in Multi-Building and Multi-Floor Indoor Localization through Deep Semi-Supervised Learning Based on Mean Teacher.
candar(2023)
摘要
Conventional indoor localization techniques, based on Wi-Fi fingerprinting under supervised learning (SL), cannot exploit unlabeled received signal strength indicators (RSSIs) measured at unknown locations. Unlabeled RSSIs could be (1) part of an initial, static fingerprint database, which are submitted by volunteers during the offline phase when the database is constructed, or (2) newly measured ones submitted by the users of an indoor localization system already deployed in the field during the online phase. In this paper, a new indoor localization framework is proposed exploiting unlabeled RSSI fingerprints in multi-building and multi-floor indoor localization through deep semi-supervised learning (SSL) based on the Mean Teacher method. The proposed framework consists of three neural network models trained in two phases, i.e., an initial model in pre-training and the student and teacher models in semi-supervised training. The pre-training phase aims to train the initial model with labeled data, for a limited number of epochs, to mitigate the cold start problem and expedite the subsequent semi-supervised training. During the semi-supervised training, the student and the teacher models, which are cloned from the pre-trained initial model, are trained with unlabeled as well as labeled data for fine tuning of their weights. To evaluate the performance of the proposed framework, experiments are conducted with the scalable indoor localization model based on a deep neural network (DNN) and the UJIIndoorLoc database, both of which are well-accepted benchmarks in multi-building and multi-floor indoor localization. Different real-world scenarios are simulated with both labeled and unlabeled data by randomly splitting the data in the UJIIndoorLoc database into labeled and unlabeled data. The results show that the proposed framework can improve the indoor localization performance of the adopted backbone network-i.e., the scalable DNN model-by up to 12.78% in terms of the minimum weighted three-dimensional localization error when only 25% of RSSI fingerprints are labeled. The localization performance of the proposed framework, in this case, is nearly equivalent to that of the scalable DNN model under the conventional framework based on SL with 100% labeled data thanks to its capability of exploiting unlabeled data through deep SSL.
更多查看译文
关键词
Multi-building and multi-floor indoor localization,Wi-Fi fingerprinting,deep semi-supervised learning,mean teacher method
AI 理解论文
溯源树
样例
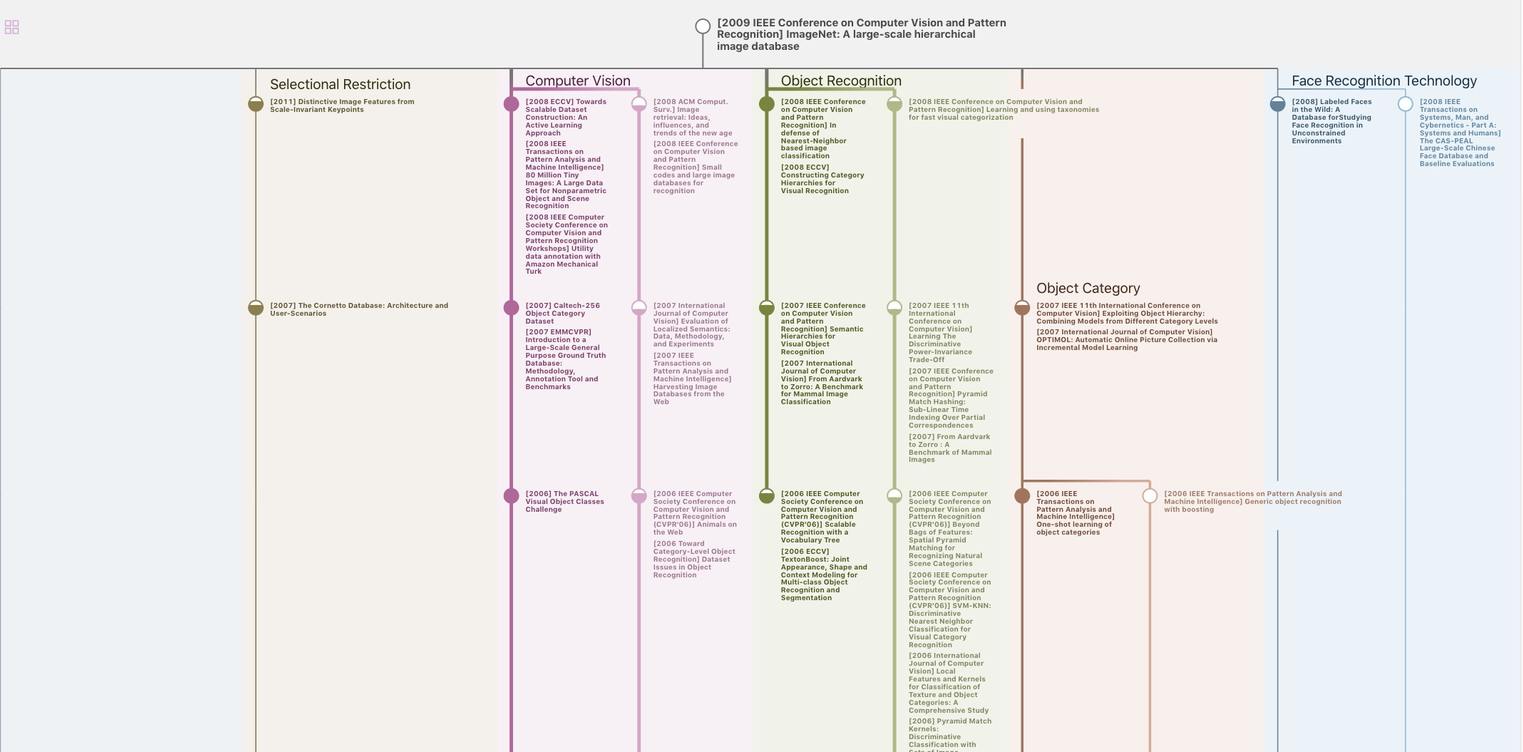
生成溯源树,研究论文发展脉络
Chat Paper
正在生成论文摘要