A blending ensemble learning model for crude oil price forecasting
Annals of Operations Research(2024)
摘要
To efficiently capture diverse fluctuation profiles in forecasting crude oil prices, we here propose to combine heterogenous predictors for forecasting the prices of crude oil. Specifically, a forecasting model is developed using blended ensemble learning that combines various machine learning methods, including k -nearest neighbor regression, regression trees, linear regression, ridge regression, and support vector regression. Data for Brent and WTI crude oil prices at various time series frequencies are used to validate the proposed blending ensemble learning approach. To show the validity of the proposed model, its performance is further benchmarked against existing individual and ensemble learning methods used for predicting crude oil price, such as lasso regression, bagging lasso regression, boosting, random forest, and support vector regression. We demonstrate that our proposed blending-based model dominates the existing forecasting models in terms of forecasting errors for both short- and medium-term horizons.
更多查看译文
关键词
Forecasting,Crude oil price,Brent,WTI,Blending,Ensemble learning,Stacking regression
AI 理解论文
溯源树
样例
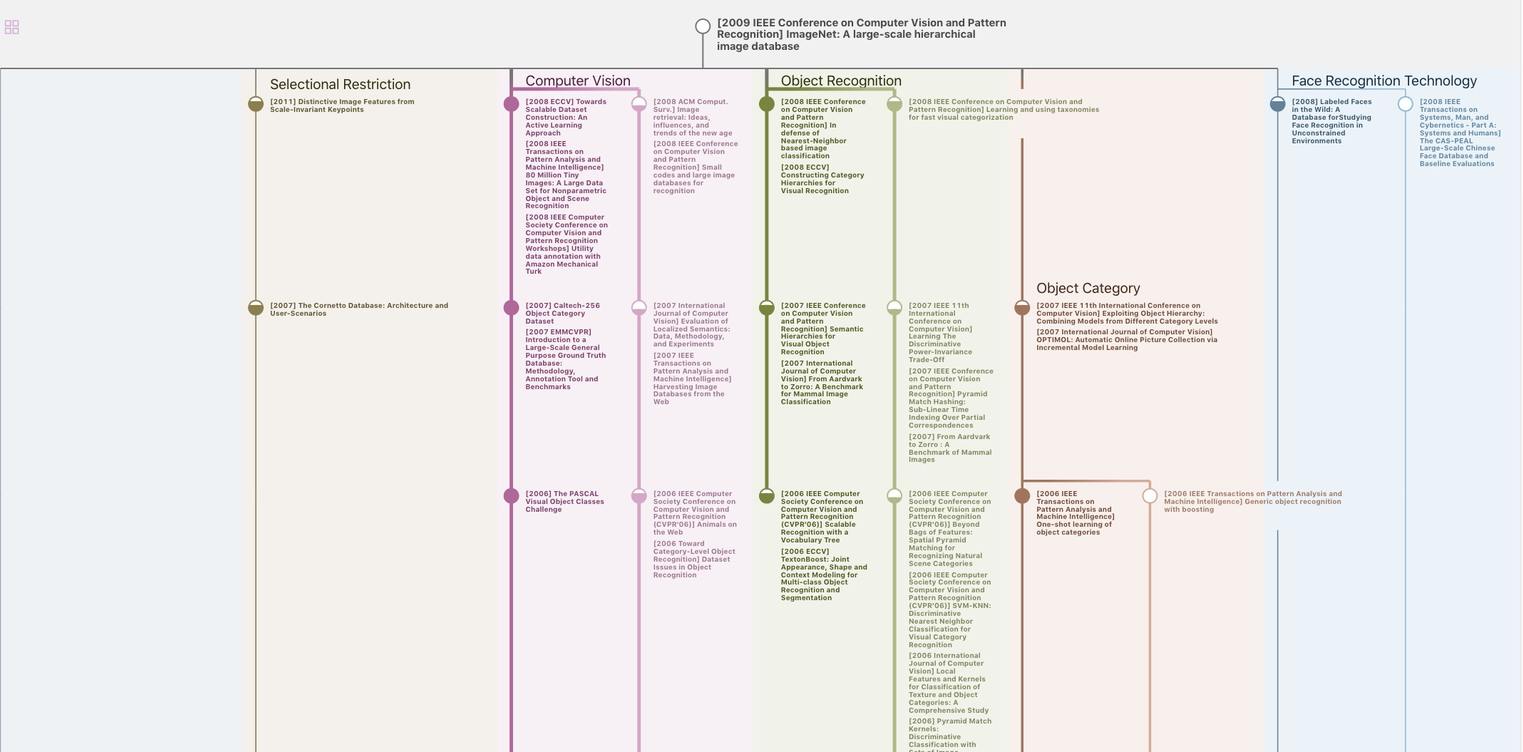
生成溯源树,研究论文发展脉络
Chat Paper
正在生成论文摘要