Contrastive Hawkes graph neural networks with dynamic sampling for event prediction
NEUROCOMPUTING(2024)
摘要
As prevalent graph-structured data evolves, representation learning for dynamic graphs has received widespread attention in recent years. Graph neural network on the dynamic graph learns dynamic features of nodes through time and structure exploration, and then performs downstream node-level (or edge-level or graph-level) task inference. Most research works model a sequence of graph snapshots for a set of discrete times (with the same interval), but such methods are difficult to handle for a dynamic graph that changes rapidly over time. In the case of a continuous-time dynamic graph, we design a Contrastive Hawkes Graph neural network with Dynamic Sampling (CHGDS) to model explicit historical importance and explore diverse neighbors. CHGDS establishes the time effect of nodes by Hawkes graph convolution, distinguishing the influence of different historical events on the current node. With dynamic sampling, our method balances time and space exploration of multi -hop neighbors. During the training phase, a contrastive learning strategy is adopted to enhance the robustness of dynamic node features. Experimental results on three continuous time dynamic graph datasets demonstrate up to 2% improvement of our model over the state -of -the -art methods.
更多查看译文
关键词
Graph neural network,Hawkes process,Contrastive learning,Dynamic sampling
AI 理解论文
溯源树
样例
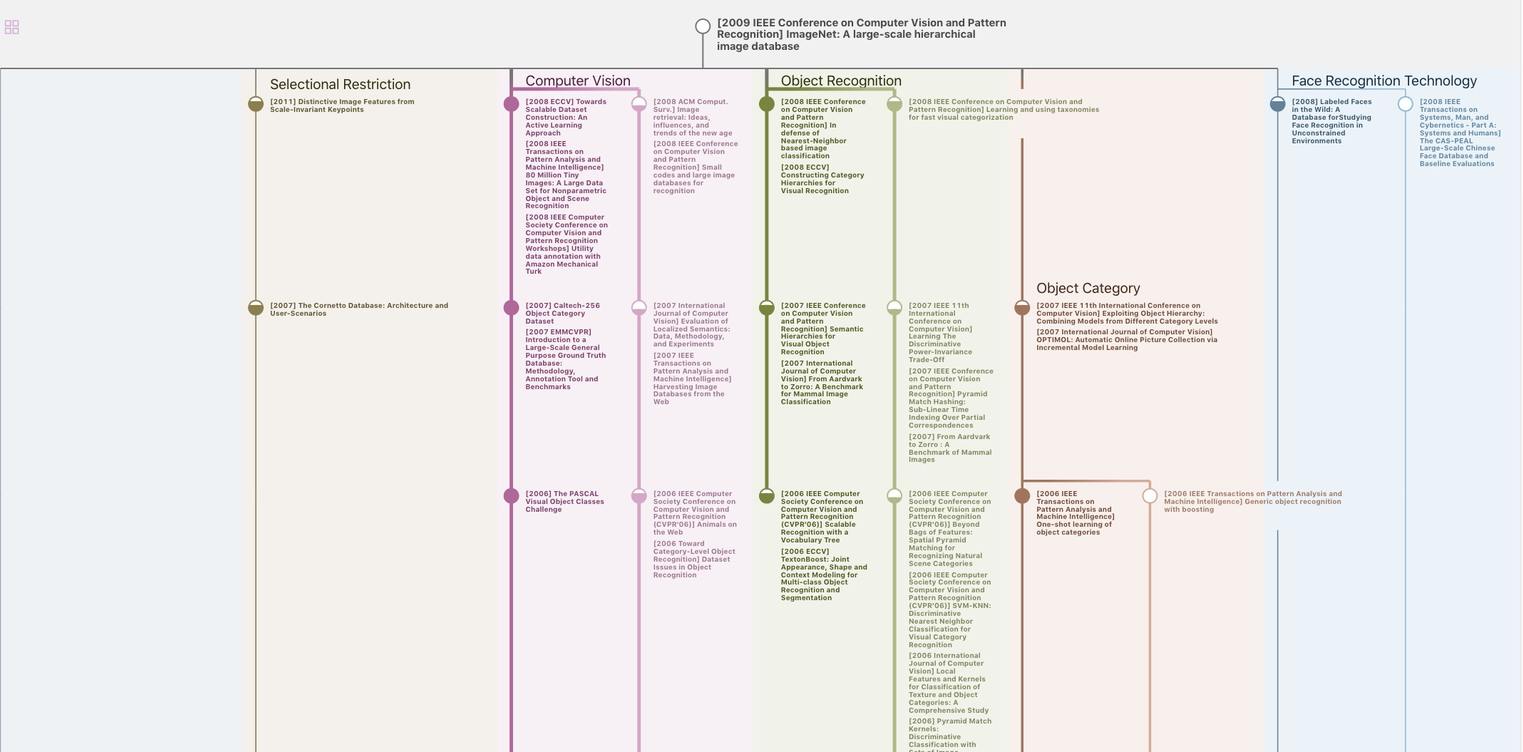
生成溯源树,研究论文发展脉络
Chat Paper
正在生成论文摘要