A robust consensus + innovations-based distributed parameter estimator
arxiv(2024)
摘要
While distributed parameter estimation has been extensively studied in the
literature, little has been achieved in terms of robust analysis and tuning
methods in the presence of disturbances. However, disturbances such as
measurement noise and model mismatches occur in any real-world setting.
Therefore, providing tuning methods with specific robustness guarantees would
greatly benefit the practical application. To address these issues, we recast
the error dynamics of a continuous-time version of the widely used consensus +
innovations-based distributed parameter estimator to reflect the error dynamics
induced by the classical gradient descent algorithm. This paves the way for the
construction of a strong Lyapunov function. Based on this result, we derive
linear matrix inequality-based tools for tuning the algorithm gains such that a
guaranteed upper bound on the L2-gain with respect to parameter variations,
measurement noise, and disturbances in the communication channels is achieved.
An application example illustrates the efficiency of the method.
更多查看译文
AI 理解论文
溯源树
样例
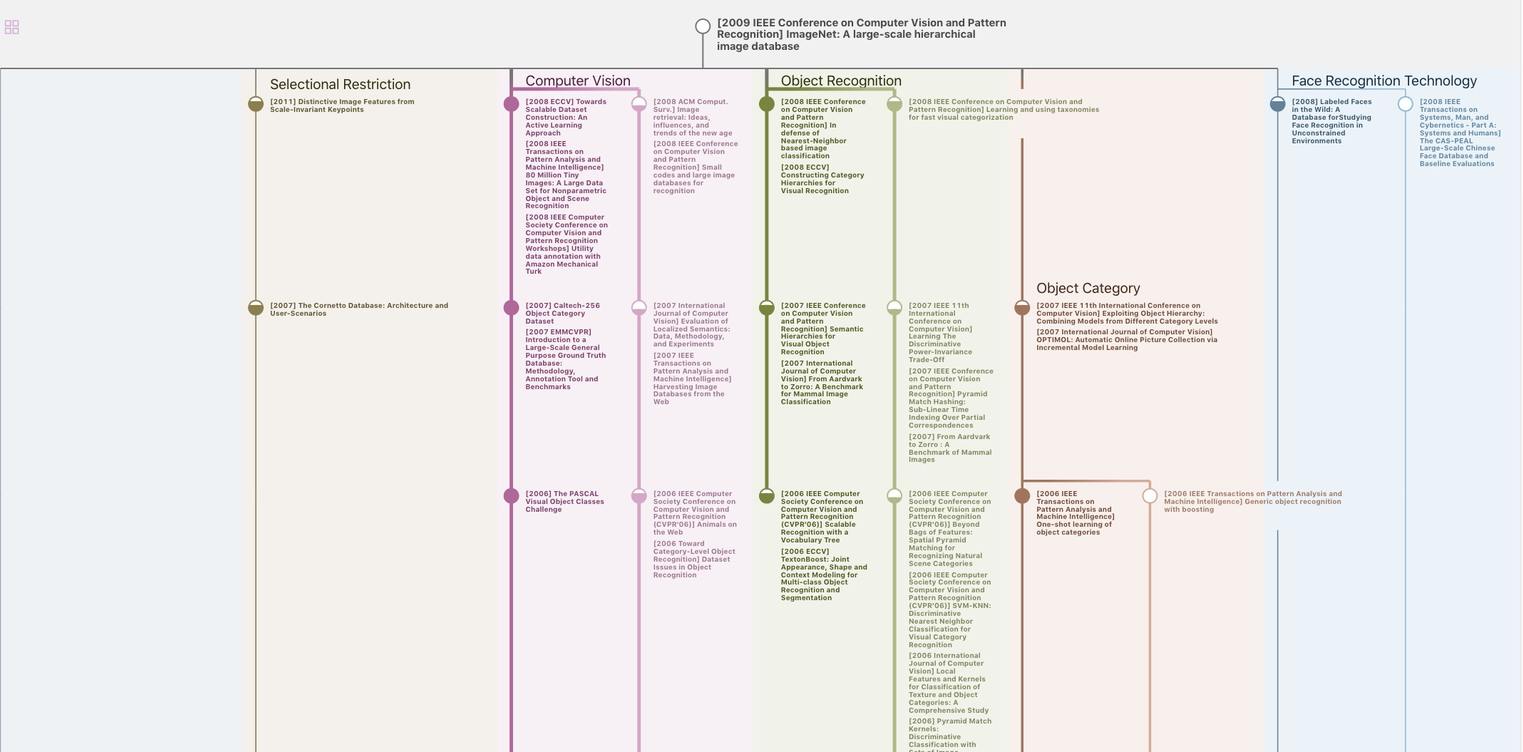
生成溯源树,研究论文发展脉络
Chat Paper
正在生成论文摘要