A robust distribution network state estimation method based on enhanced clustering Algorithm: Accounting for multiple DG output modes and data loss
INTERNATIONAL JOURNAL OF ELECTRICAL POWER & ENERGY SYSTEMS(2024)
摘要
This paper proposes a new forecasting-aided state estimation (FASE) method for distribution systems that mitigates issues with uncertain distributed generation (DG) and lost measurements. We utilize an Improved Particle Swarm Optimization (IPSO)-optimized Density-Based Spatial Clustering of Applications with Noise (DBSCAN) for examination of historical DG output data. Based on identified DG output modes, it facilitates precise state prediction and data reconstruction,. The proposed method employs a Bidirectional Gated Recurrent Unit (BiGRU) neural network for state prediction and a particle filter (PF) for final state filtering. The method verification is provided through Python simulations of the Distribution Transformer Unit (DTU)7k distribution network system, demonstrating improved accuracy and robustness against sudden load change and bad data in measurements.
更多查看译文
关键词
with noise,Forecasting -aided state estimation,Improved particle swarm optimization,Machine learning,Neural network,State estimation
AI 理解论文
溯源树
样例
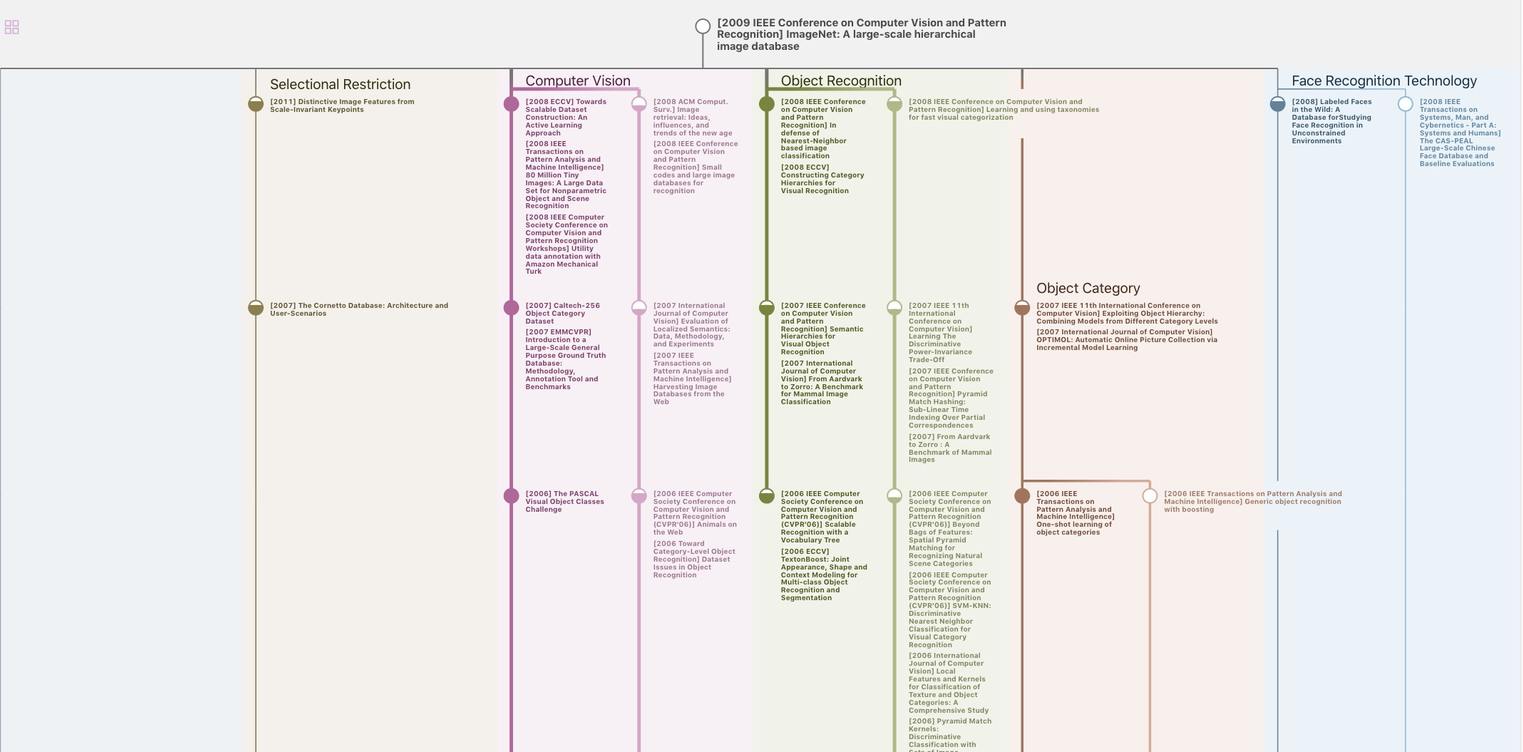
生成溯源树,研究论文发展脉络
Chat Paper
正在生成论文摘要