An LSTM-stacked autoencoder multisource response prediction and constraint optimization for scaled expansion tubes
APPLIED SOFT COMPUTING(2024)
摘要
Deep learning is attracting increasing attention due to its excellent predictive power and its ability to be applied in traditional research areas. In this paper, we propose a multisource response prediction network architecture based on a long short-term memory (LSTM)-stacked autoencoder to predict key crashworthiness indicators and complete curve reconstruction. Taking an expansion tube as an example using an equivalent scaling research method, a scaled expandable tubular (SET) finite element model was established and verified using a quasistatic compression test and a full-size coupler and buffer system experiment. A design of experiments (DOE) approach was used to obtain a dataset for training the prediction network. Neural network hyperparameters are critical to network prediction accuracy, and after comparison, the multisource response prediction network architecture showed good computational efficiency and satisfactory prediction accuracy when appropriate hyperparameters were selected. Subsequently, multiobjective constraint optimization was performed using the nondominated sorting genetic algorithm -II (NSGA-II) based on a prediction network architecture, which greatly improved the energy -absorption structure optimization accuracy. The results are expected to provide a research methodology for solving complex engineering problems by establishing a new framework for deep learning algorithms combined with optimization methods.
更多查看译文
关键词
LSTM-stacked autoencoder,Deep learning,Multisource response prediction,Constrained optimization,Scaled expandable tubular
AI 理解论文
溯源树
样例
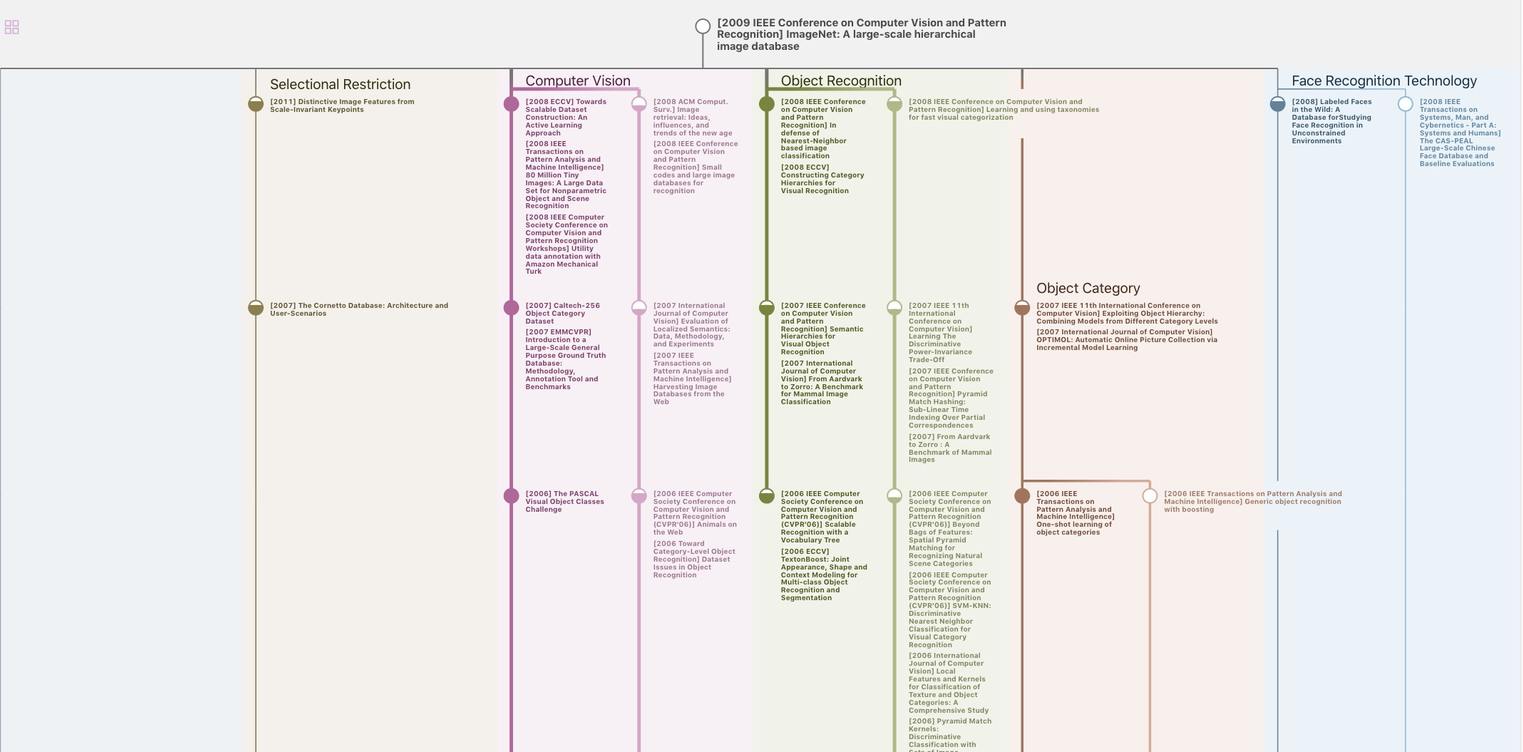
生成溯源树,研究论文发展脉络
Chat Paper
正在生成论文摘要