An Intelligent Co-Scheduling Framework for Efficient Super-Resolution On Edge Platforms With Heterogeneous Processors
IEEE Internet of Things Journal(2024)
摘要
Deep neural networks (DNNs) have shown remarkable performance in the super-resolution (SR) task, which can upscale low-resolution images to satisfy application demands on image quality. However, the high computational intensity of DNN models poses a challenge to executing SR tasks on resource-constrained edge platforms. To leverage heterogeneous computational resources (e.g., CPU, GPU, and NPU) to speed up image reconstruction through concurrent inference, we propose a novel framework, called ESHP, for Efficient Super-resolution on edge platforms with Heterogeneous Processors. Our proposed ESHP framework boasts several advantageous characteristics: 1) it substantially speeds up SR processing over the existing approaches by leveraging all available heterogeneous hardware; 2) it uses deep reinforcement learning (DRL) to enable adaptive and optimal scheduling based on runtime states; 3) it strikes a balance between SR performance and computational cost during inference; and 4) it does not modify the original architecture of given SR model. We have conducted extensive experiments on typical edge platforms with popular SR models and resolution datasets of different scales, which verify the effectiveness and the versatility of our ESHP against other commonly-used baselines.
更多查看译文
关键词
Super-resolution,Convolutional neural networks,Edge computing,Heterogeneous hardware,Deep reinforcement learning
AI 理解论文
溯源树
样例
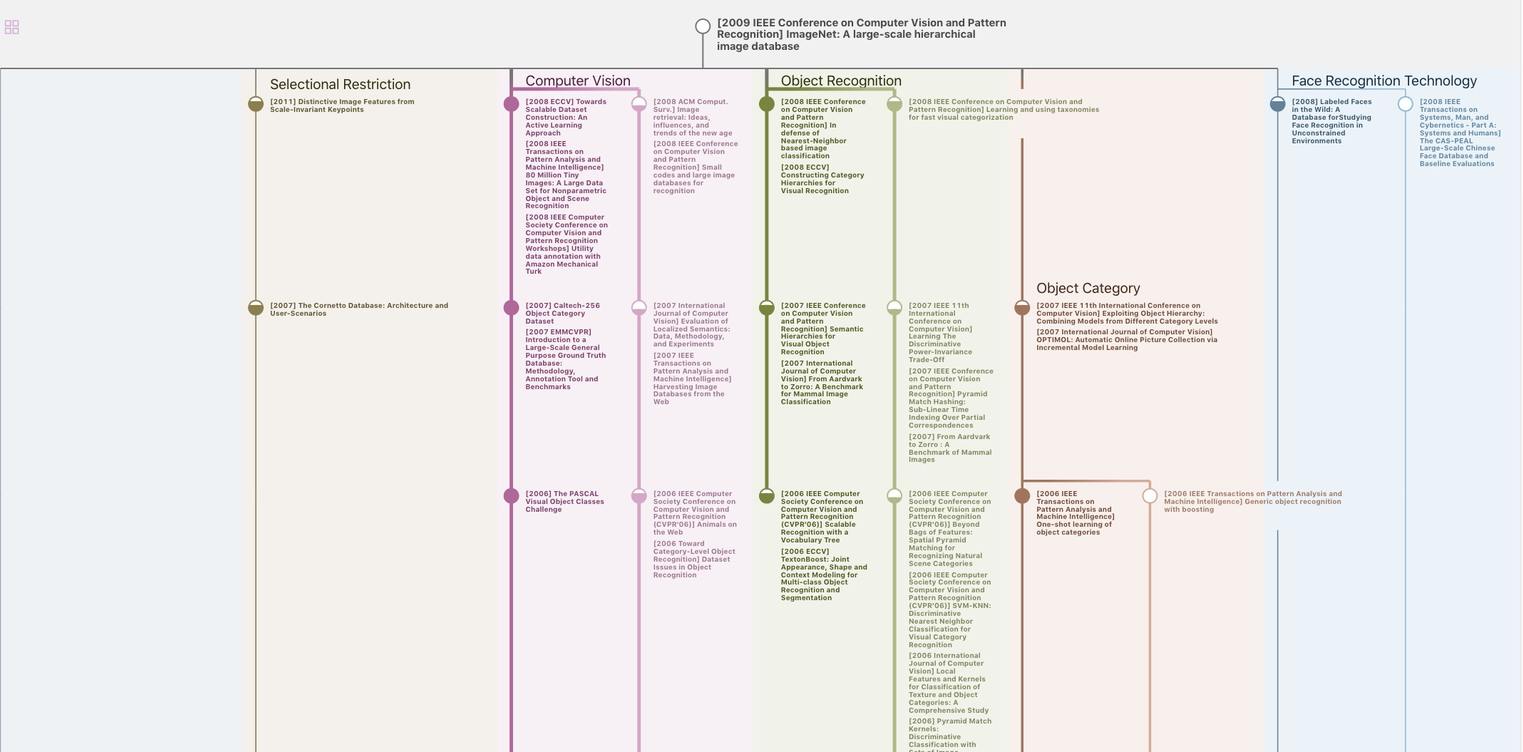
生成溯源树,研究论文发展脉络
Chat Paper
正在生成论文摘要