Dynamic Voltage Regulation in Active Distribution Networks Using Day-Ahead Multi-Agent Deep Reinforcement Learning
IEEE TRANSACTIONS ON POWER DELIVERY(2024)
摘要
The voltage deviation in an active distribution network (ADN) can effectively be managed by using smart inverters' reactive power control. A cutting-edge multi-agent deep reinforcement learning (MADRL) techniques allow smart inverters to achieve localized and coordinated voltage control by learning to take optimal actions. However, existing researches have significantly overlooked reactive power planning before training the agents, leading to inefficient use of resources and a potential increase in power losses. Further, the pre-determined network topology is the major assumption in recent works, causing inappropriate reactive power injection/absorption by the inverters. Therefore, we proposed a day-ahead multi-argent deep reinforcement learning (DA-MADRL) for dynamic voltage regulation to address the issues with earlier works. Firstly, a model-free reactive power prediction scheme is proposed, followed by determining the optimal number and the location of participants. Secondly, the MADRL is followed to train optimally located inverters for voltage regulation. The learned parameters are broadcasted on the network on a day-ahead basis. We deployed a modified IEEE-33 bus system as a test bench to verify the efficacy of our method. The results demonstrate that our method outperforms contemporary techniques in reducing voltage variation and power losses by efficient resource utilization in a distribution network.
更多查看译文
关键词
Voltage control,Reactive power,Inverters,Predictive models,Distribution networks,Training,Minimization,Voltage regulation,smart inverters,deep reinforcement learning,reactive power prediction
AI 理解论文
溯源树
样例
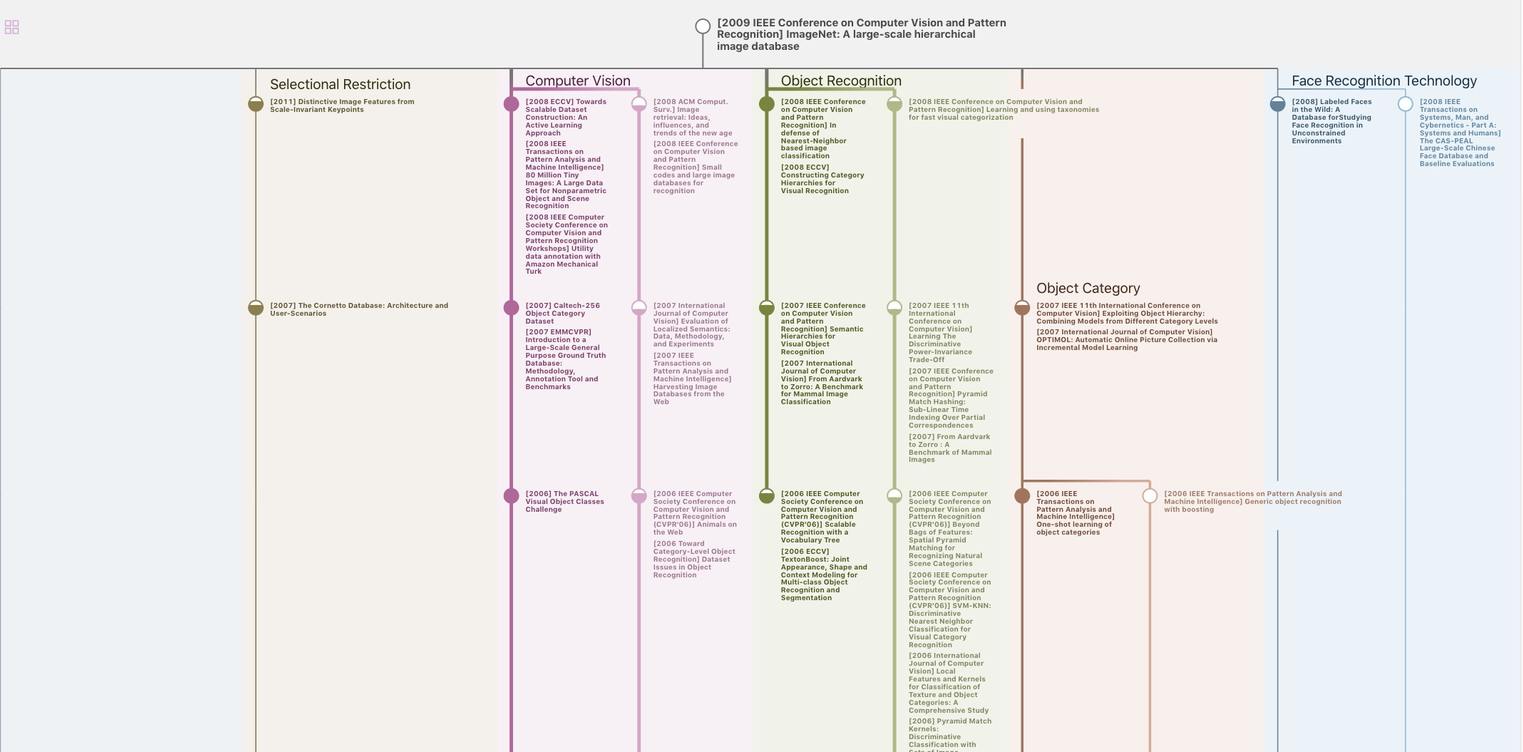
生成溯源树,研究论文发展脉络
Chat Paper
正在生成论文摘要