Improved Motor Imagery EEG Inter-device Decoding by Reweighting Multi-source Domain Samples
IEEE Transactions on Instrumentation and Measurement(2024)
摘要
Objective: Electroencephalogram (EEG) based motor imagery brain–computer interface (MI BCI) has exciting prospects in applications. Multi-source domain problem of MI EEG decoding needs to be solved urgently. That is, how to use existing vast amounts of MI EEG data (multi-source domain) to train inter-device algorithms for new equipment (target domain) decoding. Methods: In this work, we propose a compact SRENet method and a sample reweighting training strategy to solve this issue. The target domain is expressed as a weighted combination of multi-source domains to improve the decoding performance of inter-device MI. A novel sample reweighting classifier and a conditional reweighting discriminator are used for reweighting multi-source domain samples in training process. Results: We evaluated the performance of SRENet on three public datasets. The results outperformed baseline method by 6.88%, 5.90% and 3.49% on the three tasks, respectively. Conclusion: Experimental results verified the effectiveness of the proposed method for multi-source domain problems. The inter-device MI performance has been significantly improved. Significance: This study provides a new solution for multi-source domain problem in MI EEG decoding, which will make better use of existing EEG datasets and help people use BCI more easily.
更多查看译文
关键词
electroencephalogram,brain-computer interface,motor imagery,inter-device decoding
AI 理解论文
溯源树
样例
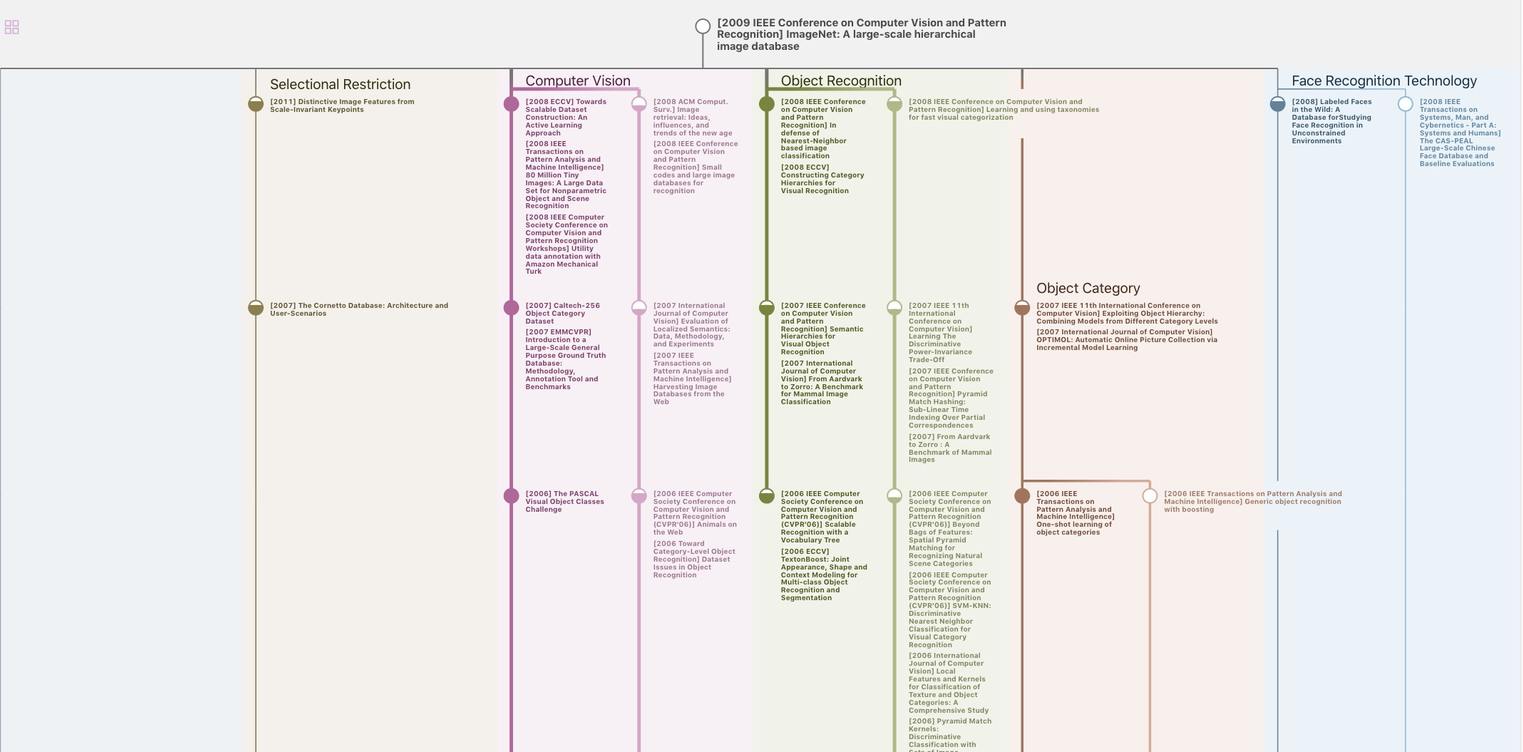
生成溯源树,研究论文发展脉络
Chat Paper
正在生成论文摘要