Is one label all you need? Single positive multi-label training in medical image analysis.
2023 IEEE International Conference on Big Data (BigData)(2023)
摘要
Deep Learning is proving its immense potential in medical image processing. However, noisy label data can weaken the generalization ability of the model and cause significant performance degradation. This issue can be more prevalent in multi-label classification tasks, since their annotation is more challenging. With too many labels, human annotators may have difficulties mentioning all possible classes, which leads to an increased number of false negative labels. Single Positive Multi-Label (SPML) training deals with the most severe version of this problem, in which for each sample only one positive label is available. All other labels are not observed, i.e. not confirmed as positive or negative. While SPML has already achieved good results for the multi-label detection of objects, its impact on the extremely pertinent medical imaging use case has not yet been explored. In this work, we therefore investigate the performance of state-of-the-art SPML loss functions in the analysis of chest X-rays, both on the public CheXpert and on an in-house data set of the University Hospital Bonn. In addition, we propose our new SPML loss functions, the Generalized Assume Negative and Implicit Weighting Assume Negative loss, which increase the mean average precision by up to 4.6% compared to the popular binary cross-entropy loss.
更多查看译文
关键词
Single Positive Multi-Label,Chest X-Rays,Deep Learning
AI 理解论文
溯源树
样例
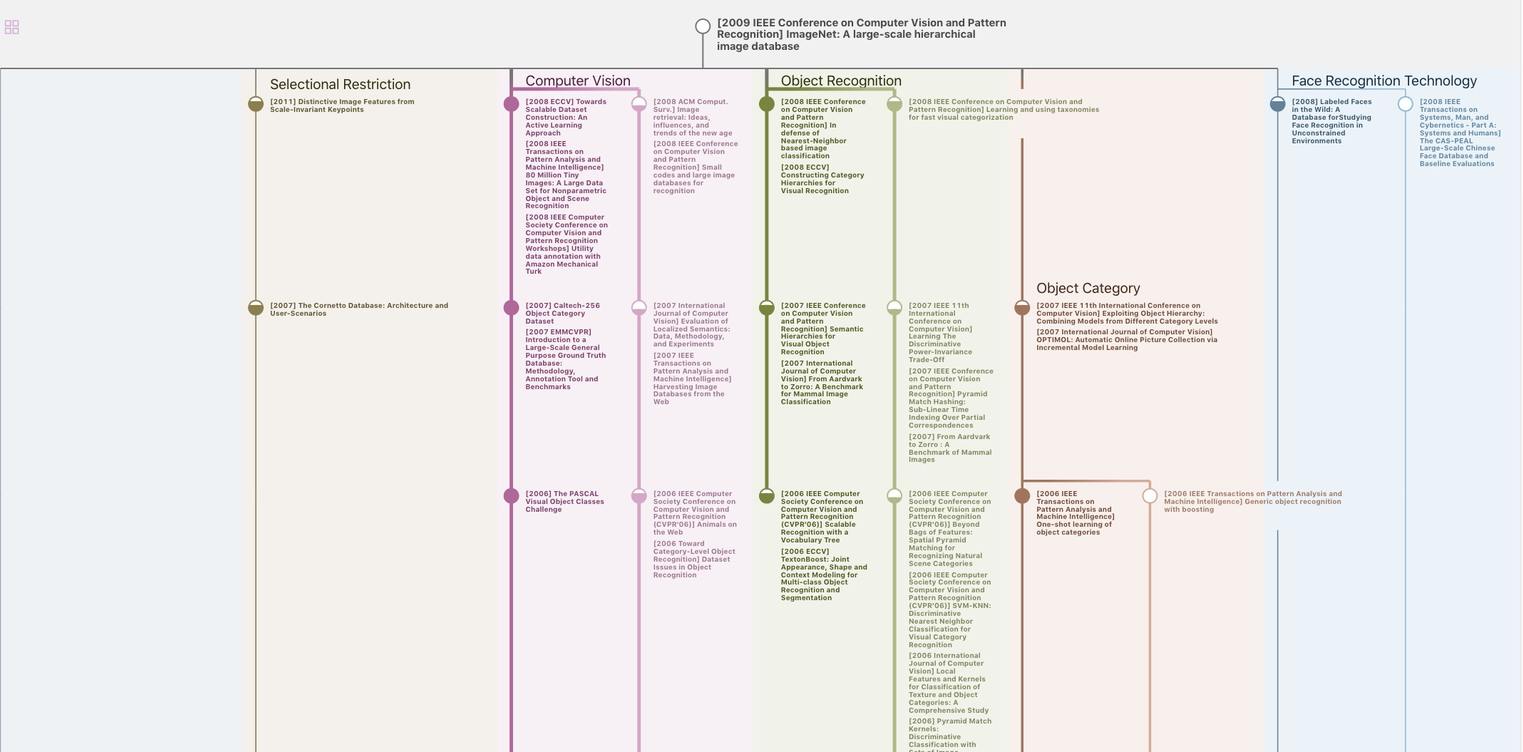
生成溯源树,研究论文发展脉络
Chat Paper
正在生成论文摘要