Learning the Dynamics of Concentration Fields in Vascular Stenosis with Deep Hidden Physics Models.
2023 IEEE International Conference on Big Data (BigData)(2023)
摘要
Understanding the dynamics of blood flow is crucial in the context of cardiovascular health and disease. The dynamics of the blood flow can be a significant parameter for the development of decision support systems to enable early detection and accurate diagnosis of coronary artery diseases. Uncovering the underlying dynamics from high-dimensional data generated from experiments is a highly complex problem at the intersection of artificial intelligence and applied mathematics. Deep Hidden Physics Models can be used to learn the underlying dynamics without additional physical knowledge.In this work, the potential of Deep Hidden Physics Models to model the clinically relevant dynamics of blood flow is investigated. The experiments consider the use case of stenosis in two-dimensional spatial space. Based on the learned dynamics, the concentration field can be approximated accurately, indicating that the dynamics are learned correctly. Additionally, we examine the capability of the model to extrapolate the learned dynamics for unknown time intervals.
更多查看译文
关键词
Machine Learning,Deep Hidden Physics Models,Blood Flow Simulations,Stenosis
AI 理解论文
溯源树
样例
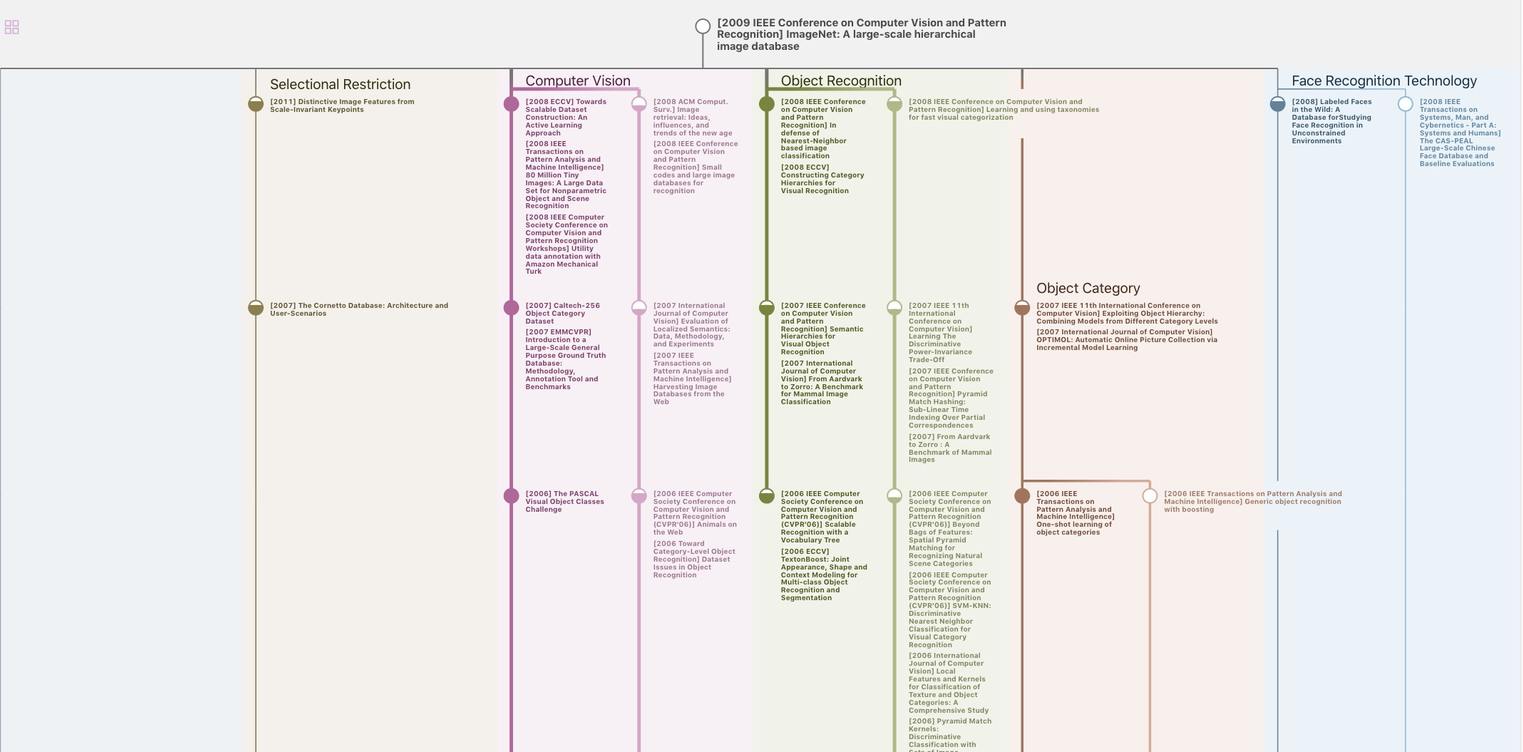
生成溯源树,研究论文发展脉络
Chat Paper
正在生成论文摘要