Modular Deep Learning for Big Data: Motivation, Challenges, and Approaches.
2023 IEEE International Conference on Big Data (BigData)(2023)
摘要
Deep learning (DL) technologies have the potential to fundamentally transform many Big Data domains ranging from agriculture and healthcare to transportation and entertainment. However, a large percentage of “non-tech” sectors have been unable to fully harness the power of DL technologies because of the lack of expertise, resources and training data for developing, tuning and managing DL models. The monolithic model paradigm that dominates the current DL landscape has a number of drawbacks that have impeded wider adoption of DL in various Big Data domains. This paper presents an alternate vision, namely modular DL paradigm. Semantically meaningful DL modules form the basic building blocks of this paradigm, and specific DL tasks are accomplished by creating pipelines of these modules. This paper motivates the need for modular DL through a case study that includes an experimental analysis examine the trade-offs between modular and monolithic DL paradigms. We discuss the challenges in designing a modular DL framework for robust and cost-effective Big Data analytics, and outline approaches to address the challenges.
更多查看译文
关键词
deep learning,machine learning,modularity,deep learning solution engineering,machine learning systems
AI 理解论文
溯源树
样例
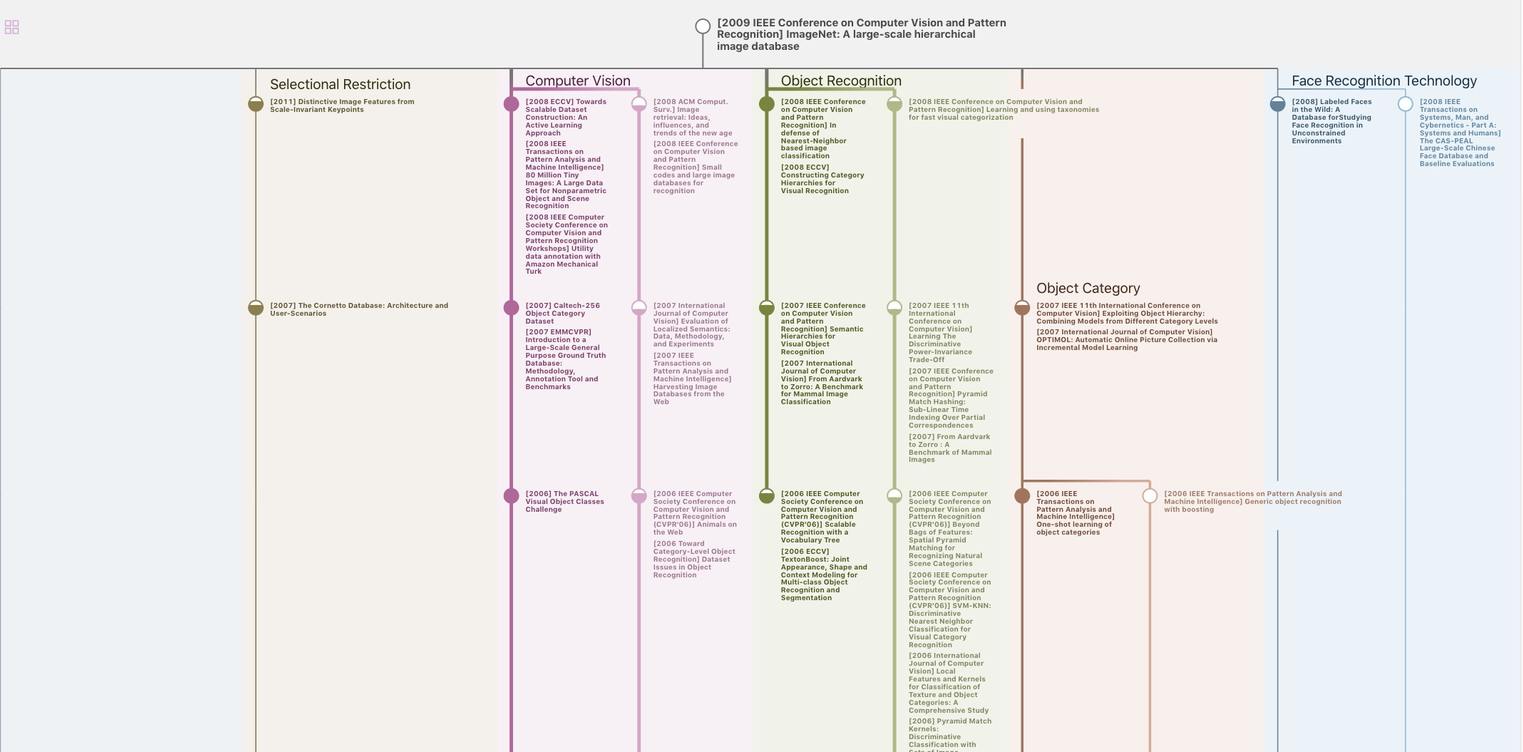
生成溯源树,研究论文发展脉络
Chat Paper
正在生成论文摘要