FedCIO: Efficient Exact Federated Unlearning with Clustering, Isolation, and One-shot Aggregation.
2023 IEEE International Conference on Big Data (BigData)(2023)
摘要
Data are invaluable in machine learning (ML), yet they raise significant privacy concerns. In the real world, data are often distributed across isolated silos, challenging conventional ML methods that centralize data. Federated learning (FL) offers a privacy-preserving solution that enables learning without direct data transfer. Meanwhile, the “right to be forgotten” sparks privacy-preserving methods from another viewpoint as machine unlearning, enabling data owners to erase specific data contributions from ML models. However, the invisibility of data in FL scenarios complicates effective local data removal, necessitating tailored unlearning algorithms for FL. Existing federated unlearning methods fall into approximate unlearning, leaving residual memorization of target data, consequently diminishing user trust. To bridge this gap, we propose FedCIO, a novel framework for exact federated unlearning, designed to efficiently manage precise data removal requests in FL scenarios. Specifically, the framework involves client clustering, isolation among clusters, and one-shot aggregation of cluster models. This framework facilitates efficient unlearning by retraining only a relevant model subset rather than from scratch. To enhance the capability to handle Non-Independent and Identically Distributed (Non-IID) data, we further introduce an advanced spectral clustering implementation based on model similarity for better cluster partitioning. Comprehensive evaluation across common FL datasets with varied distributions demonstrates the superior performance of our proposed framework.
更多查看译文
关键词
federated learning,machine unlearning,federated unlearning,non-IID data,privacy protection
AI 理解论文
溯源树
样例
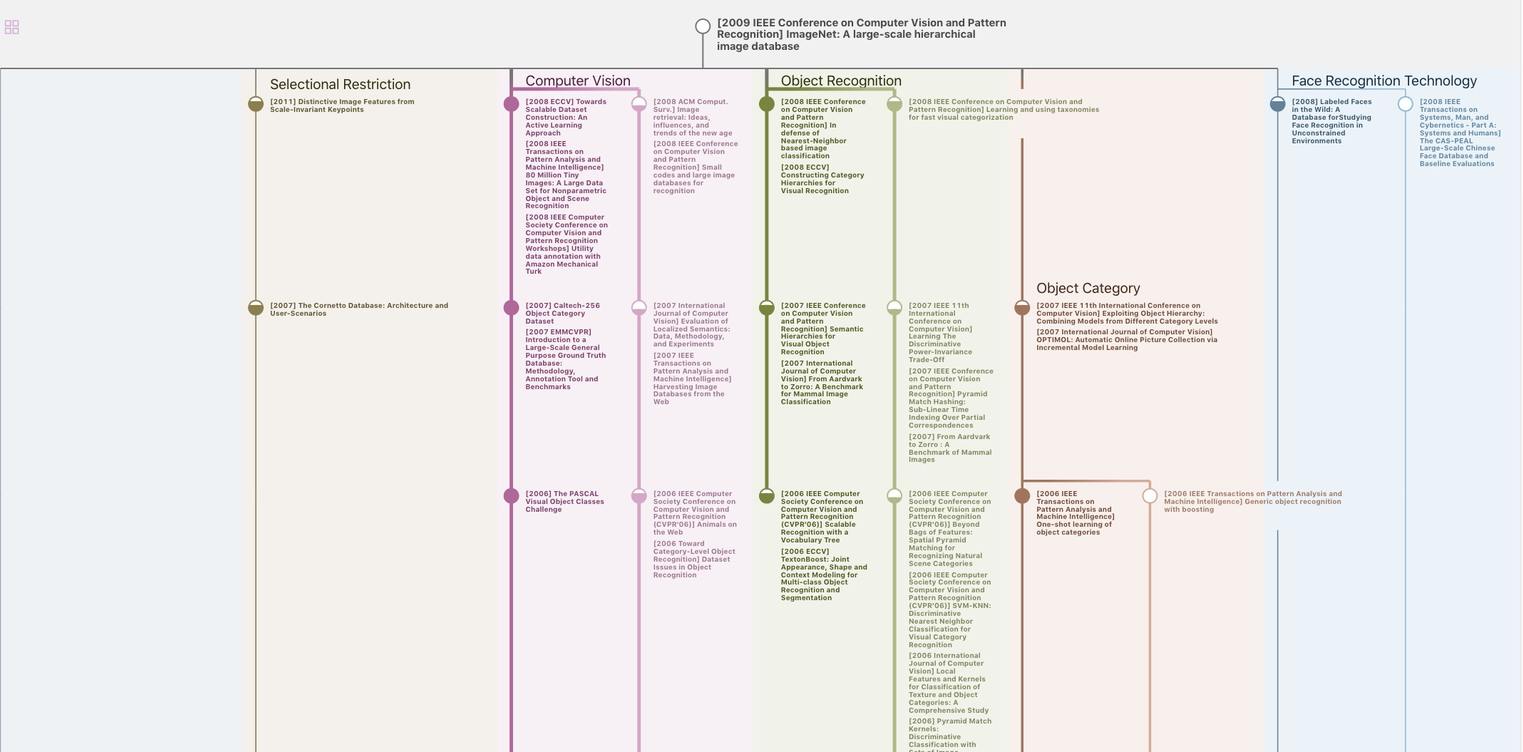
生成溯源树,研究论文发展脉络
Chat Paper
正在生成论文摘要