Source Separation of Piano Concertos Using Musically Motivated Augmentation Techniques
IEEE-ACM TRANSACTIONS ON AUDIO SPEECH AND LANGUAGE PROCESSING(2024)
摘要
In this work, we address the novel and rarely considered source separation task of decomposing piano concerto recordings into separate piano and orchestral tracks. Being a genre written for a pianist typically accompanied by an ensemble or orchestra, piano concertos often involve an intricate interplay of the piano and the entire orchestra, leading to high spectro-temporal correlations between the constituent instruments. Moreover, in the case of piano concertos, the lack of multi-track data for training constitutes another challenge in view of data-driven source separation approaches. As a basis for our work, we adapt existing deep learning (DL) techniques, mainly used for the separation of popular music recordings. In particular, we investigate spectrogram- and waveform-based approaches as well as hybrid models operating in both spectrogram and waveform domains. As a main contribution, we introduce a musically motivated data augmentation approach for training based on artificially generated samples. Furthermore, we systematically investigate the effects of various augmentation techniques for DL-based models. For our experiments, we use a recently published, open-source dataset of multi-track piano concerto recordings. Our main findings demonstrate that the best source separation performance is achieved by a hybrid model when combining all augmentation techniques.
更多查看译文
关键词
Adaptation models,Spectrogram,Source separation,Recording,Music,Speech processing,Instruments,Audio source separation,piano concerto,orchestral music,music processing,music information retrieval
AI 理解论文
溯源树
样例
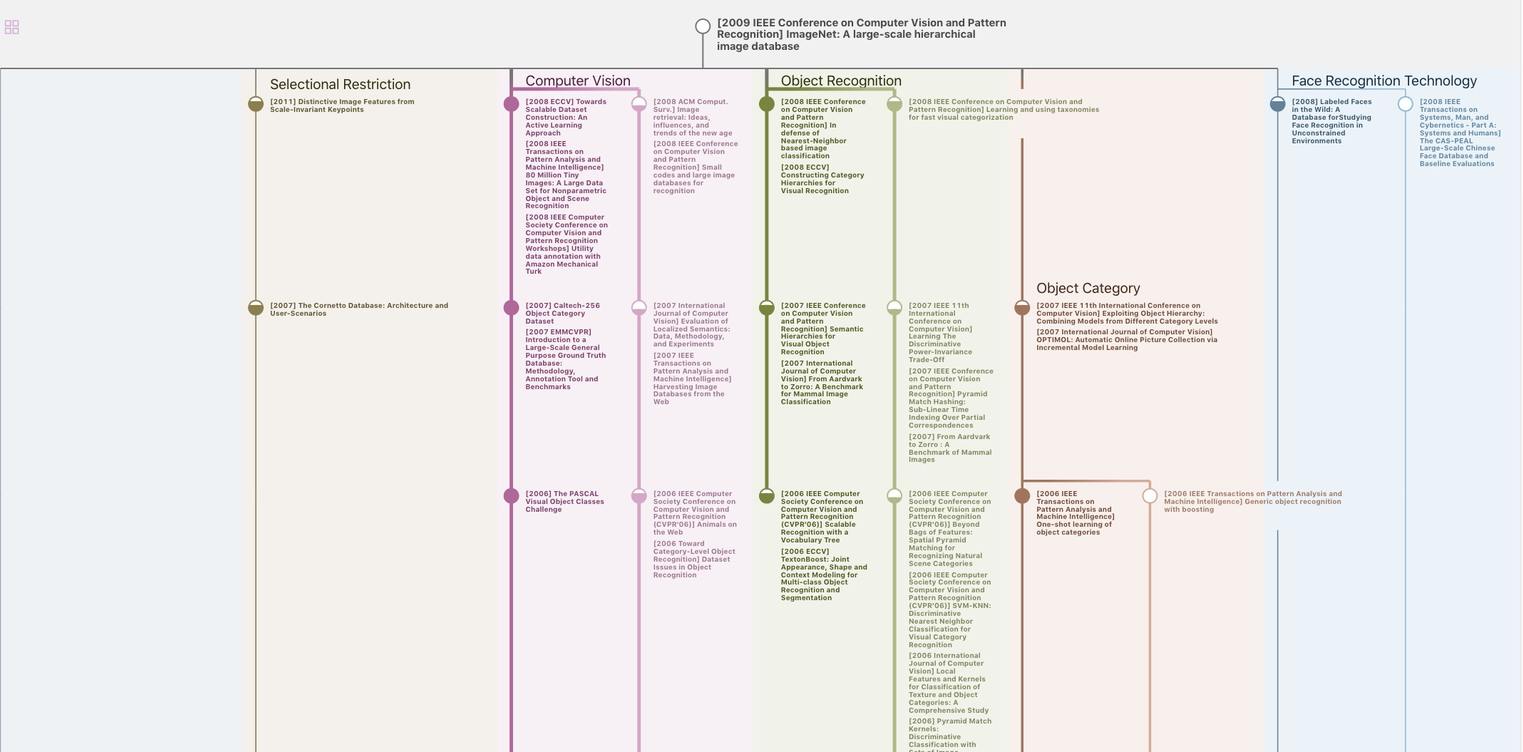
生成溯源树,研究论文发展脉络
Chat Paper
正在生成论文摘要