Optimal Rates for Agnostic Distributed Learning.
IEEE Trans. Inf. Theory(2024)
摘要
The existing optimal rates for distributed kernel ridge regression (DKRR) often rely on a strict assumption, assuming that the true concept belongs to the hypothesis space. However, agnostic distributed learning is more common in practice, where the target regression may lie outside the kernel space. In this paper, we refine the excess risk bounds for DKRR and demonstrate that DKRR still achieve capacity-dependent optimal rates in the agnostic setting. Our theoretical findings indicate that the condition on the number of partitions not only influences computational efficiency but also impacts the range of situations where optimal rates are applicable. To relax the strict condition on the number of partitions, we first derive a sharper estimate for the difference between empirical and expected covariance operators. We then leverage additional unlabeled examples to reduce the label-independent error terms, further extending the optimal rates to more situations in the agnostic setting. In addition to the generalization error bounds in expectation, we also present refined excess risk bounds in high probability, where the optimal rates can also pertain to the agnostic setting. Finally, through both theoretical and empirical comparisons with related work, we demonstrate that our findings provide higher statistical applicability and computational advantages.
更多查看译文
关键词
Distributed kernel ridge regression (DKRR),Capacity-dependent optimal rates,Agnostic learning,High probability bounds
AI 理解论文
溯源树
样例
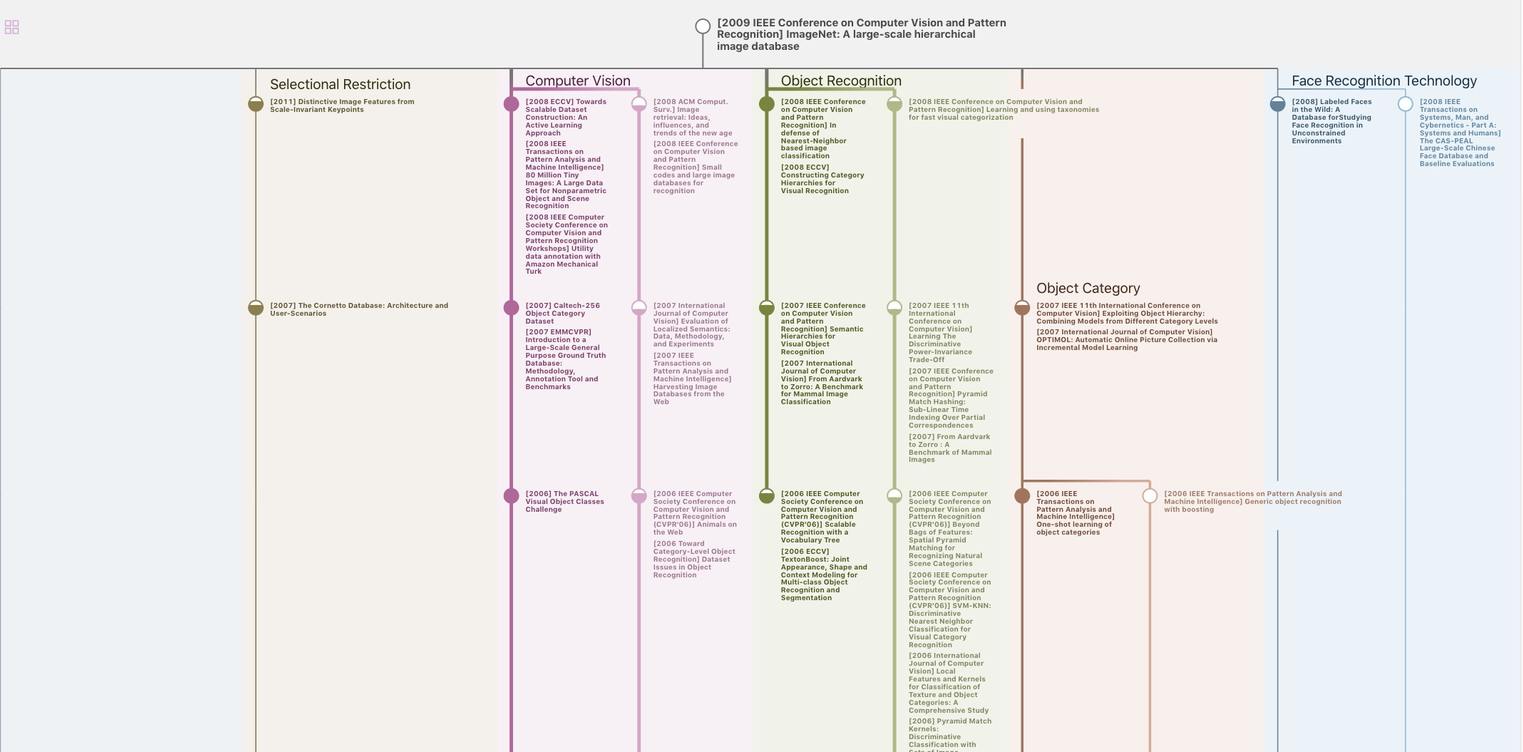
生成溯源树,研究论文发展脉络
Chat Paper
正在生成论文摘要