Achieving Multi-Time-Step Segment Routing via Traffic Prediction and Compressive Sensing Techniques.
IEEE Trans. Netw. Serv. Manag.(2024)
摘要
Traffic engineering (TE) is one of the most critical issues in networking, as it enables efficient and reliable network operations. With the advent of Machine Learning (ML) techniques, many ML-based TE methods have emerged in recent years, especially those employing Deep Neural Networks for future traffic prediction to enhance the performance of traditional approaches. However, current methods suffer from two major issues. Firstly, most prior works only solve the TE problem based on short-term traffic prediction, neglecting the network traffic dynamics over an extended time period. This oversight results in high network disturbance when numerous traffic flows need to be rerouted to adapt to traffic changes. Secondly, although traffic prediction models rely on historical traffic data to perform future prediction, ML-based TE studies often ignore the high overhead for network traffic monitoring. To address these issues, we propose a traffic prediction-based routing algorithm in which the routing rules can be applied to multiple time-steps without requiring changes, ultimately leading to reduced network disturbance. We employ the segment routing (SR) technique as the routing algorithm and formulate the multi-time-step segment routing method that incorporates future traffic prediction. To address the high monitoring overhead, we present an approach that combines partial traffic prediction and compressive sensing techniques to estimate unmeasured data. Through extensive experiments on real backbone network traffic datasets, we demonstrate that our proposal can achieve more than 80% of the optimal performance in reducing maximum link utilization while significantly reducing the number of routing changes and traffic monitoring cost.
更多查看译文
关键词
Traffic engineering,segment routing,traffic prediction,graph neural network,network monitoring,compressive sensing
AI 理解论文
溯源树
样例
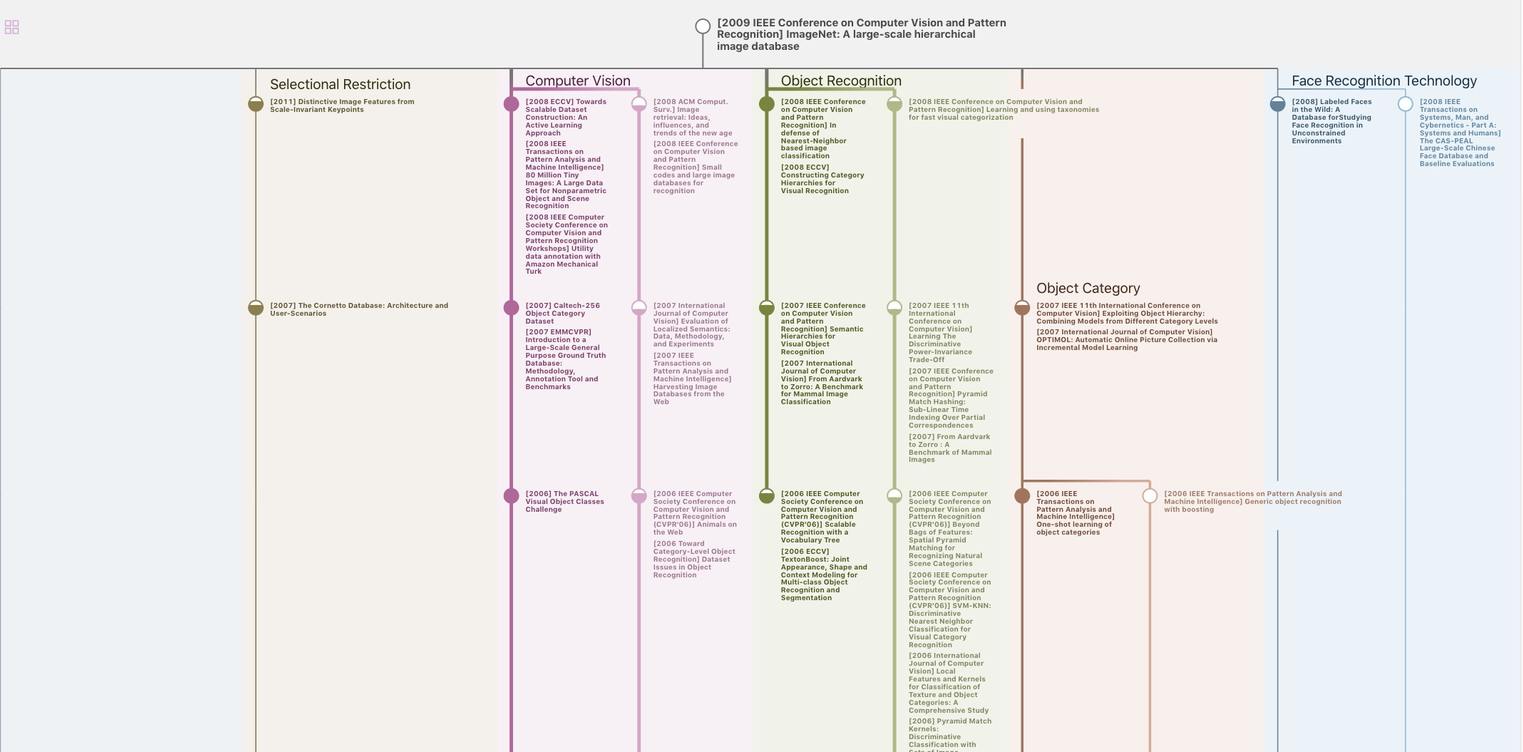
生成溯源树,研究论文发展脉络
Chat Paper
正在生成论文摘要