Fraud's Bargain Attack: Generating Adversarial Text Samples via Word Manipulation Process
IEEE Transactions on Knowledge and Data Engineering(2024)
摘要
Recent research has revealed that natural language processing (NLP) models are vulnerable to adversarial examples. However, the current techniques for generating such examples rely on deterministic heuristic rules, which fail to produce optimal adversarial examples. In response, this study proposes a new method called the Fraud's Bargain Attack (FBA), which uses a randomization mechanism to expand the search space and produce high-quality adversarial examples with a higher probability of success. FBA uses the Metropolis-Hasting sampler, a type of Markov Chain Monte Carlo sampler, to improve the selection of adversarial examples from all candidates generated by a customized stochastic process called the Word Manipulation Process (WMP). The WMP method modifies individual words in a contextually-aware manner through insertion, removal, or substitution. Through extensive experiments, this study demonstrates that FBA outperforms other methods in terms of attack success rate, imperceptibility and sentence quality.
更多查看译文
关键词
Adversarial learning,evasion attacks,natural language processing
AI 理解论文
溯源树
样例
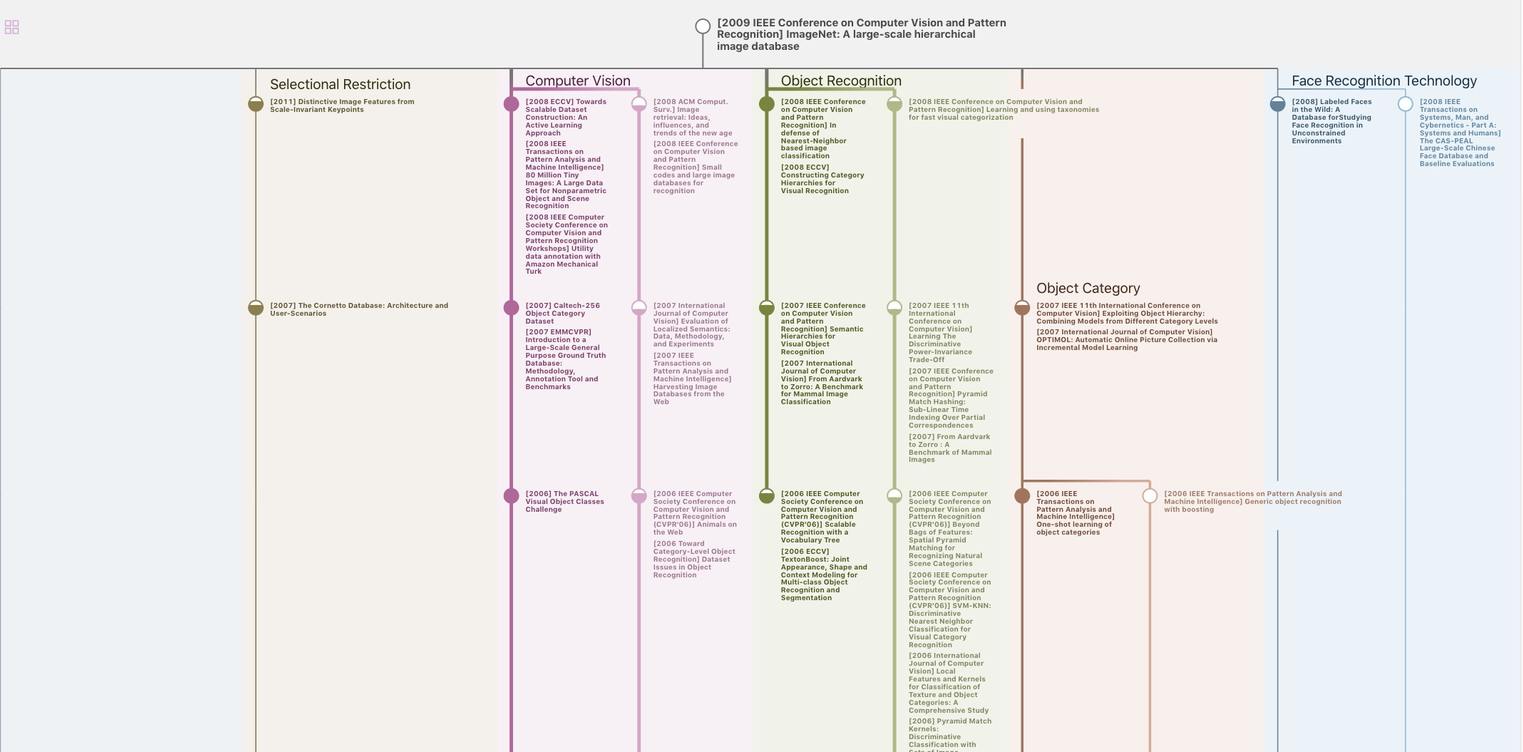
生成溯源树,研究论文发展脉络
Chat Paper
正在生成论文摘要