ARES: On Adversarial Robustness Enhancement for Image Steganographic Cost Learning
IEEE Transactions on Multimedia(2024)
摘要
Taking the steganalytic discriminators as the adversaries, the existing Generative Adversarial Networks (GAN)-based steganographic approaches learn the implicit cost functions to measure the embedding distortion for steganography. However, the steganalytic discriminators in these approaches are trained by the stego-samples with insufficient diversity, and their network structures offer very limited representational capacity. As a result, these steganalytic discriminators will not exhibit robustness to various steganographic patterns, which causes learning suboptimal cost functions, thus compromising the anti-steganalysis capability. To address this issue, we propose a novel GAN-based steganographic approach, in which the Diversified Inverse-Adversarial Training (DIAT) strategy and the Steganalytic Feature Attention (SteFA) structure are designed to train a robust steganalytic discriminator. Specifically, the DIAT strategy provides the steganalytic discriminator with an expanded feature space by generating diversified adversarial stego-samples; the SteFA structure enables the steganalytic discriminator to capture more various steganalytic features by employing the channel-attention mechanism on higher-order statistics. Consequently, the steganalytic discriminator can build a more precise decision boundary to make it more robust, which facilitates learning a superior steganographic cost function. Extensive experiments demonstrate that the proposed steganographic approach achieves promising anti-steganalysis capability over the state-of-the-arts under the same embedding payloads.
更多查看译文
关键词
Steganography,GAN,Adversarial training,Cost learning
AI 理解论文
溯源树
样例
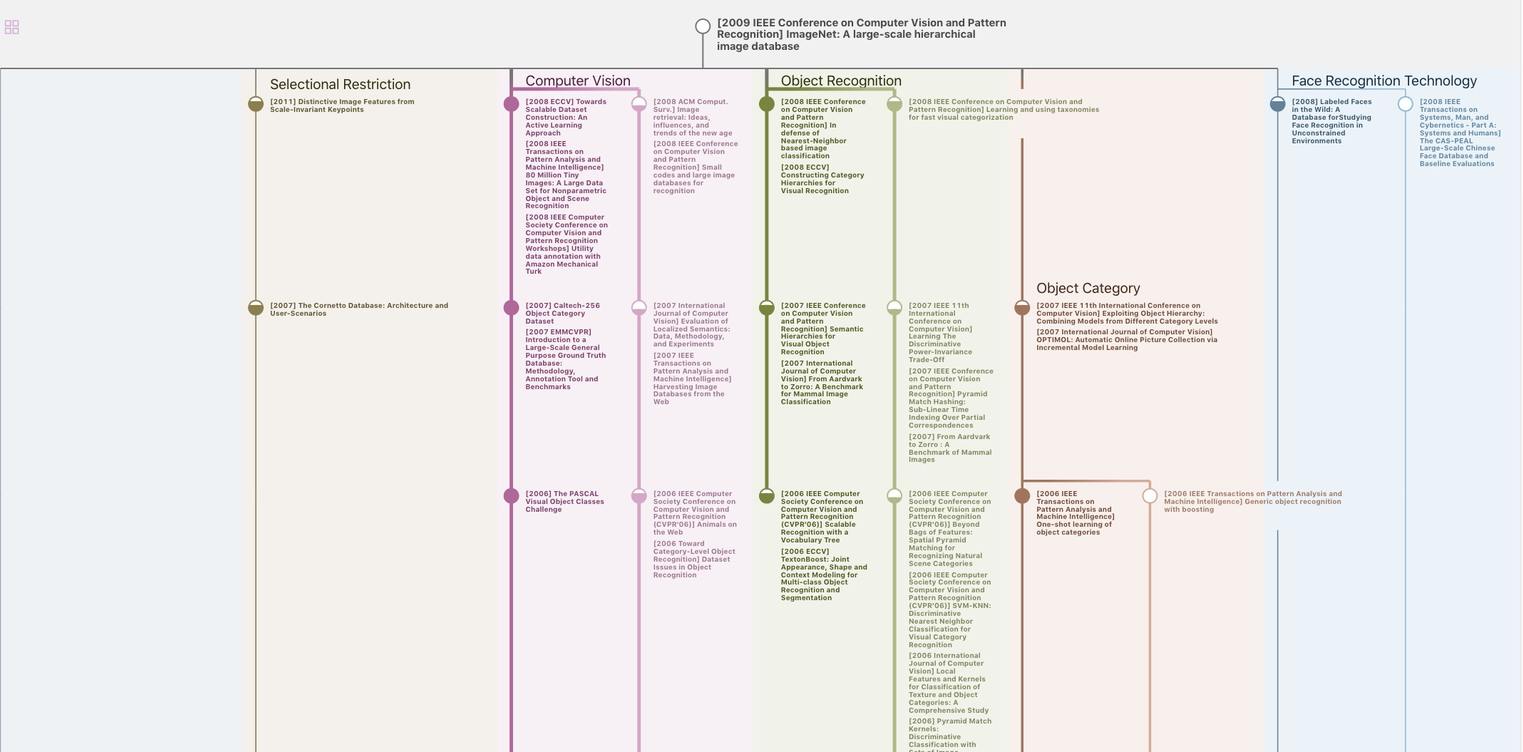
生成溯源树,研究论文发展脉络
Chat Paper
正在生成论文摘要