Robust Beamforming for Massive MIMO LEO Satellite Communications: A Risk-Aware Learning Framework
IEEE Transactions on Vehicular Technology(2023)
摘要
This paper proposes a robust beamforming algorithm for massive multiple-input multiple-output (MIMO) low earth-orbit (LEO) satellite communications under uncertain channel conditions. Specifically, a risk-aware optimization problem is formulated to optimize the hybrid digital and analog precoding aiming at maximizing the energy efficiency of the LEO satellite while considering the required quality-of-service (QoS) by each ground user. The Conditional Value at Risk (CVaR) is used as a risk measure of the downlink data rate to capture the high dynamic and random channel variations due to satellite movement, achieving the required QoS under the worst-case scenario. A deep reinforcement learning (DRL) based framework is developed to solve the formulated stochastic problem over time slots. Considering the limited computation capabilities of the LEO satellite, the training process of the proposed learning algorithm is performed offline at a central terrestrial server. The trained models are then sent periodically to the LEO satellite through ground stations to provide online executions on the transmit precoding based on the current network state. Simulation results demonstrate the efficacy of the proposed approach in achieving the QoS requirements under uncertain wireless channel conditions.
更多查看译文
关键词
6G,LEO satellites communication,NTN,massive MIMO,risk-aware learning,DRL,digital precoding,analog beamforming
AI 理解论文
溯源树
样例
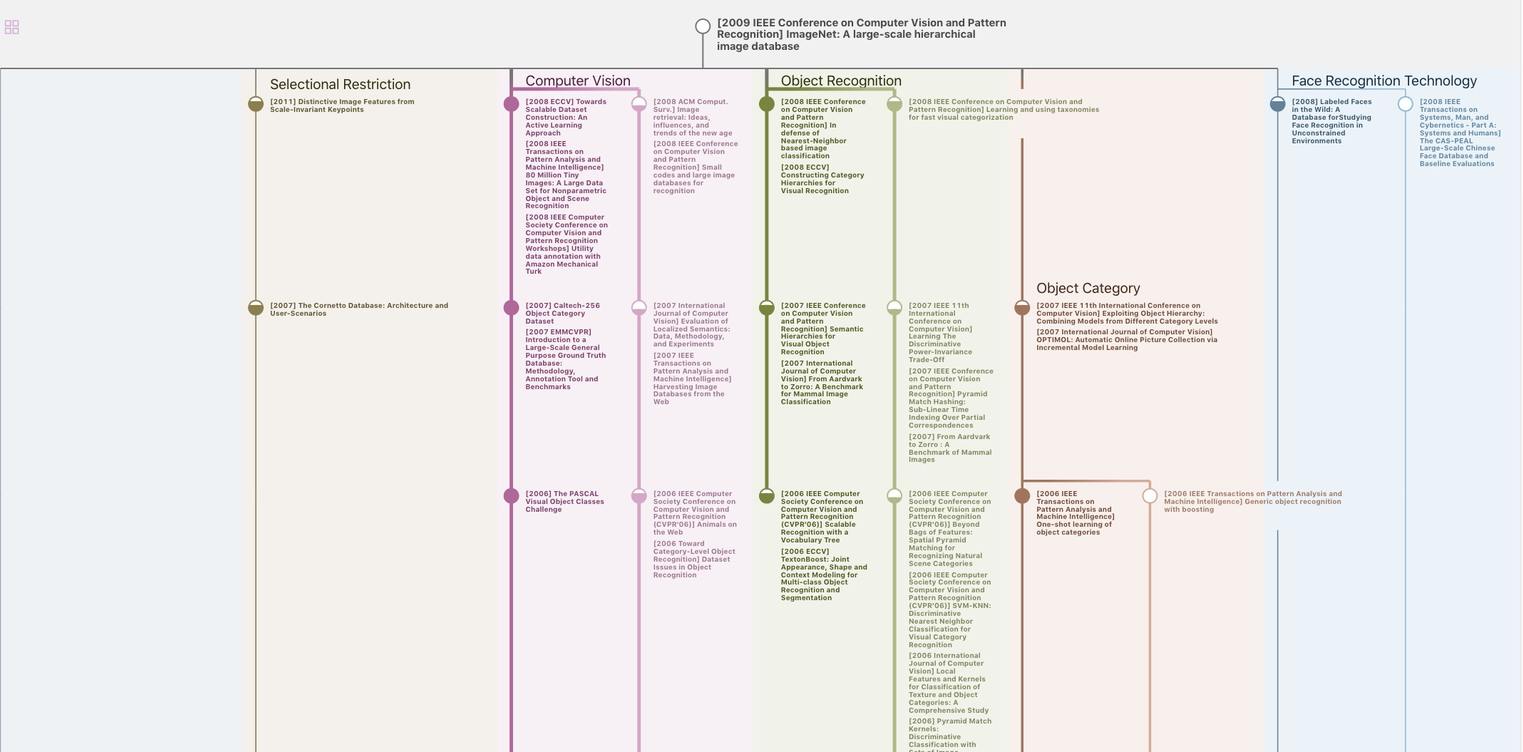
生成溯源树,研究论文发展脉络
Chat Paper
正在生成论文摘要