A Novel Fall Detection Framework with Age Estimation Based on Cloud-fog Computing Architecture
IEEE Sensors Journal(2023)
摘要
Falls are one of the main causes of injuries and even fatalities among the elderly. However, few existing research on fall detection focuses on the supervision of the elderly. Furthermore, the face image of the target usually exhibits low resolution in the surveillance videos, posing a challenge in achieving a balance between the response latency and the computational cost. To this end, a novel fall detection framework with age estimation based on cloud-fog computing architecture is proposed in this paper. Specifically, an optimized Soft Stage Regression-Shallow (SSR-S) network is presented to achieve excellent performance on age estimation for the low-resolution images collected by the edge layer. A fall pre-judgment mechanism and a Shallow-Convolutional Neural Networks (S-CNN) are proposed to make a better judgment on fall behaviors among different age groups at the fog layer and the cloud layer respectively. Besides, an age estimation-based priority algorithm is presented to prioritize the people aged 60 or older in fall detection at the cloud layer, with the aim to make a trade-off between the response latency and the computational overhead. Finally, extensive simulations have been conducted to evaluate the performance of our proposal. Experimental results have shown that the minimum Mean Absolute Error (MAE) of SSR-S reaches 7.59. The fall prejudgment mechanism can achieve 0% miss rate, and the accuracy of S-CNN reaches 90.5%. The detection speed of the overall framework is 17.0 frames per second (FPS).
更多查看译文
关键词
Age estimation,fall prejudgment,distributed model,scheduling algorithm
AI 理解论文
溯源树
样例
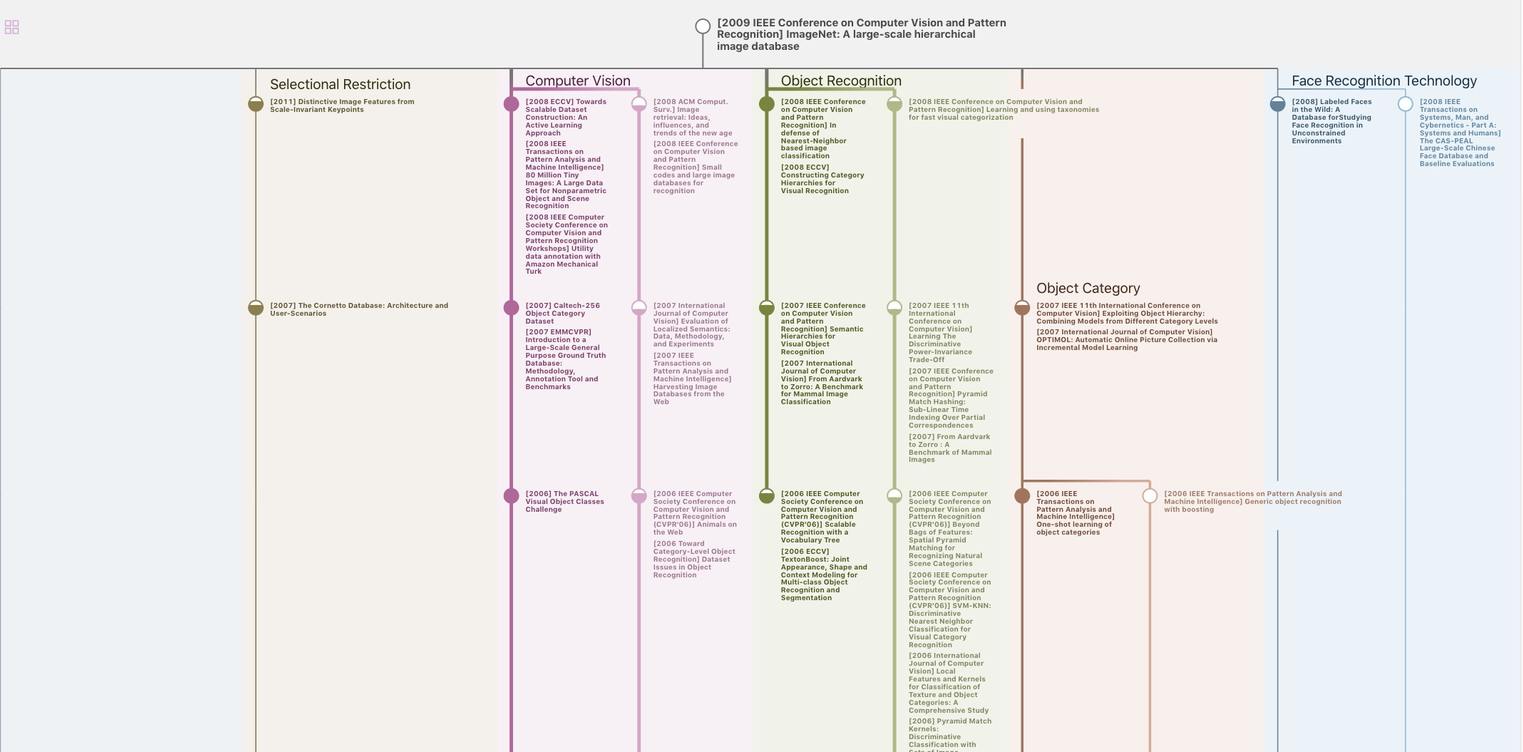
生成溯源树,研究论文发展脉络
Chat Paper
正在生成论文摘要