Snippet-to-Prototype Contrastive Consensus Network for Weakly Supervised Temporal Action Localization
IEEE Transactions on Multimedia(2024)
摘要
Weakly-supervised temporal action localization aims to localize action instances from untrimmed videos with only video-level labels. Due to the lack of frame-wise annotations, most methods embrace a localization-by-classification paradigm. However, the large supervision gap between classification and localization hinders models from obtaining accurate snippet-wise classification sequences and action proposals. We propose a snippet-to-prototype contrastive consensus network (SPCC-Net) to simultaneously generate feature-level and label-level supervision information to narrow the supervision gap between classification and localization. Specifically, the network adopts a two-stream framework incorporating the optical flow and fusion streams to fully leverage the motion and complementary information from multiple modalities. Firstly, the snippet-to-prototype contrast module is executed within each stream to learn prototypes for all categories and contrast them with action snippets to guarantee intra-class compactness and inter-class separability of snippet features. Secondly, for generating accurate label-level supervision information through complementary information of multimodal features, the multi-modality consensus module ensures not only category consistency through knowledge distillation but also semantic consistency through contrastive learning. Finally, we introduce the auxiliary multiple instance learning (MIL) loss to alleviate the issue that existing MIL-based methods only localize sparse discriminative snippets. Extensive experiments are conducted on two public datasets, THUMOS-14 and ActivityNet-1.3, to demonstrate the superior performance of our method over state-of-the-art methods.
更多查看译文
关键词
Weakly-supervised temporal action localization,contrastive learning,knowledge distillation
AI 理解论文
溯源树
样例
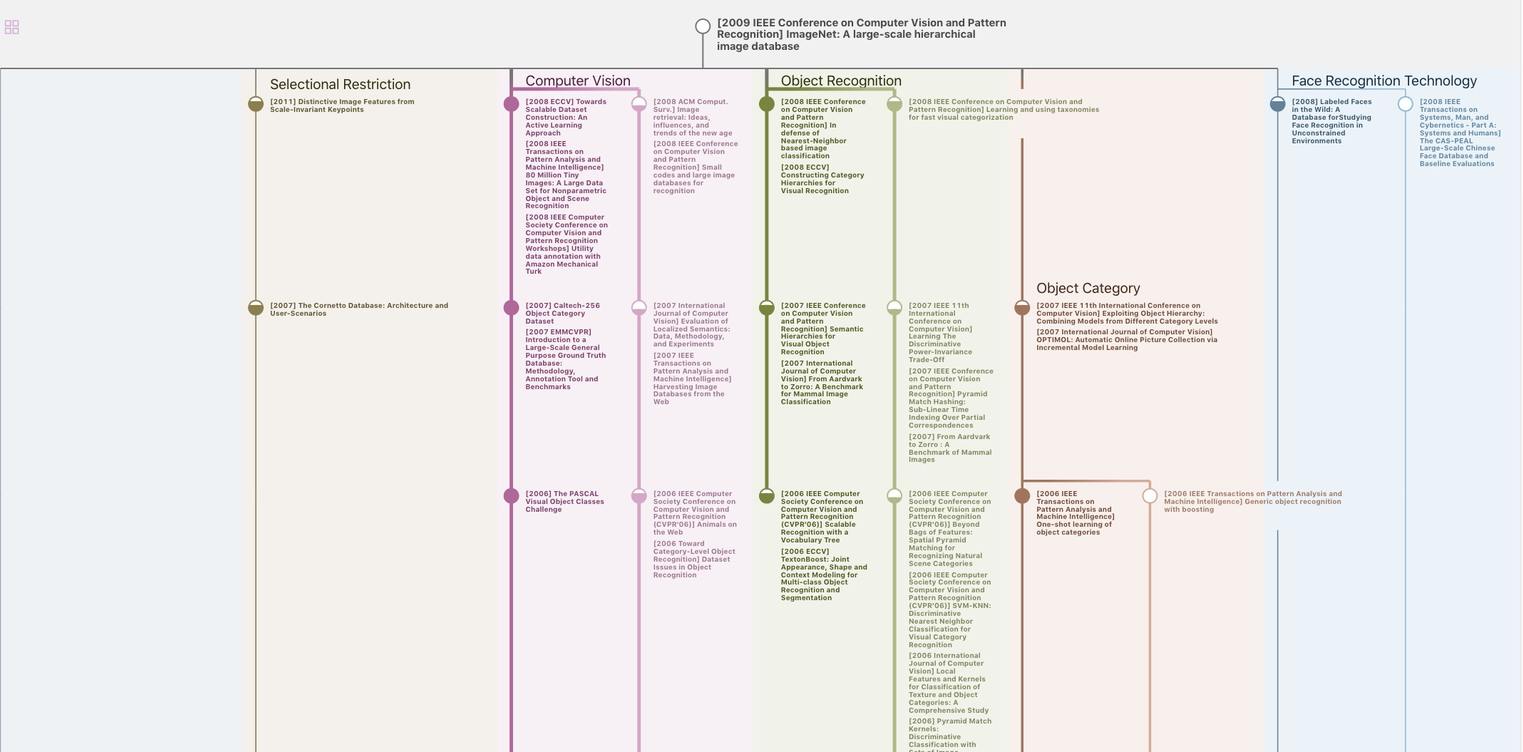
生成溯源树,研究论文发展脉络
Chat Paper
正在生成论文摘要