Improving Deepfake Detection Generalization by Invariant Risk Minimization
IEEE Transactions on Multimedia(2024)
摘要
The abuse of deepfake techniques has raised serious concerns about social security and ethical problems, which motivates the development of deepfake detection. However, without fully addressing the domain gap issue, existing deepfake detection methods still show weak generalization ability among datasets belonging to different domains with domain-specific characteristics like identities and generation methods, limiting their practical applications. In this paper, we propose the
Invariant Domain-oriented Deepfake Detection method (ID$_{3}$ )
, which improves the generalization of deepfake detection on multiple domains through invariant risk minimization, a novel learning paradigm that addresses the domain gap problem by jointly training a purified invariant predictor and learning an aligned invariant representation. To train a purified invariant predictor, we design the
Domain Refinement Data Augmentation
strategy with self-face-swapping and region-erasing approaches, which suppresses domain-specific features and encourages the models to focus on critical domain-invariant characteristics. To learn an aligned invariant representation, we propose the
Domain Calibration Batch Normalization
approach with multiple BN branches, which normalizes input features from different domains into aligned representations during both training and testing. Extensive experiments on multiple datasets demonstrate that our framework can boost the deepfake detection generalization ability and outperform other baselines by large margins. Our codes can be found here
更多查看译文
关键词
Deepfake detection,model generalization,invariant risk minimization
AI 理解论文
溯源树
样例
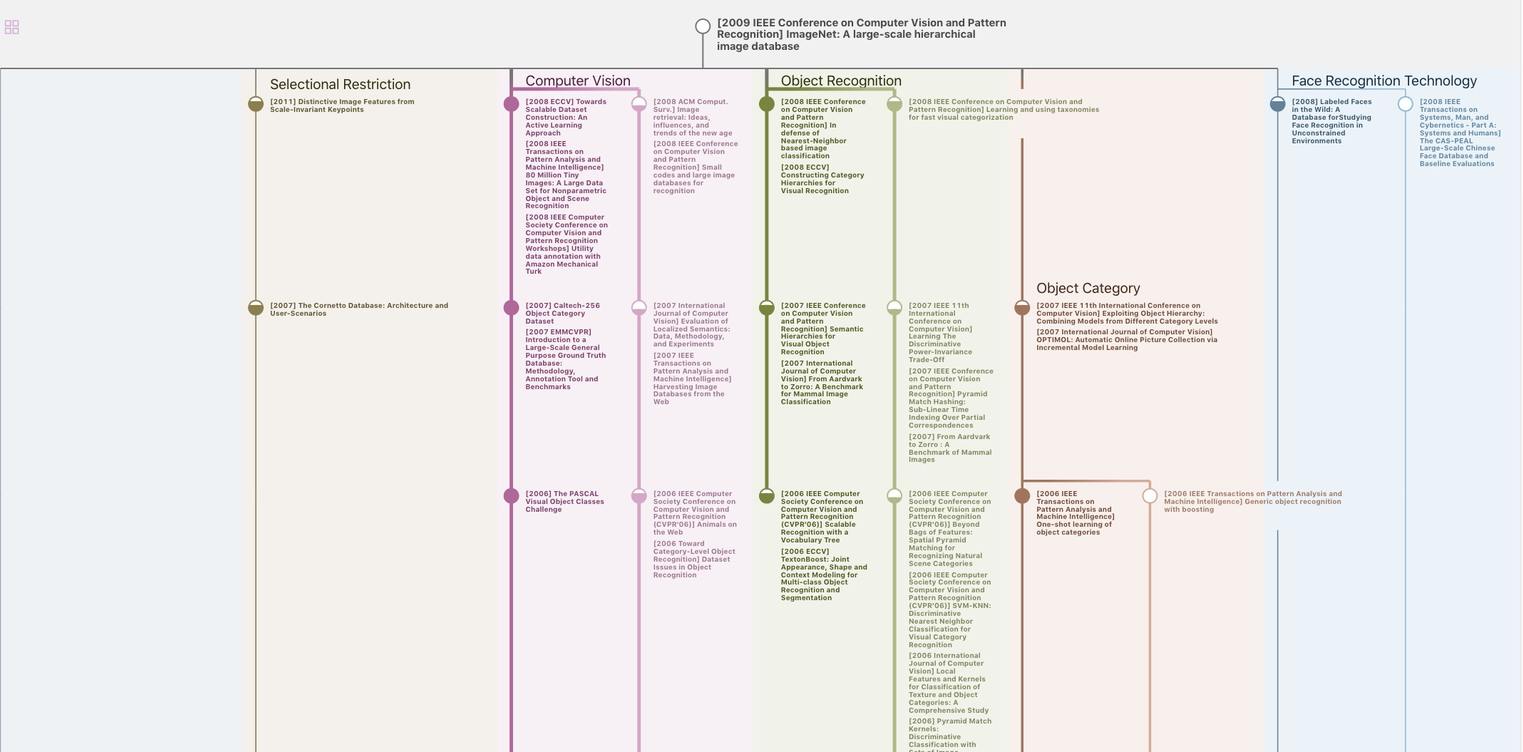
生成溯源树,研究论文发展脉络
Chat Paper
正在生成论文摘要