BiaS: Incorporating Biased Knowledge to Boost Unsupervised Image Anomaly Localization
IEEE TRANSACTIONS ON SYSTEMS MAN CYBERNETICS-SYSTEMS(2024)
摘要
Image anomaly localization is a pivotal technique in industrial inspection, often manifesting as a supervised task where abundant normal samples coexist with rare abnormal samples. Existing supervised methods in this context are prone to overfitting, as they primarily encounter anomalies that represent only a fraction of the open-world anomalies. Conversely, unsupervised methods excel in performance, yet they disregard the essential biased knowledge pertaining to both seen and unseen anomalies within the open world. To bridge this gap and refine unsupervised methods for supervised applications, this study introduces a comprehensive framework called biased students (BiaS), mainly comprising a three-step strategy. This strategy encompasses biased knowledge generation, transfer, and fusion. BiaS effectively segregates the vast anomaly space into two subsets: 1) unseen anomalies and 2) seen anomalies. Subsequently, it generates specialized biased knowledge for these subsets and transfers this knowledge to two distinct subnetworks. As a result, one subnetwork becomes adept at detecting unseen anomalies, while the other excels in localizing seen anomalies. To optimize their capabilities, BiaS synergistically fuses these subnetworks based on their expertise. Rigorous experimentation has empirically validated the effectiveness, generality, and scalability of BiaS, underscoring its potential to enhance unsupervised methods and effectively address the challenges of supervised anomaly localization.
更多查看译文
关键词
Location awareness,Task analysis,Knowledge engineering,Inspection,Training,Testing,Sun,Anomaly localization,disentangle learning,few-shot learning,knowledge distillation
AI 理解论文
溯源树
样例
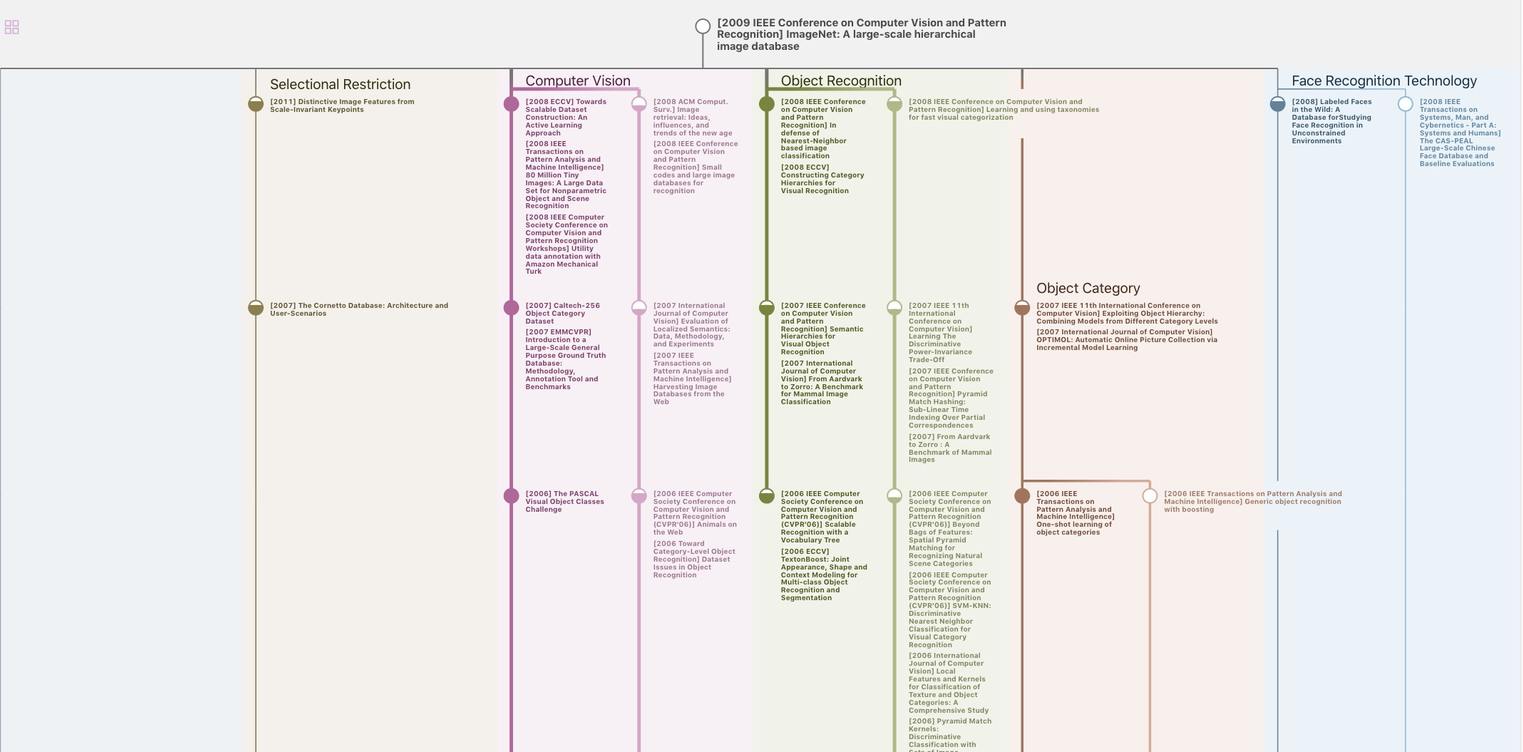
生成溯源树,研究论文发展脉络
Chat Paper
正在生成论文摘要