Generative-Adversarial-Based Feature Compensation to Predict Quality of Service.
IEEE Trans. Serv. Comput.(2024)
摘要
Predicting quality of service (QoS) is an important issue in the field of service recommendation that has been widely studied in the past few years. Many current methods predict QoS values based on the historical invocation records of services, but most of them ignore the time-varying characteristics of these values. Capturing time-varying characteristics to ensure accurate prediction of QoS values has become a key problem in the area. To solve this problem, in this paper, we first apply probabilistic matrix factorization in QoS time series of sparse QoS matrices to extract time-varying feature series of users and services. Then we construct a gated feature extraction network (GFEN) to compensate for feature loss due to matrix factorization and enrich the limited information due to the sparsity of QoS matrices, where the heart of GFEN is an enhanced gated recurrent unit (EGRU) and a generative adversarial network is proposed to train GFEN. Extensive experimental results show that the proposed model outperforms state-of-the-art methods in terms of QoS prediction accuracy.
更多查看译文
关键词
Sparse QoS matrix,generative adversarial network,feature compensation,QoS prediction
AI 理解论文
溯源树
样例
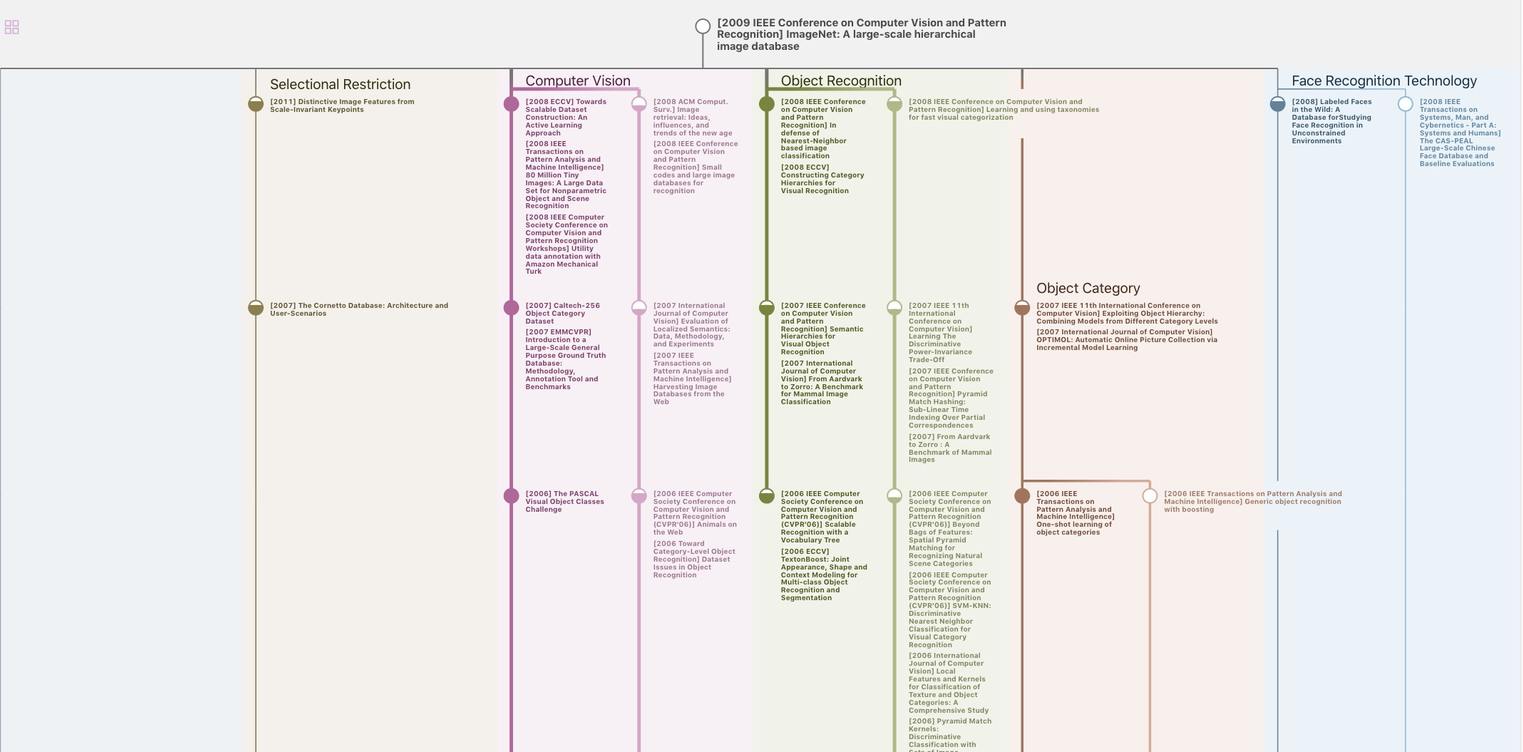
生成溯源树,研究论文发展脉络
Chat Paper
正在生成论文摘要