A 4-bit Calibration-Free Computing-In-Memory Macro With 3T1C Current-Programed Dynamic-Cascode Multi-Level-Cell eDRAM
IEEE JOURNAL OF SOLID-STATE CIRCUITS(2023)
摘要
Analog computing-in-memory (CIM) has been widely explored for computing neural networks (NNs) efficiently. However, most analog CIM implementations trade compute accuracy for energy efficiency. The low accuracy restricts the practical application of analog CIM. In this article, a current-programming CIM that unifies the weight programming and computing in the current domain is proposed to address this dilemma. The enabled technique is a novel 3-transistor 1-capacitor (3T1C) embedded dynamic random access memory (eDRAM) cell. The current-programming mechanism and the dynamic-cascode read structure of the 3T1C cell make it immune to transistor-level non-idealities, including nonlinear I - V , threshold voltage variations, and short-channel effect. Therefore, the cell enables multi-level-cell (MLC) operations without any calibration, supporting eight current-weight levels (0-700 nA). In addition, a voltage-current two-step programming scheme is proposed to boost the sub-microamphere current-weight writing speed. To support signed 4-b weights, a pseudo-differential CIM cell composed of two 3T1C MLCs is developed. Fabricated in a 65-nm CMOS, the prototype demonstrates 2.2x reduction in macro-level variation through current programming. Benefiting from sub-microamphere compute currents, the prototype achieves the 4-b energy efficiencies of 233-304 TOPS/W. With a refresh interval of 0.4 ms, the macro achieves > 90% inference accuracy on CIFAR10.
更多查看译文
关键词
Analog,computing-in-memory (CIM),current programming,dynamic cascode,embedded dynamic random access memory (eDRAM),multi-level-cell (MLC),neural network (NN),variation
AI 理解论文
溯源树
样例
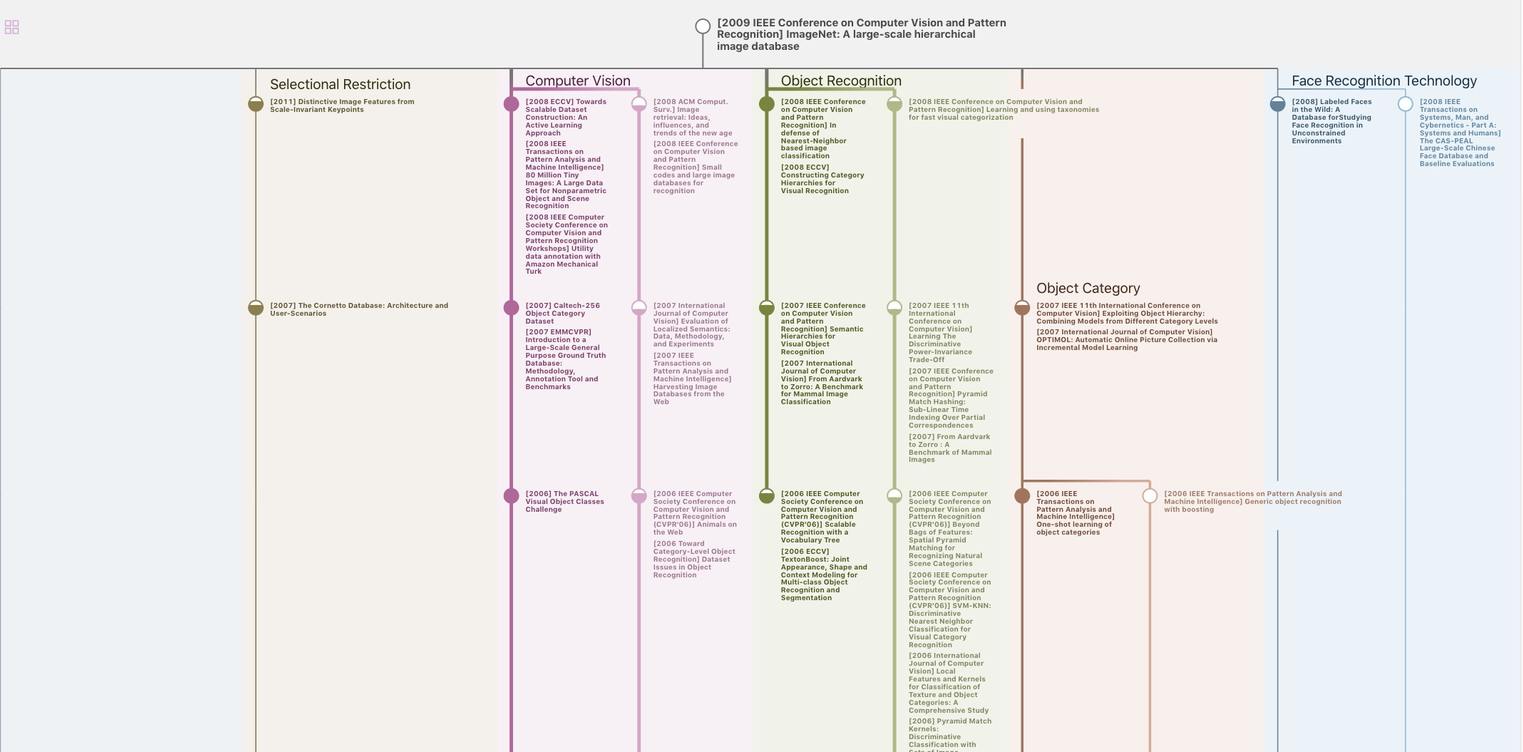
生成溯源树,研究论文发展脉络
Chat Paper
正在生成论文摘要