Securing Fast and High-Precision Localization for Shallow Underground Explosive Source: A Curiosity-Driven Deep Reinforcement Learning Approach
IEEE JOURNAL OF SELECTED TOPICS IN APPLIED EARTH OBSERVATIONS AND REMOTE SENSING(2024)
摘要
Shallow underground explosive source localization technology is a key technology in the field of underground space localization. The existing approaches mainly aim to improve the localization accuracy, but need to deploy enormous sensors in the monitoring area, and rely on a large number of backend workstations to solve. These methods have the defects of considerable calculation and high time cost, and are hard to satisfy the precise and real-time requirements of onsite testing, ultimately resulting in slow localization speed and accurate localization failure. Fortunately, emerging deep reinforcement learning can effectively solve the problem of slow search policy by modeling the source localization as a Markov decision process (MDP). Therefore, a curiosity-driven deep dueling double Q-learning network (C-D3QN) is subsequently proposed to solve the above MDP. The overestimation problem is solved by decoupling selection and evaluation of the bootstrap action, and the action difference is effectively increased by introducing the dueling network that separately represents state values and action advantages. Meanwhile, the exploration is jointly reinforced by an intrinsic reward outputted from the curiosity module and an extrinsic reward supplied by the environment, guaranteeing the convergence to global optimal. Finally, extensive simulation results based on the outfield experiment data show that compared with other algorithms, the proposed scheme can significantly improve exploration ability and learning speed as well as generalization and robustness. In addition, compared to the baseline algorithm deep Q-learning network, the C-D3QN algorithm can offer an improved localization accuracy as high as 99.62% and an increased localization speed of 66.23%.
更多查看译文
关键词
Location awareness,Vibrations,Optimization,Deep learning,Explosives,Monitoring,Three-dimensional displays,Curiosity-driven deep reinforcement learning (DRL),dueling neural network,underground source localization,vibration energy field
AI 理解论文
溯源树
样例
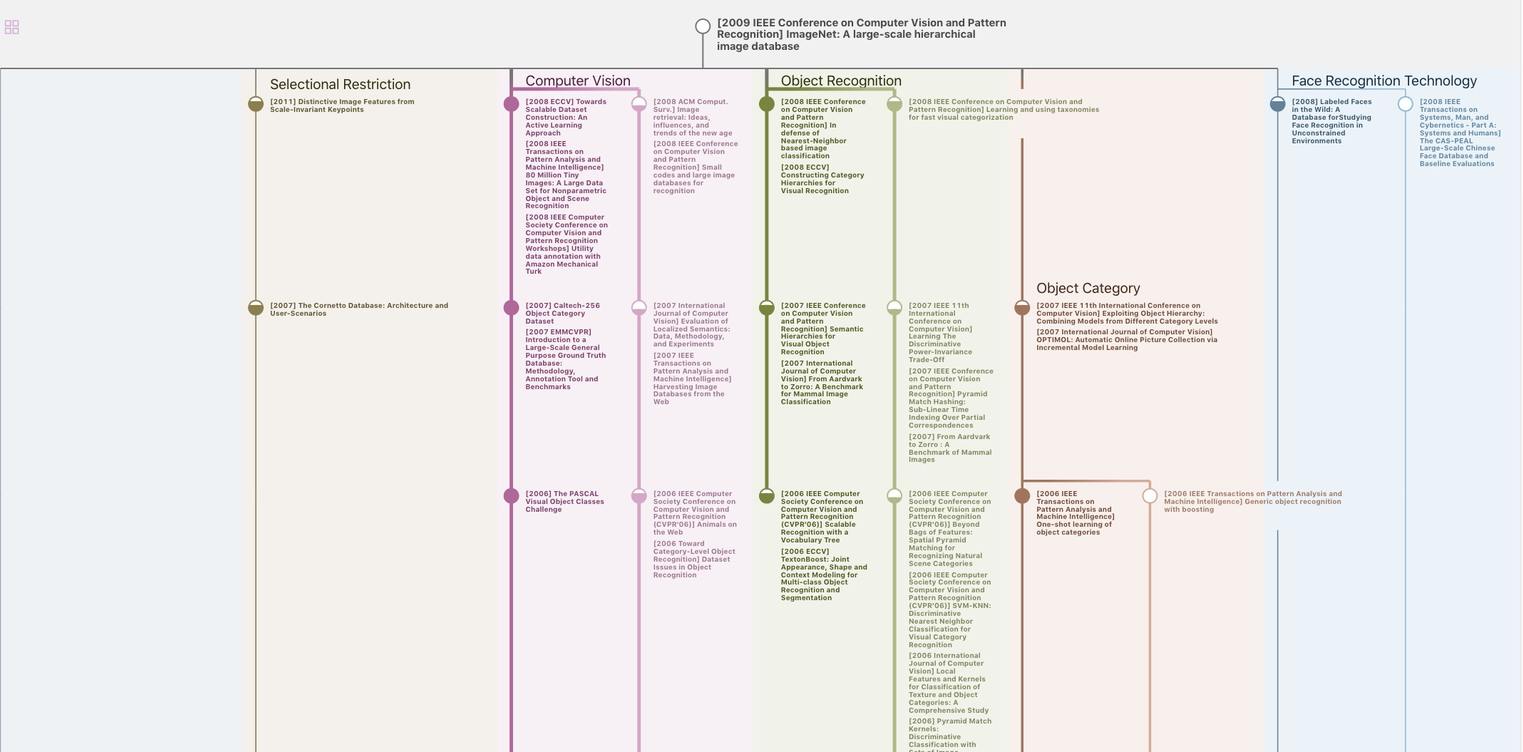
生成溯源树,研究论文发展脉络
Chat Paper
正在生成论文摘要