Spatio-Temporal Identity Multi-Graph Convolutional Network for Traffic Prediction in the Metaverse
IEEE JOURNAL ON SELECTED AREAS IN COMMUNICATIONS(2024)
摘要
The metaverse is at the forefront of the next-generation internet application, where billions of users seamlessly immerse themselves in a hybrid reality of physical-virtual worlds and switch between virtual environments thanks to reliable resource allocation and synchronization. However, the exponential growth of users and computationally intensive applications make joint optimization of multiple indicators challenging. Therefore, predicting user behavior is pivotal in assisting the optimization process. Although graph neural networks have demonstrated remarkable performance in traffic prediction, most existing schemes link nodes based on their distances and require significant computational resources, limiting their generalization and deployment in the metaverse. To solve this problem, we propose an efficient Spatio-temporal Identity Multi-graph convolutional network Framework (SIMF) for application-level traffic prediction in the metaverse. In the SIMF, we design a spatio-temporal embedding layer and multi-graph convolutional module to jointly capture spatio-temporal correlations among nodes (avatars) and reduce the dependence on topology information, which is more consistent with the real relationship between avatars in the metaverse. We conduct extensive experiments to evaluate the SIMF, which show that our proposed framework achieves superior accuracy even without graph information while maintaining low time complexity, making it suitable for traffic prediction in the metaverse.
更多查看译文
关键词
Metaverse,Avatars,Resource management,Graph neural networks,Costs,Correlation,Synchronization,traffic prediction,graph neural network,digital twin,artificial intelligence
AI 理解论文
溯源树
样例
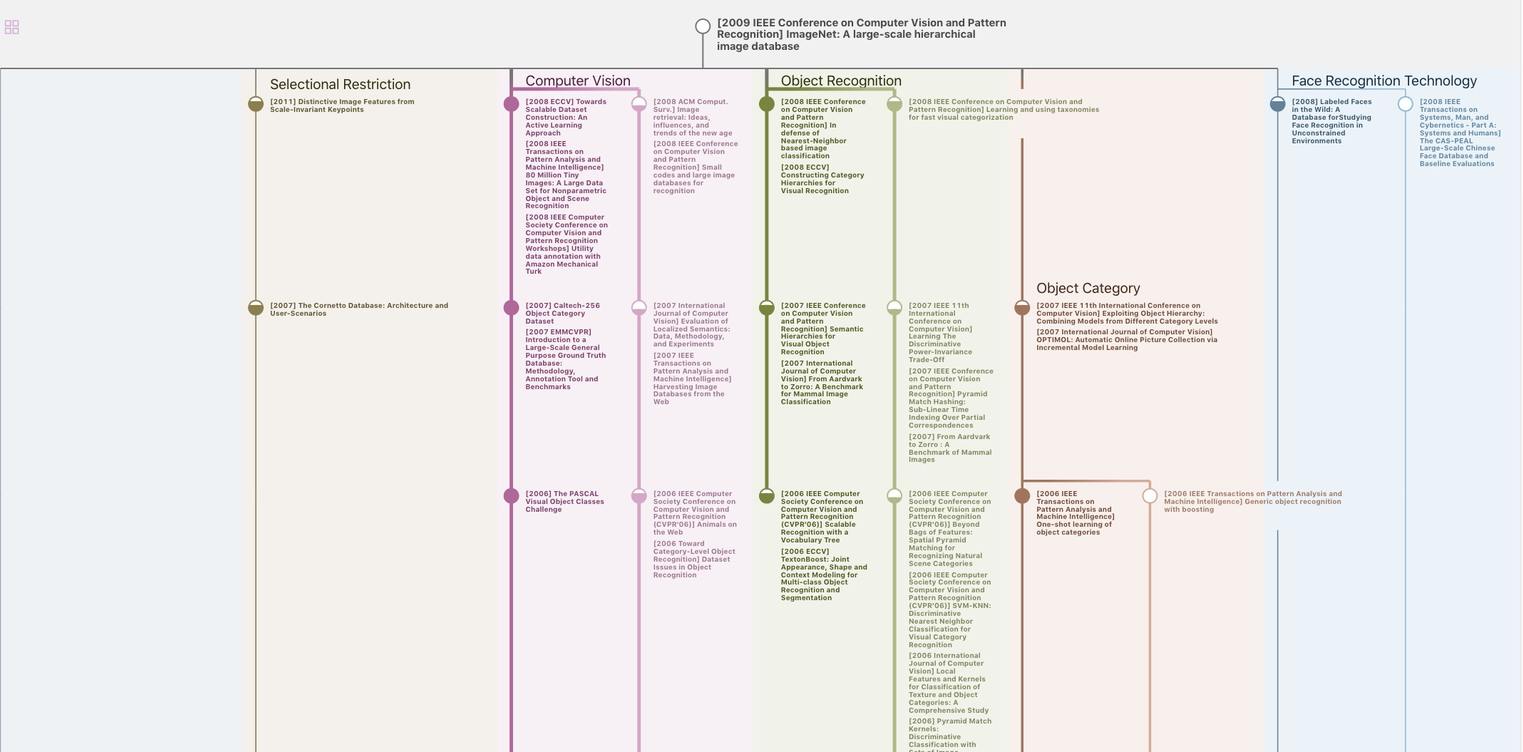
生成溯源树,研究论文发展脉络
Chat Paper
正在生成论文摘要