Gaussian Mixture Model Uncertainty Modeling for Power Systems Considering Mutual Assistance of Latent Variables
IEEE Transactions on Sustainable Energy(2024)
摘要
The accurate modeling of uncertain variables, such as wind power and load uncertainty, is an essential foundation for the planning operation and scheduling decisions in power systems. Currently, Gaussian mixture model (GMM) and Dirichlet process mixture model (DPMM) are the primary techniques used to characterize uncertainties in power systems, which are commonly solved by expectation-maximization (EM) algorithm. However, for the massive data of uncertain variables, the algorithm encounters challenges in accurately obtaining GMM and DPMM with a lower time consumption. To address this issue, we propose a method for GMM uncertainty modeling in power systems considering the mutual assistance of latent variables. Specifically, the GMM of uncertain variables is first constructed, and the conditional probability is employed to characterize the mutual assistance of latent variables. Then, an improved EM algorithm is developed to obtain the optimal GMM parameters, in which the expectation step (E-step) and maximization step (M-step) of the algorithm are revised using the conditional probability. Importantly, the closed-form solutions for GMM parameters are rederived in the revised E-step and M-step. Finally, the proposed uncertainty modeling method is compared with the traditional GMM and DPMM on actual wind power and load data from Australia. The proposed method performs the efficiency and accuracy in characterizing uncertainty.
更多查看译文
关键词
Gaussian mixture model,uncertainty modeling,latent variables,EM algorithm
AI 理解论文
溯源树
样例
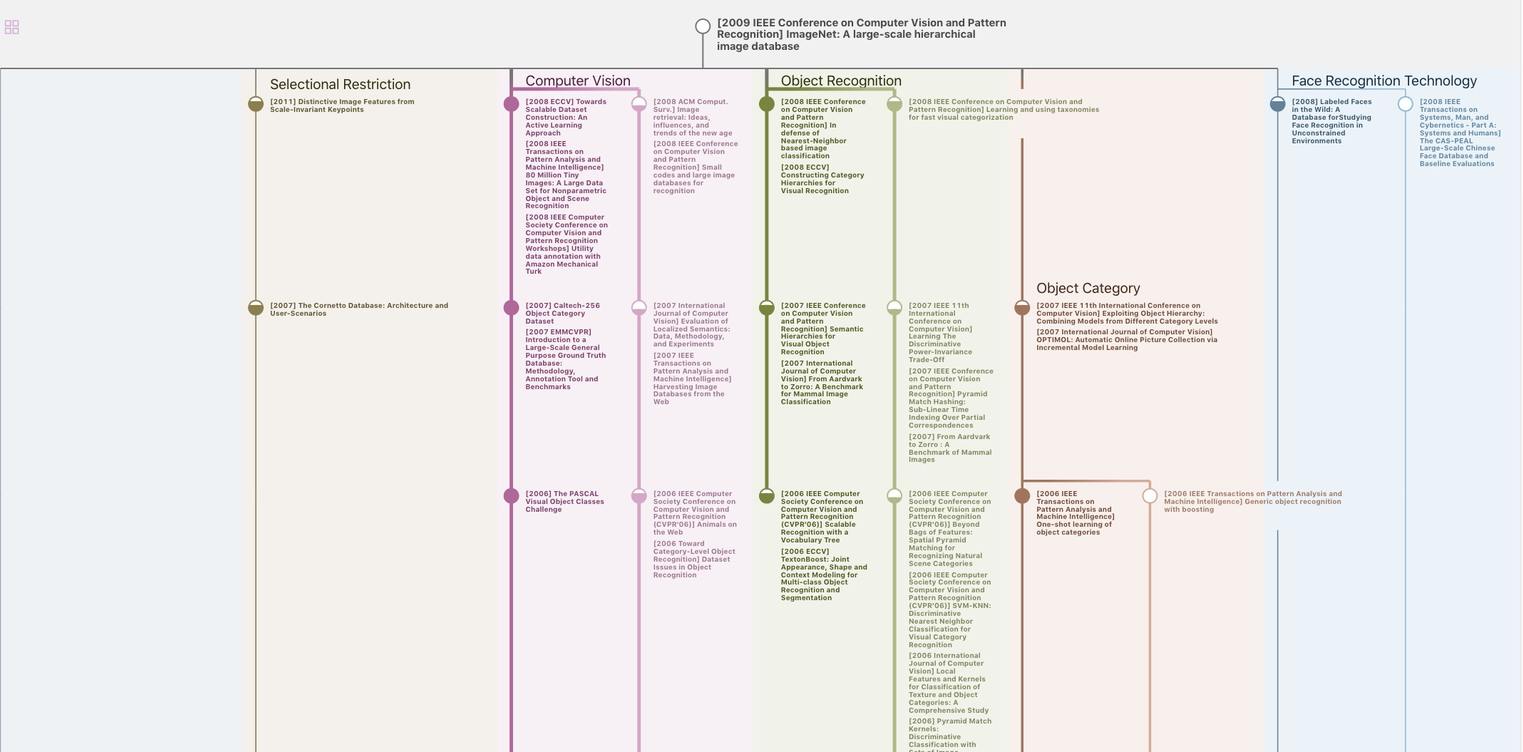
生成溯源树,研究论文发展脉络
Chat Paper
正在生成论文摘要