Multiscale BLS-Based Lightweight Prediction Model for Remaining Useful Life of Aero-Engine
IEEE TRANSACTIONS ON RELIABILITY(2024)
摘要
Remaining useful life (RUL) prediction of aero-engines is one of the important issues in research related to engine health management. Although deep learning has made great progress in fault diagnosis research, successful training of deep learning models is very time-consuming and difficult to meet the real-time requirements of online RUL prediction applications. Broad learning systems (BLS) provide an alternative to deep learning networks with low computational resource requirements, fast training time, and incremental scalability. Based on the typical BLS, we propose a new lightweight multiscale BLS (MSBLS). Considering that RUL is influenced by the working condition factor, the discrete wavelet transform is used to generate multiresolution components, and then feature nodes are extracted on top of the components. An elastic net regularization technique is used to constrain the output weights of the nodes, preserving the significant nodes, and finally obtaining a more sparse MSBLS. Experiments are conducted using the NASA publicly available commercial modular aero-propulsion system simulation (C-MAPSS) dataset and the N-CMAPSS dataset, and our proposed MSBLS not only improves the accuracy of RUL prediction but also has a very short training time compared with the latest research methods nowadays.
更多查看译文
关键词
Predictive models,Feature extraction,Engines,Aircraft propulsion,Discrete wavelet transforms,Real-time systems,Atmospheric modeling,Aero-engines,broad learning system (BLS),discrete wavelet transforms (DWT),elastic net regularization,remaining useful life (RUL)
AI 理解论文
溯源树
样例
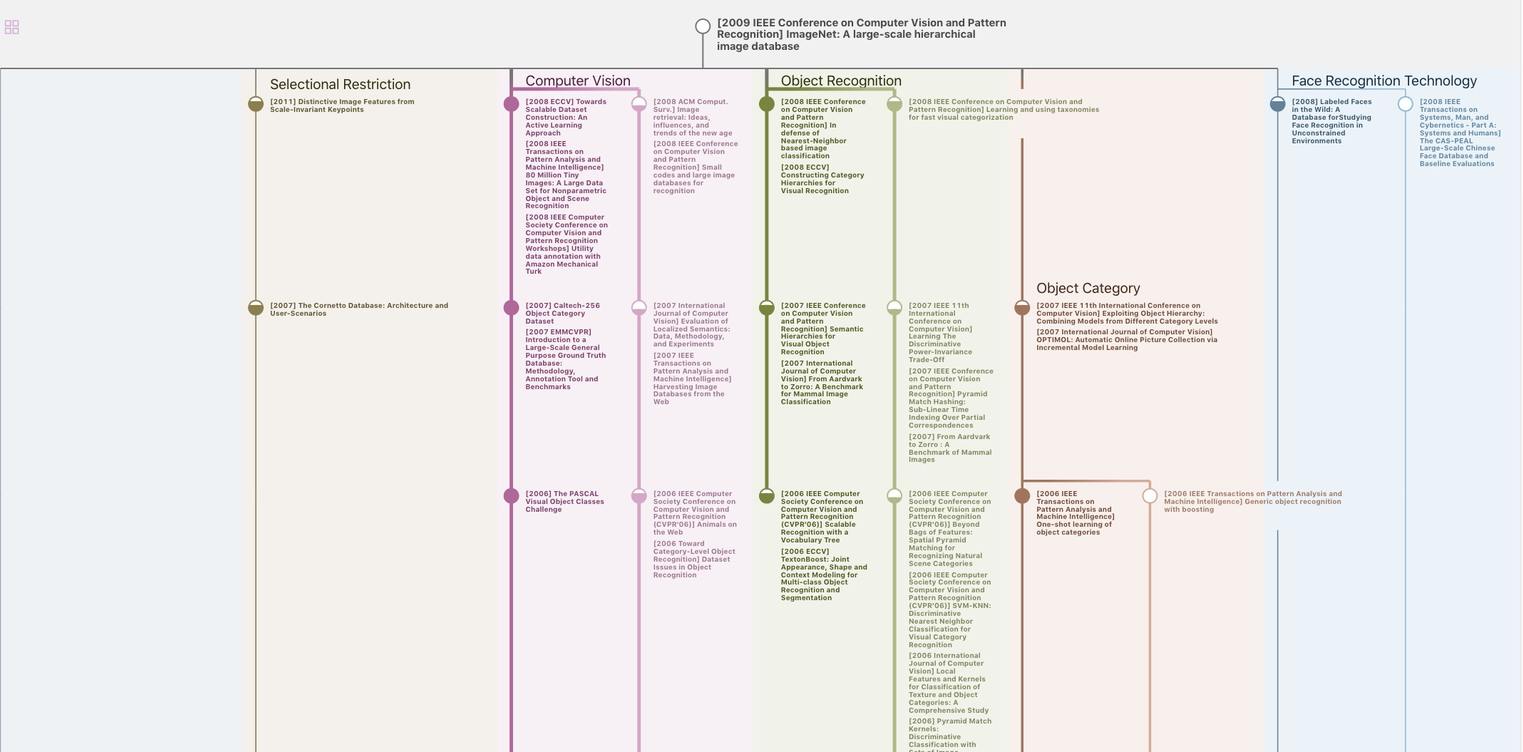
生成溯源树,研究论文发展脉络
Chat Paper
正在生成论文摘要