Cas-PRED: A Limited-Angle Cone Beam CT Reconstruction Research Based on Prior Information
IEEE TRANSACTIONS ON INSTRUMENTATION AND MEASUREMENT(2024)
摘要
Due to limitations in radiation dose or scanning conditions, there are instances where computed tomography (CT) data can only be collected within a restricted scanning angle. However, the use of limited-angle projection data often results in reconstructed images that exhibit significant noise and artifacts, which can significantly impact diagnostic accuracy. Conventional iterative reconstruction algorithms, such as the total variation (TV) algorithm, consume considerable time simulation of the cone beam forward and backward projection processes. Furthermore, these algorithms often struggle to address the challenges of limited-angle reconstruction due to their inherent limitations. To tackle these challenges and achieve superior reconstruction outcomes, we introduce a novel approach known as the cascaded prior-based residual encoder-decoder (Cas-PRED) network. This method employs a multilevel cascaded network as its core architecture, leveraging full-angle CT reconstruction results as prior information to guide the deep learning network's training process. Our approach consistently delivers superior results compared with the existing reconstruction methods, with impressive performance metrics, including a peak signal-to-noise ratio (PSNR) of 33.69 +/- 0.90 dB, a structural similarity index (SSIM) of 0.9557 +/- 0.0029, and a root-mean-square error (RMSE) of 22.73 +/- 1.56 for the result of [0(degrees), 150(degrees)] reconstruction, highlighting its effectiveness in enhancing image quality and accuracy.
更多查看译文
关键词
Cone beam computed tomography (CT) reconstruction,deep learning,limited angle,prior information
AI 理解论文
溯源树
样例
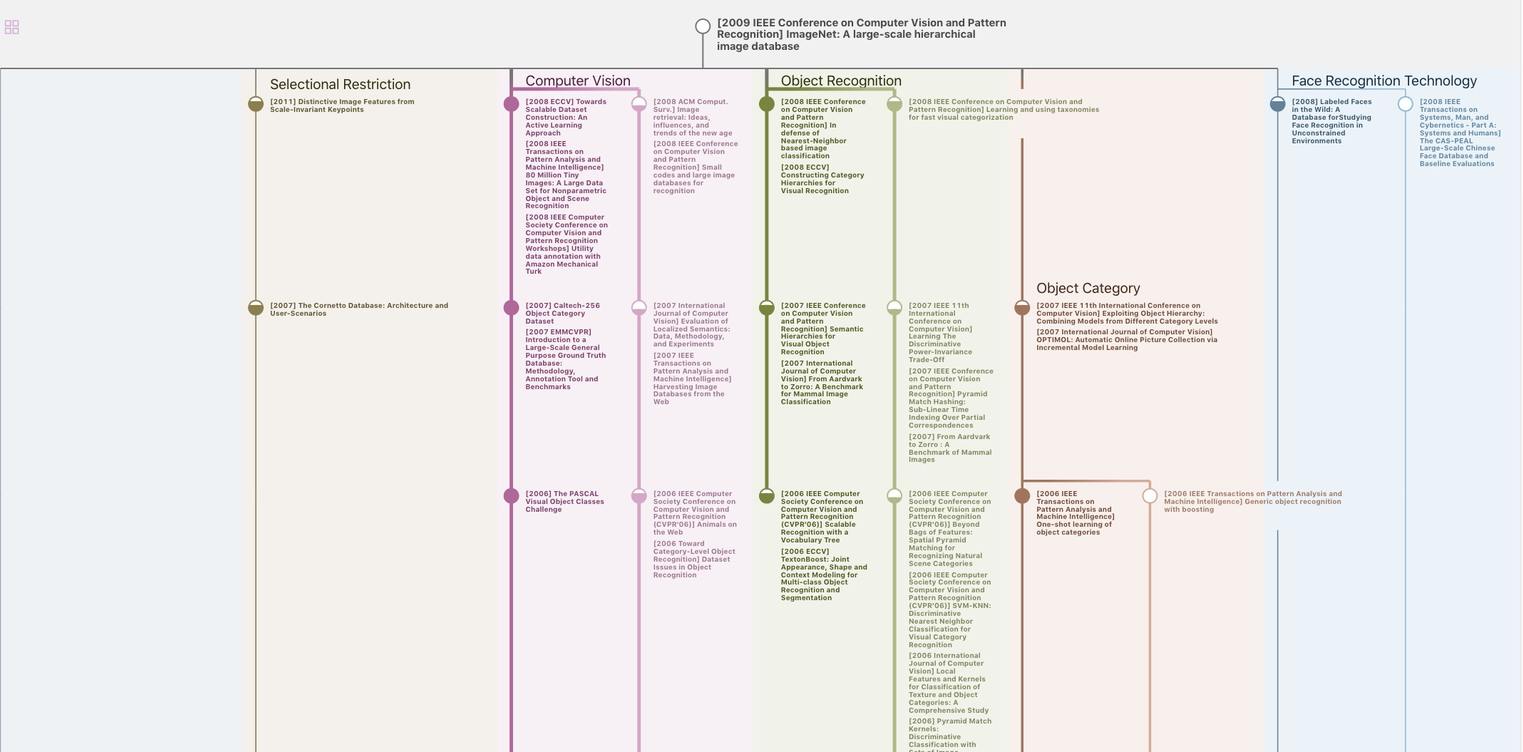
生成溯源树,研究论文发展脉络
Chat Paper
正在生成论文摘要