Edge-Carrier-Assisted Phase Retrieval at Low-CSPR and Low-Dispersion Diversity With Deep Learning
JOURNAL OF LIGHTWAVE TECHNOLOGY(2024)
摘要
We investigate the performance of deep learning in recovering the complex-valued field of a weak-carrier-assisted single side-band signal from two intensity measurements that are decorrelated by dispersion. The proposed scheme relies on a supervised learning-based convolutional neural network to map the intensity measurements to the full-field. Unlike conventional iterative phase retrieval schemes, the proposed scheme does not require any iterations, digital upsampling, or pilot symbols, and can operate both at low carrier-to-signal-power ratio (CSPR) and at low applied dispersion value. Through numerical simulations in relevant system settings, we compare the performance of the proposed scheme with two recently proposed carrier-assisted iterative phase retrieval schemes: one based on the solution of a nonlinear optimization problem, and the other based on a modified Gerchberg-Saxton algorithm. The results show that the proposed scheme complies with the 7% hard-decision forward error correction threshold after 24 GBaud 32-QAM transmission over 100 km of standard single-mode fiber at a CSPR of 0 dB, with 3.6 times lower applied dispersion value, 30% to 90% lower complexity, and with less than 2 dB sensitivity penalty compared to conventional iterative phase retrieval schemes. These results support the potential of deep learning to realize phase retrieval-based coherent receivers that are compatible with the low complexity requirements of short-reach optical networks.
更多查看译文
关键词
Phase retrieval,coherent receiver,neural network,Gerchberg-Saxton algorithm,carrier-to-signal-power ratio,direct-detection,short-reach communications
AI 理解论文
溯源树
样例
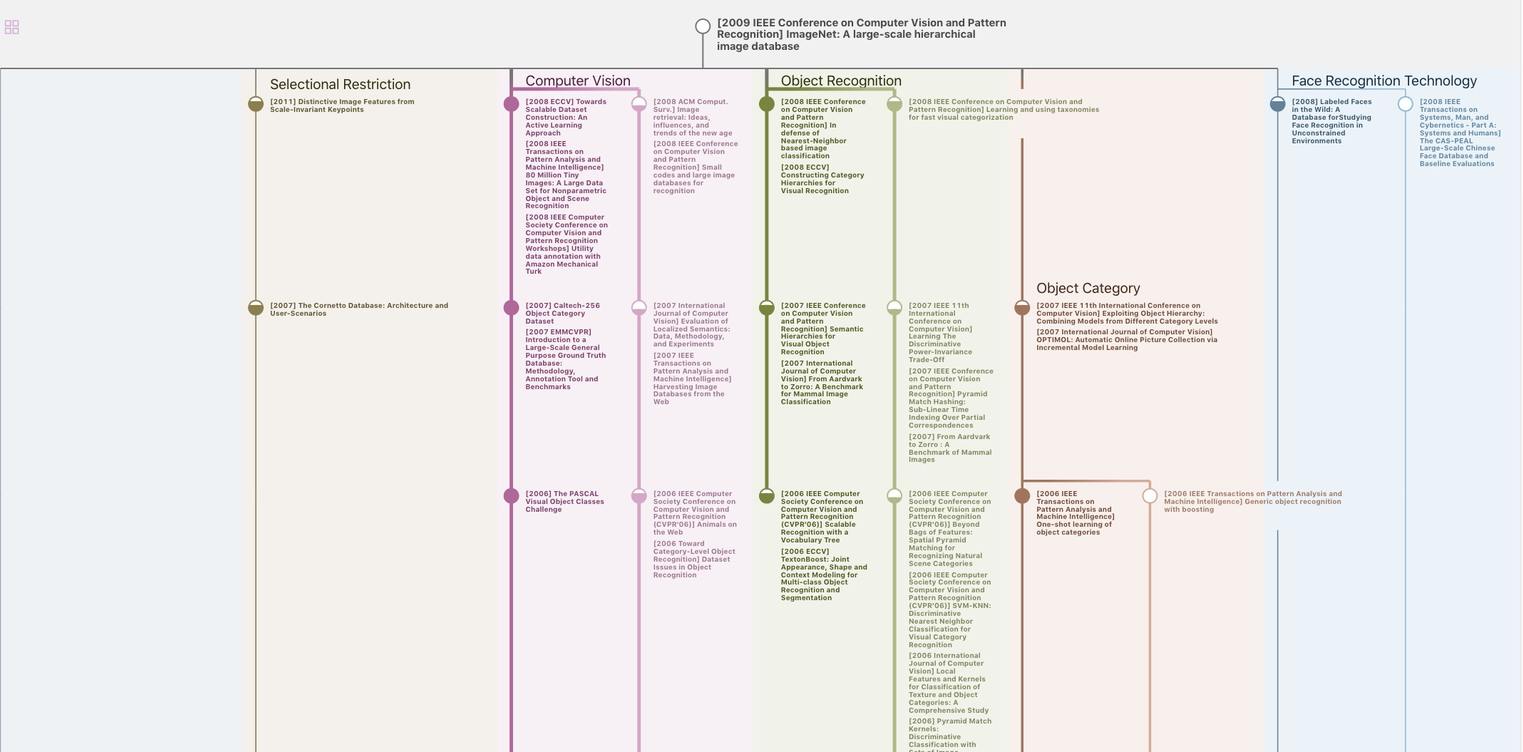
生成溯源树,研究论文发展脉络
Chat Paper
正在生成论文摘要