Small-Sample-Learning-Based Lithium-Ion Batteries Health Assessment: An Optimized Ensemble Framework
IEEE Transactions on Industry Applications(2024)
摘要
Machine Learning is widely studied in battery state of health (SOH) estimation due to its advantage in establishing the non-linear mapping between measurements and SOH. However, the requirement of a big dataset and the lack of robustness limit the practical application, especially in small sample learning. To tackle these challenges, an optimal ensemble framework called BaggELM (bagging extreme learning machine) is proposed for battery SOH estimation. Specifically, the required dataset is reduced by optimizing the input voltage and the hyperparameters of the BaggELM algorithm. Moreover, a statistical post-processing method is used to aggregate multiple ELMs, and the final estimate is determined by the maximum probability density value. As a result, the effects of random parameterization of ELM and the training data size on SOH estimation are suppressed, thus improving the robustness and accuracy of the conventional BaggELM. Compared to other classic machine learning methods, the proposed method reduces the required data size, maintains strong robustness and high estimation accuracy, making it a promising solution for small-sample-based SOH estimation. Finally, the effectiveness of the proposed estimation framework is verified using the accelerated aging dataset from Lithium Iron Phosphate (LFP) and Nickel Manganese Cobalt (NMC) batteries.
更多查看译文
关键词
Ensemble learning,health assessment,lithium-ion batteries,post-processing,small sample learning
AI 理解论文
溯源树
样例
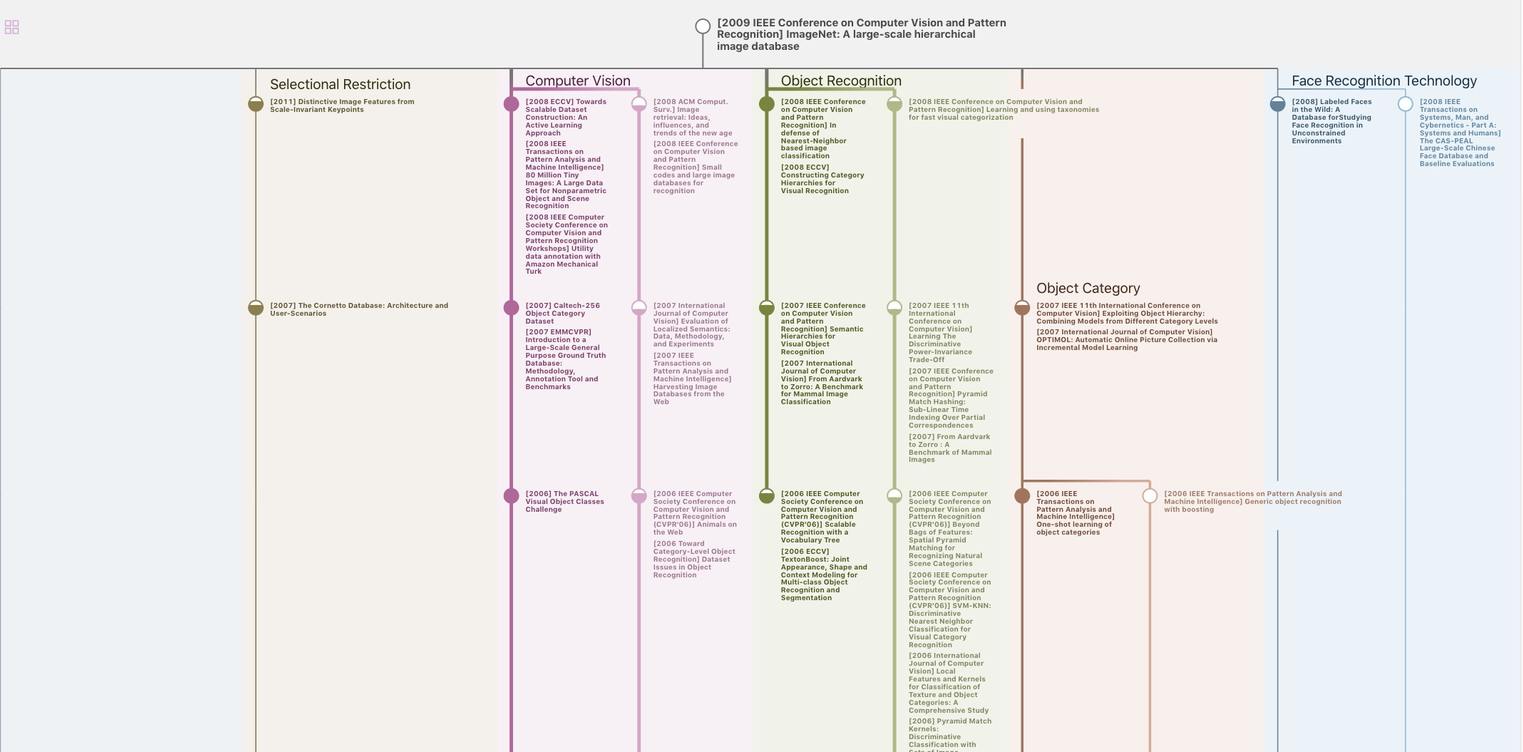
生成溯源树,研究论文发展脉络
Chat Paper
正在生成论文摘要