S $^{3}$ M-Net: Joint Learning of Semantic Segmentation and Stereo Matching for Autonomous Driving
IEEE Transactions on Intelligent Vehicles(2024)
摘要
Semantic segmentation and stereo matching are two essential components of 3D environmental perception systems for autonomous driving. Nevertheless, conventional approaches often address these two problems independently, employing separate models for each task. This approach poses practical limitations in real-world scenarios, particularly when computational resources are scarce or real-time performance is imperative. Hence, in this article, we introduce S
$^{3}$
M-Net, a novel joint learning framework developed to perform semantic segmentation and stereo matching simultaneously. Specifically, S
$^{3}$
M-Net shares the features extracted from RGB images between both tasks, resulting in an improved overall scene understanding capability. This feature sharing process is realized using a feature fusion adaption (FFA) module, which effectively transforms the shared features into semantic space and subsequently fuses them with the encoded disparity features. The entire joint learning framework is trained by minimizing a novel semantic consistency-guided (SCG) loss, which places emphasis on the structural consistency in both tasks. Extensive experimental results conducted on the vKITTI2 and KITTI datasets demonstrate the effectiveness of our proposed joint learning framework and its superior performance compared to other state-of-the-art single-task networks. Our project webpage is accessible at mias.group/S3M-Net.
更多查看译文
关键词
semantic segmentation,stereo matching,environmental perception,autonomous driving,joint learning
AI 理解论文
溯源树
样例
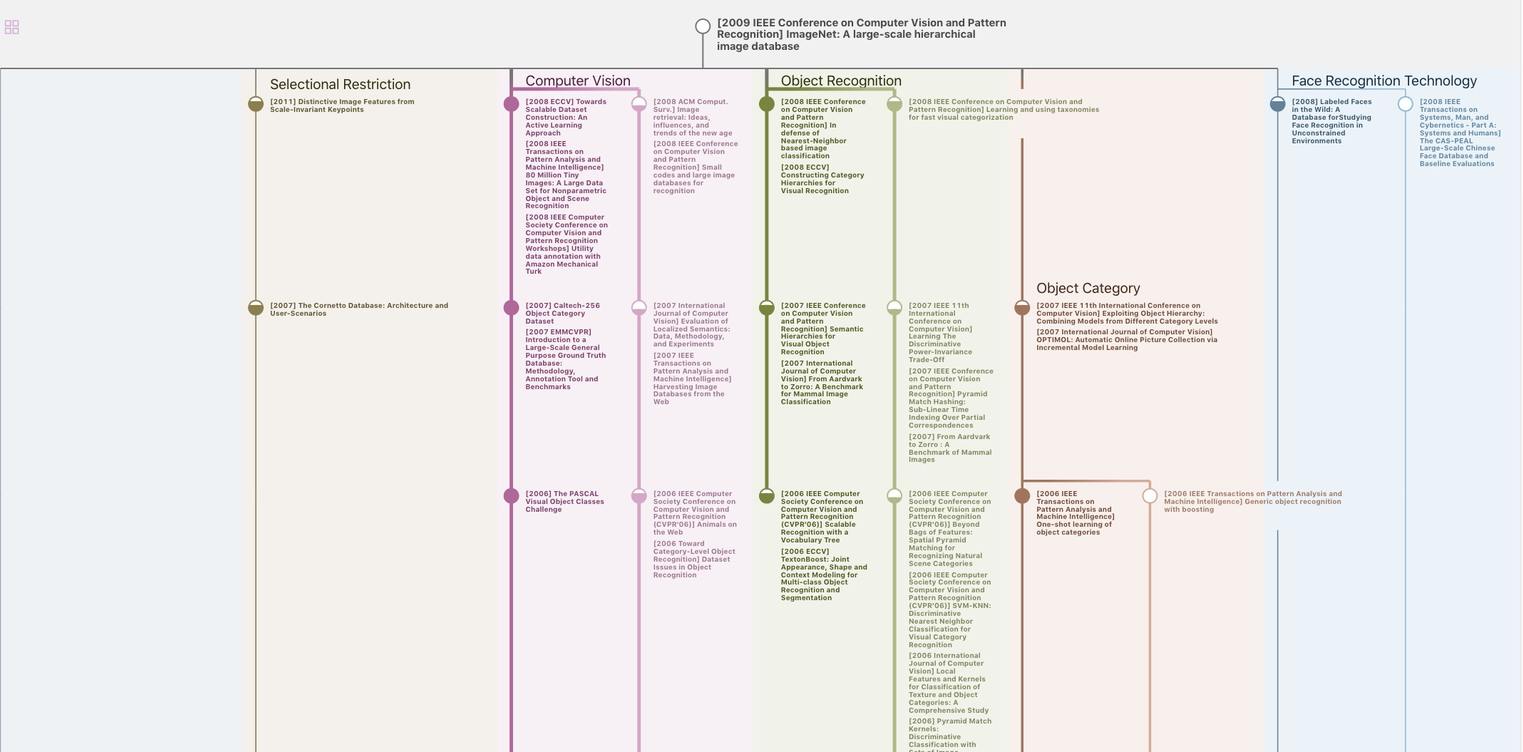
生成溯源树,研究论文发展脉络
Chat Paper
正在生成论文摘要