A Black-Box Attack Algorithm Targeting Unlabeled Industrial AI Systems With Contrastive Learning
IEEE TRANSACTIONS ON INDUSTRIAL INFORMATICS(2024)
摘要
Adversarial attack algorithms are useful for testing and improving the robustness of industrial AI models. However, attacking black-box models with limited queries and unknown real labels remains a significant challenge. To overcome this challenge, we propose using contrastive learning to train a generated substitute model called attack contrastive learning network (ACL-Net) to attack black-box models with very few queries and no real labels. ACL-Net achieves end-to-end contrastive learning during training without labels, which differs from previous contrastive learning methods that required separate training for the classification layer with labels. We improve ACL-Net's robustness by using adversarial examples to train it during the attack stage. This approach results in more effective adversarial examples generated by ACL-Net. We conducted extensive experiments to validate the effectiveness of ACL-Net. Compared with the latest algorithms, ACL-Net requires fewer queries to achieve better attack performance, demonstrating its superiority in query-efficient black-box attacks. Overall, our approach presents a promising solution to the challenge of attacking black-box models with limited queries and unknown real labels. Our results show the effectiveness of using contrastive learning to train generated substitute models, and the potential for improving the robustness of industrial AI models through adversarial attacks.
更多查看译文
关键词
Adversarial examples,contrastive learning (CL),industrial AI models,limited queries,robustness
AI 理解论文
溯源树
样例
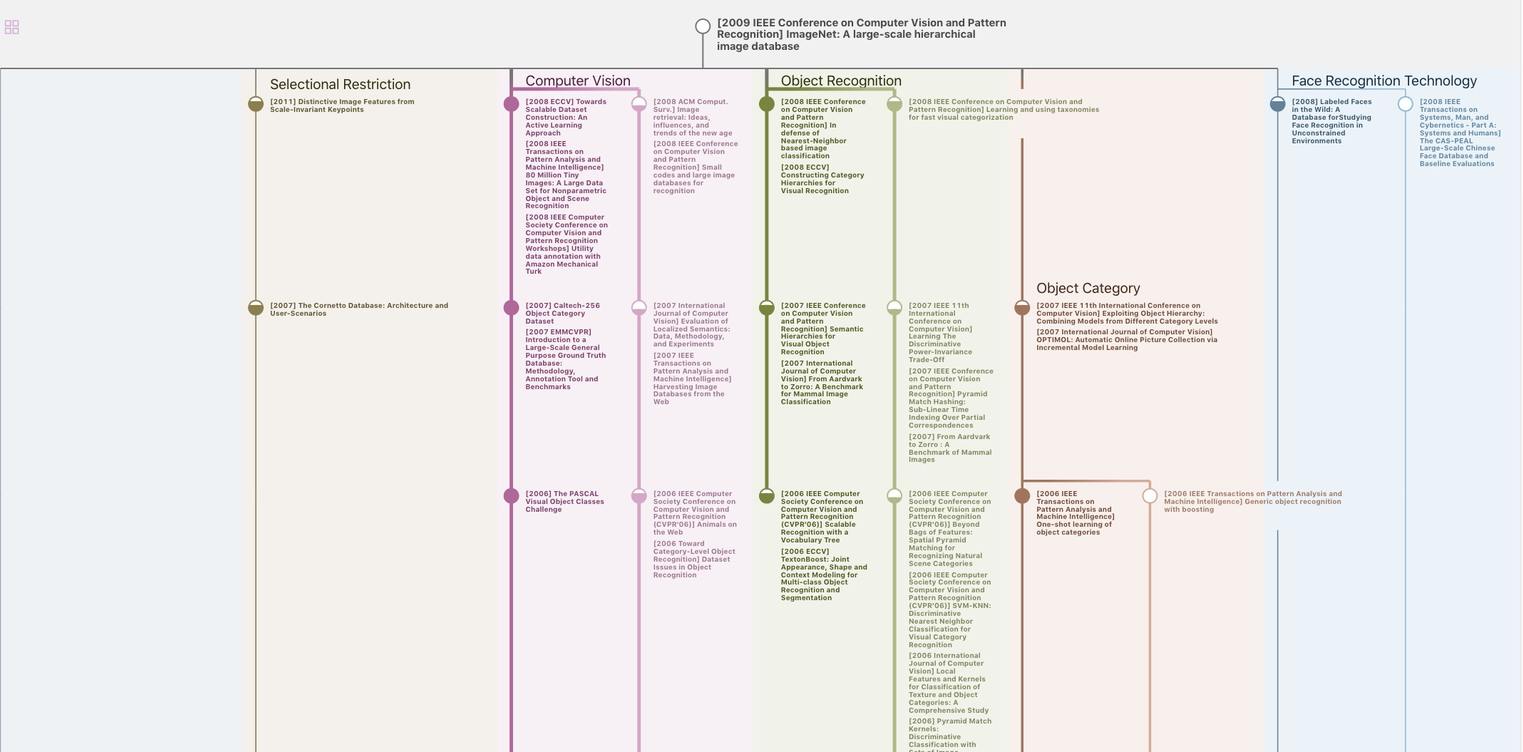
生成溯源树,研究论文发展脉络
Chat Paper
正在生成论文摘要