Support-Sample-Assisted Domain Generalization via Attacks and Defenses: Concepts, Algorithms, and Applications to Pipeline Fault Diagnosis
IEEE TRANSACTIONS ON INDUSTRIAL INFORMATICS(2024)
摘要
This article is concerned with domain generalization (DG), a practical yet challenging scenario in transfer learning where the target data are not available in advance. The key insight of DG is focused on learning a robust model that can generalize to the unseen domain by leveraging knowledge from the source domain. To this end, we propose a novel algorithm known as support-sample-assisted Adversarial Attacks (SSAA) for DG. In the SSAA algorithm, an attack-defense strategy is deployed to enhance the target model's generalizability and transferability. This strategy includes a nontargeted attack stage, during which attack samples are generated to form pseudotarget domains with near-realistic covariate shifts. Subsequently, in the model defense stage, a biclassifier structure is used to distinguish support samples from the generated attack samples. These support samples form a new decision boundary encompassing all unseen samples, prompting an extension of the existing decision boundary to meet these samples. Experimental results on cross-domain fault diagnosis tasks suggest that SSAA outperforms current state-of-the-art DG methods, indicating a promising avenue for further DG development.
更多查看译文
关键词
Feature extraction,Training,Task analysis,Generators,Perturbation methods,Pipelines,Informatics,Attack-defense strategy,domain adaptation (DA),domain generalization (DG),support sample,transfer learning (TL)
AI 理解论文
溯源树
样例
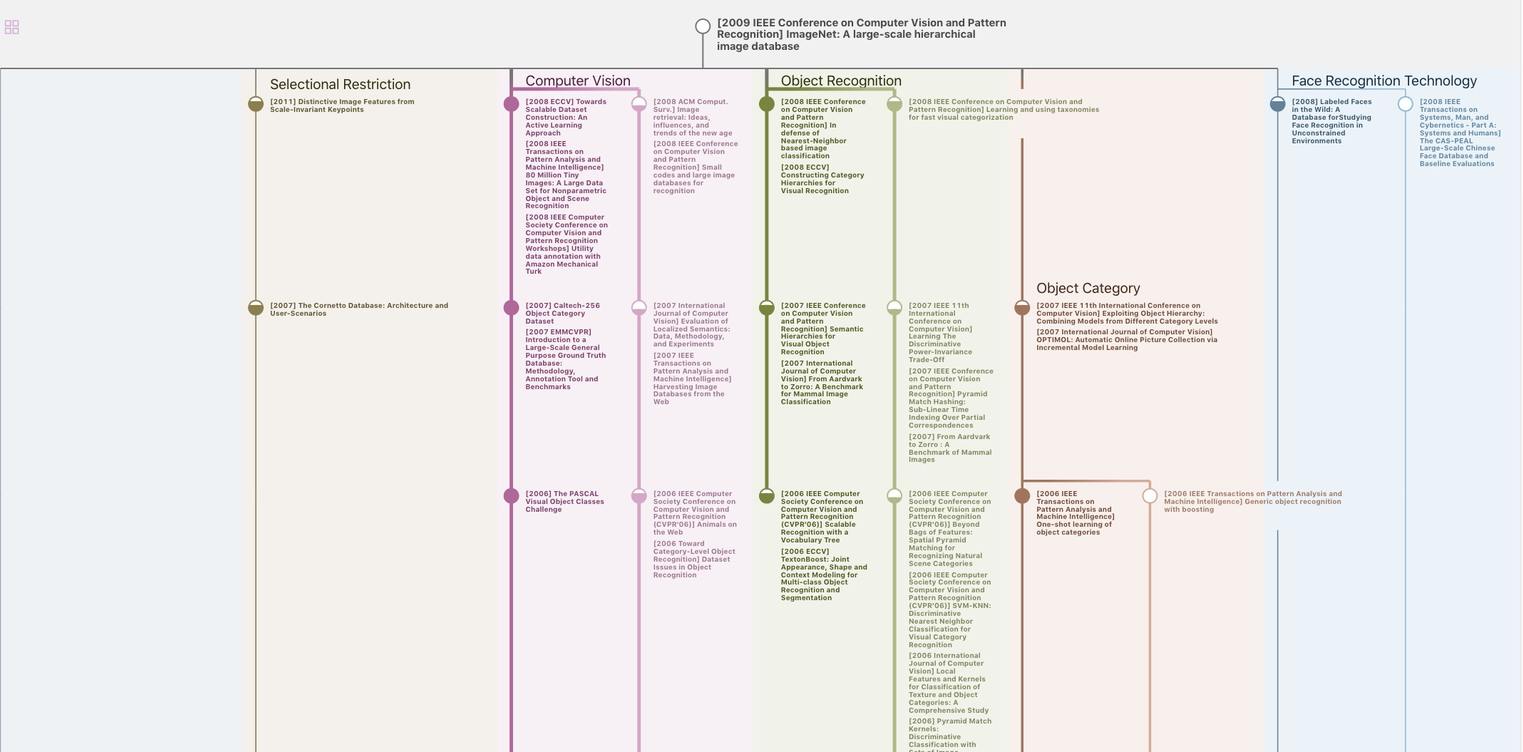
生成溯源树,研究论文发展脉络
Chat Paper
正在生成论文摘要