Class-Aware Adversarial Multiwavelet Convolutional Neural Network for Cross-Domain Fault Diagnosis
IEEE TRANSACTIONS ON INDUSTRIAL INFORMATICS(2024)
摘要
Incomplete feature extraction and underutilization of unlabeled target data exist in the actual situation of rotating machinery fault diagnosis. To this end, a class-aware adversarial multiwavelet convolutional neural network (CAMCNN) is developed for cross-domain fault diagnosis of rotating machinery. Specifically, a class-aware classification mechanism (CCM) is first designed to autonomously sense the prediction effect of the target samples and improve the discrimination of classifiers. Thereafter, a class-aware adversarial domain adaptation approach based on CCM is proposed to precisely align the domain features at the class level, with the help of the developed reinforced conditional distribution alignment strategy. Finally, multiple wavelet convolutional kernels are introduced to replace the conventional convolutional kernel, and a multiwavelet convolutional neural network is constructed as the feature extractor to distill the implied feature information. Numerous results verify the validity of CAMCNN, while comparison results with mainstream methods demonstrate the superiority of CAMCNN.
更多查看译文
关键词
Class-aware adversarial domain adaptation,class-aware classification mechanism,multiwavelet convolutional neural network,reinforced conditional distribution alignment strategy,rotating machinery fault diagnosis
AI 理解论文
溯源树
样例
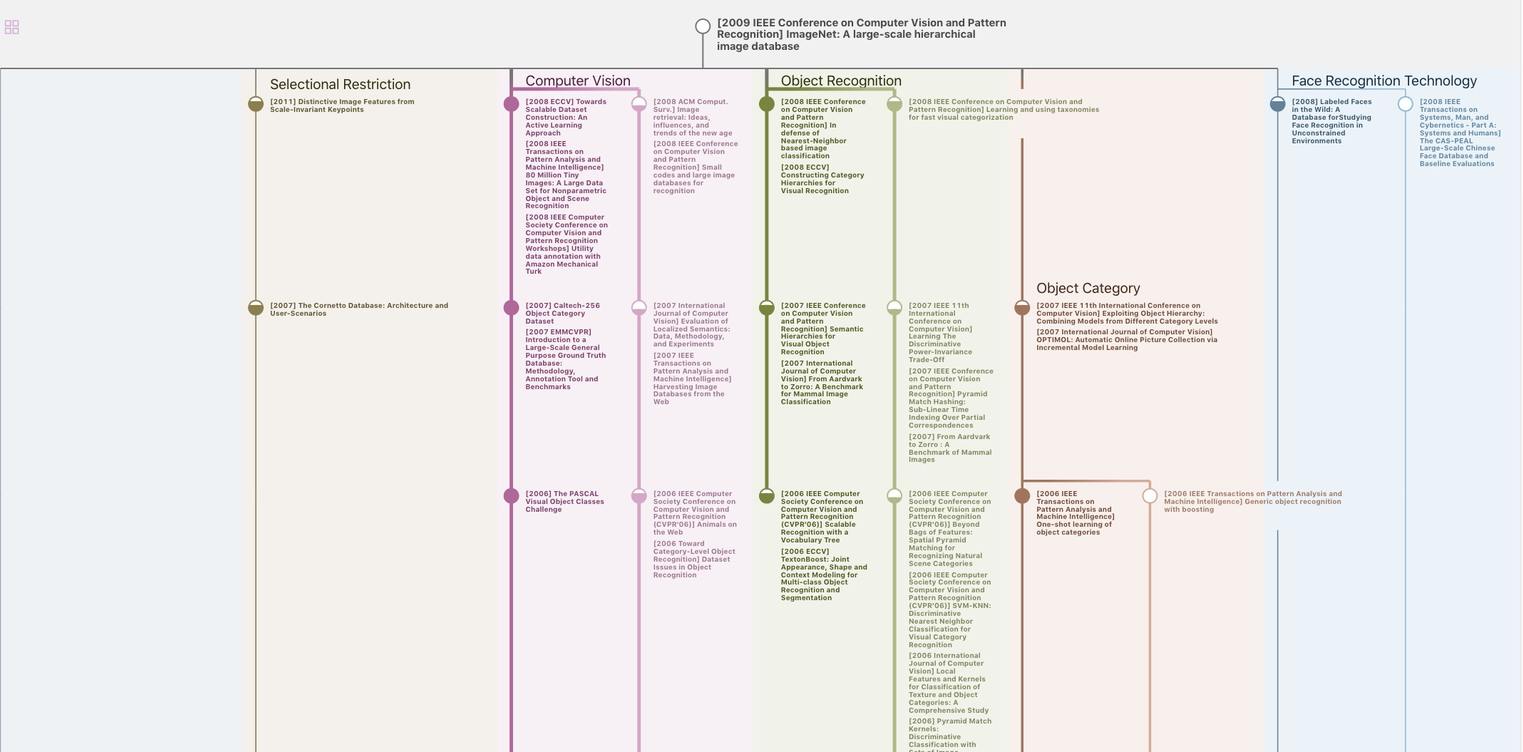
生成溯源树,研究论文发展脉络
Chat Paper
正在生成论文摘要