MC-Net: Realistic Sample Generation for Black-Box Attacks.
IEEE Trans. Inf. Forensics Secur.(2024)
摘要
One area of current research on adversarial attacks is how to generate plausible adversarial examples when only a small number of datasets are available. Current adversarial attack algorithms used to attack these black-box systems face a number of challenges, such as difficulty in training convergence, ambiguous sample images, substitute models collapse, unsatisfactory attack success rates, high query cost, and low defense capability improvement of target models. As a result, constructing plausible adversarial situations in a few known real-world sample circumstances remains difficult. As a solution to the aforementioned issues, this study introduces MC-Net, a novel multi-stage and multi-class balanced generating method based on a limited number of samples to generate realistic adversarial examples. Firstly, a multi-task learning approach is used to train the GAN by fully utilizing the small samples, ensuring that the size of the generated dataset for each category is balanced. In addition, we design a weight-balancing strategy to ensure faster convergence of each sub-network. Then, in the second stage, the generated samples of different categories are used to train a substitute model, and the distillation method is adopted to learn the output distribution of the target model. Finally, adversarial examples are constructed on the generated samples to complete the attack on the target models. Extensive experiments have proven that MC-Net has the following advantages: 1) The substitute model converges quickly using limited samples and queries; 2) High attack success rates can be obtained with a few queries; and 3) The constructed adversarial examples significantly improve the target model’s defense. Furthermore, we only utilize a few queries for the Microsoft Azure online model to obtain a satisfactory result. Our code can be found at https://github.com/jiaokailun/A-fast.
更多查看译文
关键词
Adversarial Attacks,Multi-class,Generating Method,Limited Samples
AI 理解论文
溯源树
样例
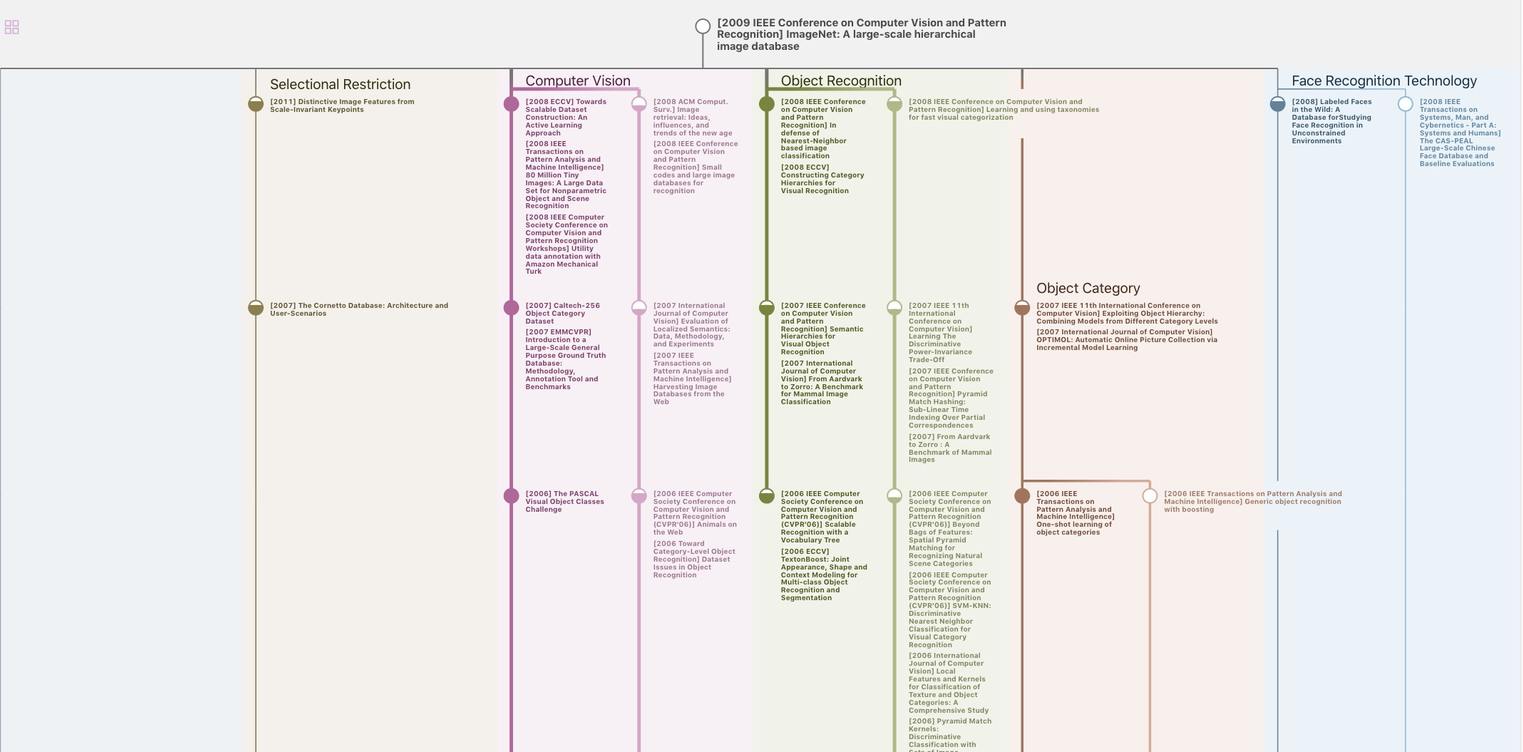
生成溯源树,研究论文发展脉络
Chat Paper
正在生成论文摘要