A Self-Supplementary and Revised Network for Remote Sensing Object Detection
IEEE GEOSCIENCE AND REMOTE SENSING LETTERS(2024)
摘要
Object detection is an essential and crucial task in interpretation of optical remote sensing images (RSIs). However, its performance is usually limited due to the complex background and multiscale characteristics of targets. To overcome these limitations, a self-supplementary and revised anchor-free detector is proposed. First, to reduce the computational cost of detection, a partial bottleneck (PBottleneck) structure is designed to efficiently extract multiscale feature information in a lightweight manner. Second, pure spatial feature pyramid network (PSFPN) attaches importance to description of distance and suppresses environmental disturbance by a devised multidirectional distance attention (MDDA) mechanism. In addition, pure fusion strategy (PFS) is created to boost information with no occlusion between various features. Third, toward the multiscale objects issue, self-learning supplementary and revised module (SSRM) is explored to generate more abundant and balanced expression by adaptively incorporating the supplementary and corrected information from adjacent features. Finally, comprehensive experiments are conducted on several publicly available datasets, demonstrating effectiveness of our proposed detector, leading to a new benchmark.
更多查看译文
关键词
Feature extraction,Semantics,Remote sensing,Convolution,Interference,Data mining,YOLO,Anchor-free,multiscale fusion,object detection,remote sensing
AI 理解论文
溯源树
样例
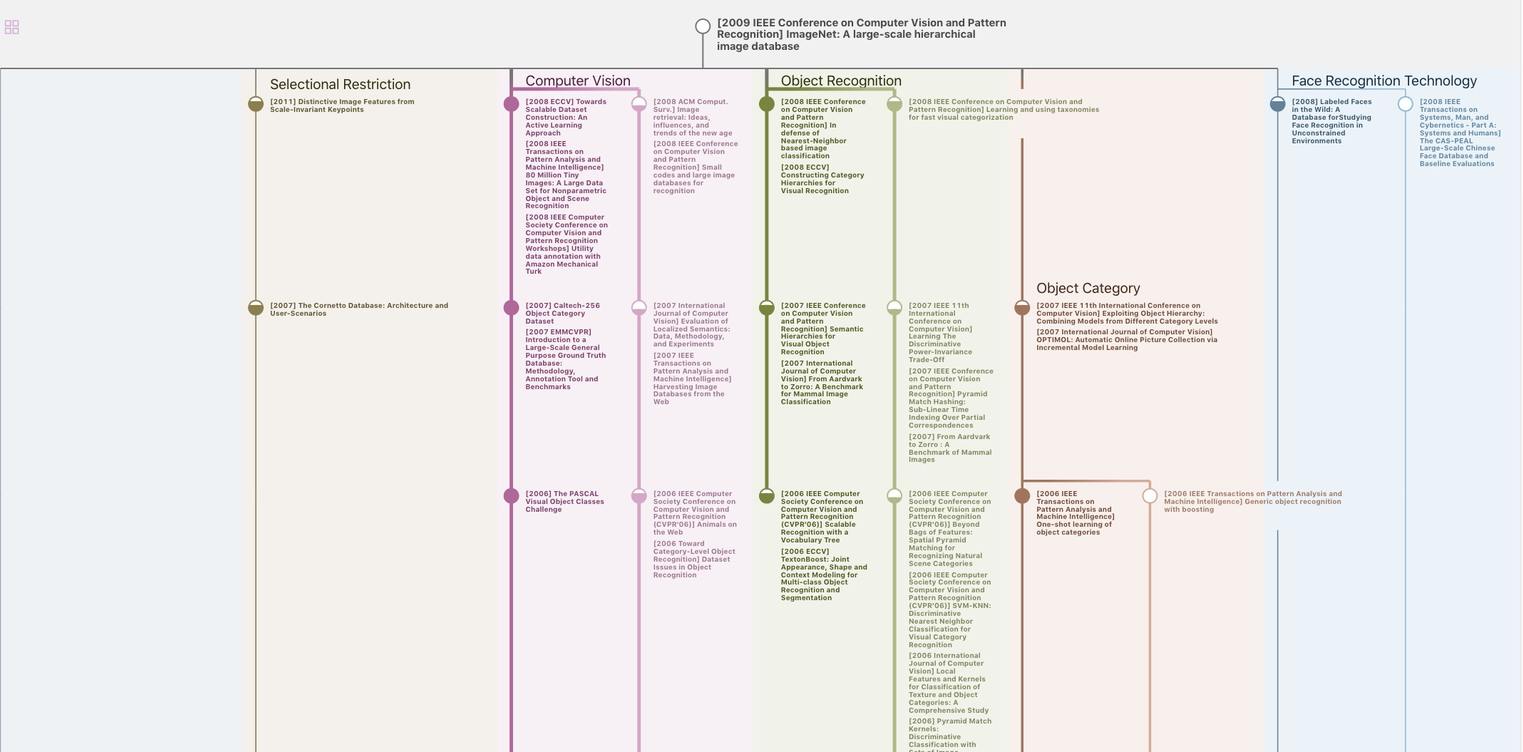
生成溯源树,研究论文发展脉络
Chat Paper
正在生成论文摘要