Feature Selection Graph Neural Network for Optimized Node Categorization.
IEEE Trans. Consumer Electron.(2024)
摘要
Graph Neural Networks (GNNs) is one of the most essential tools for learning from graph-structured data. A wide range of tasks has demonstrated their usefulness in graph-structured data. Engineering configuration has progressed fundamentally, further developing execution on different forecast errands. Utilizing learnable weight matrices, these neural networks typically incorporate feature transformation and node feature aggregation in the same layer. The articulateness of the layers of neural network and the significance of node information gathered from various hops are complicated to analyze. Because diverse graph datasets exhibit changeable degrees of heterophily and homophily in class label dissemination, it is necessary to recognize which features are crucial for the forecast tasks without preceding knowledge. The proposed work focus on demonstrating that the GNN model’s efficiency can be harmed by frequently employing less informative features and that not all aggregation process features are beneficial. The experimental outcomes validated that the performance can be enhanced on a wide range of datasets by learning specific subsets of these features. Based on the observations, several significant design concepts for neural graph networks are being introduce. More specifically, L2-Normalization is employed over GNN layers using SoftMax as a regularize and a “soft-selector” of characteristics gathered from neighbors at various hop distances. The Feature Selection Graph Neural Network (FSGNN), a straightforward and shallow model, is framed by combining these approaches. Nine standard datasets for the node classification task empirically exhibit that the proposed model outperforms current GNN models, with significant improvements of up to 50.80%.
更多查看译文
关键词
Graph neural network,feature selection,neural network,node classification
AI 理解论文
溯源树
样例
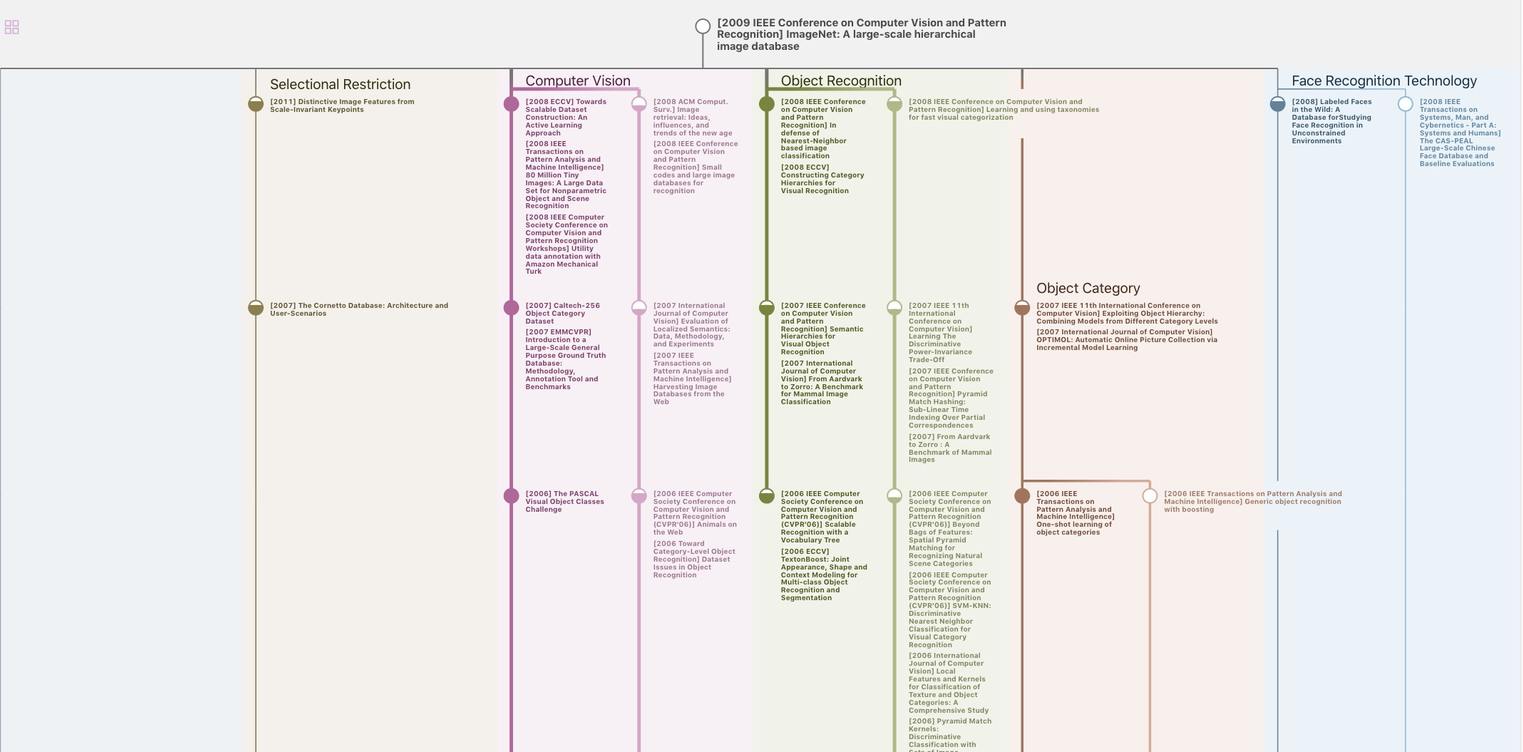
生成溯源树,研究论文发展脉络
Chat Paper
正在生成论文摘要