MedNAS: Multi-Scale Training-Free Neural Architecture Search for Medical Image Analysis
IEEE Transactions on Evolutionary Computation(2024)
摘要
Deep neural networks have demonstrated impressive results in medical image analysis, but designing suitable architectures for each specific task is expertise-dependent and time-consuming. Neural architecture search (NAS) offers an effective means of discovering architectures. It has been highly successful in numerous applications, particularly in natural image classification. Yet, medical images possess unique characteristics, such as small regions and a wide variety of lesion sizes, that differentiate them from natural images. Furthermore, most current NAS methods struggle with high computational costs, especially when dealing with high-resolution image datasets. In this paper, we present a novel evolutionary neural architecture search method called Multi-Scale Training-Free Neural Architecture Search to address these challenges. Specifically, to accommodate the broad range of lesion region sizes in disease diagnosis, we develop a new reduction cell search space that enables the search algorithm to explicitly identify the optimal scale combination for multi-scale feature extraction. To overcome the issue of high computational costs, we utilize training-free indicators as performance measures for candidate architectures, which allows us to search for the optimal architecture more efficiently. More specifically, by considering the capability and simplicity of various networks, we formulate a multi-objective optimization problem that involves two training-free indicators and model complexity for candidate architectures. Extensive experiments on a large medical image benchmark and a publicly available breast cancer detection dataset are conducted. The empirical results demonstrate that our MSTF-NAS outperforms both human-designed architectures and current state-of-the-art NAS algorithms on both datasets, indicating the effectiveness of our proposed method.
更多查看译文
关键词
Neural architecture search,evolutionary optimization,training-free architecture search,medical image classification
AI 理解论文
溯源树
样例
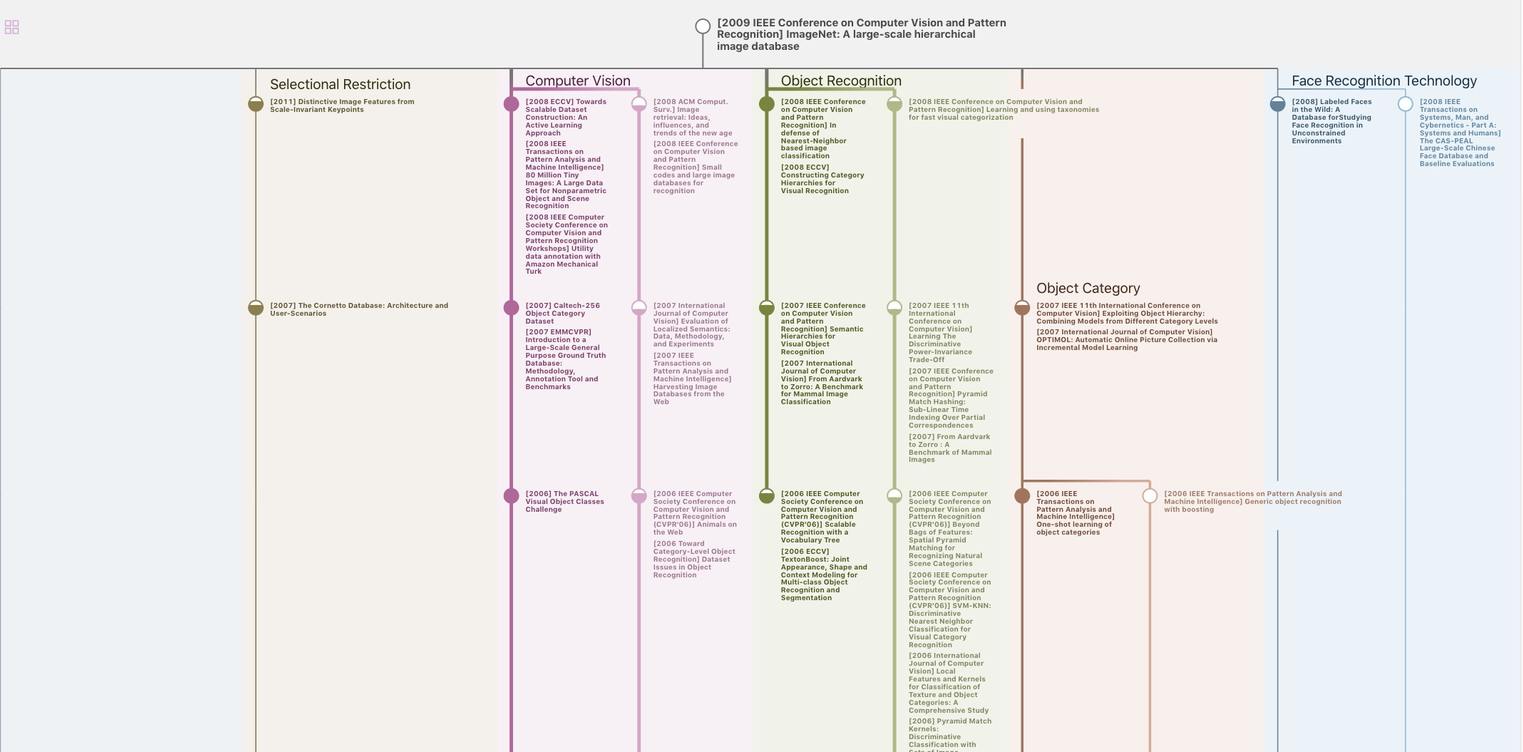
生成溯源树,研究论文发展脉络
Chat Paper
正在生成论文摘要