A Flexible Ranking-Based Competitive Swarm Optimizer for Large-Scale Continuous Multi-Objective Optimization
IEEE Transactions on Evolutionary Computation(2024)
摘要
Due to the curse of dimensionality, the search efficiency of existing operators in large-scale decision space deteriorates dramatically. The competitive swarm optimizer-based framework has great potential in tackling large-scale single-objective optimization problems. However, the existing competitive swarm optimizers only focus on the loser to winner learning paradigm and neglect the significance of the winner determination mechanism for large-scale search, which makes the algorithm difficult to escape from local optima. To remedy this issue, a flexible ranking-based competitive swarm optimizer has been tailored for handling large-scale multi-objective optimization problems (MOPs). Concretely, a novel winner determination strategy is introduced to broadly identify high-quality individuals in the population to enhance diversity maintenance. In addition, a special competitive mechanism is adopted to guide the search direction, which is capable of efficiently increasing search space utilization. The simulation results validate that the proposed algorithm can significantly enhance the exploration and exploitation ability of the conventional competitive swarm optimizer, and outperforms several state-of-the-art large-scale multi-objective optimization algorithms on both large-scale benchmark MOPs and application examples.
更多查看译文
关键词
Competitive swarm optimizer,large-scale multi-objective optimization,search paradigm
AI 理解论文
溯源树
样例
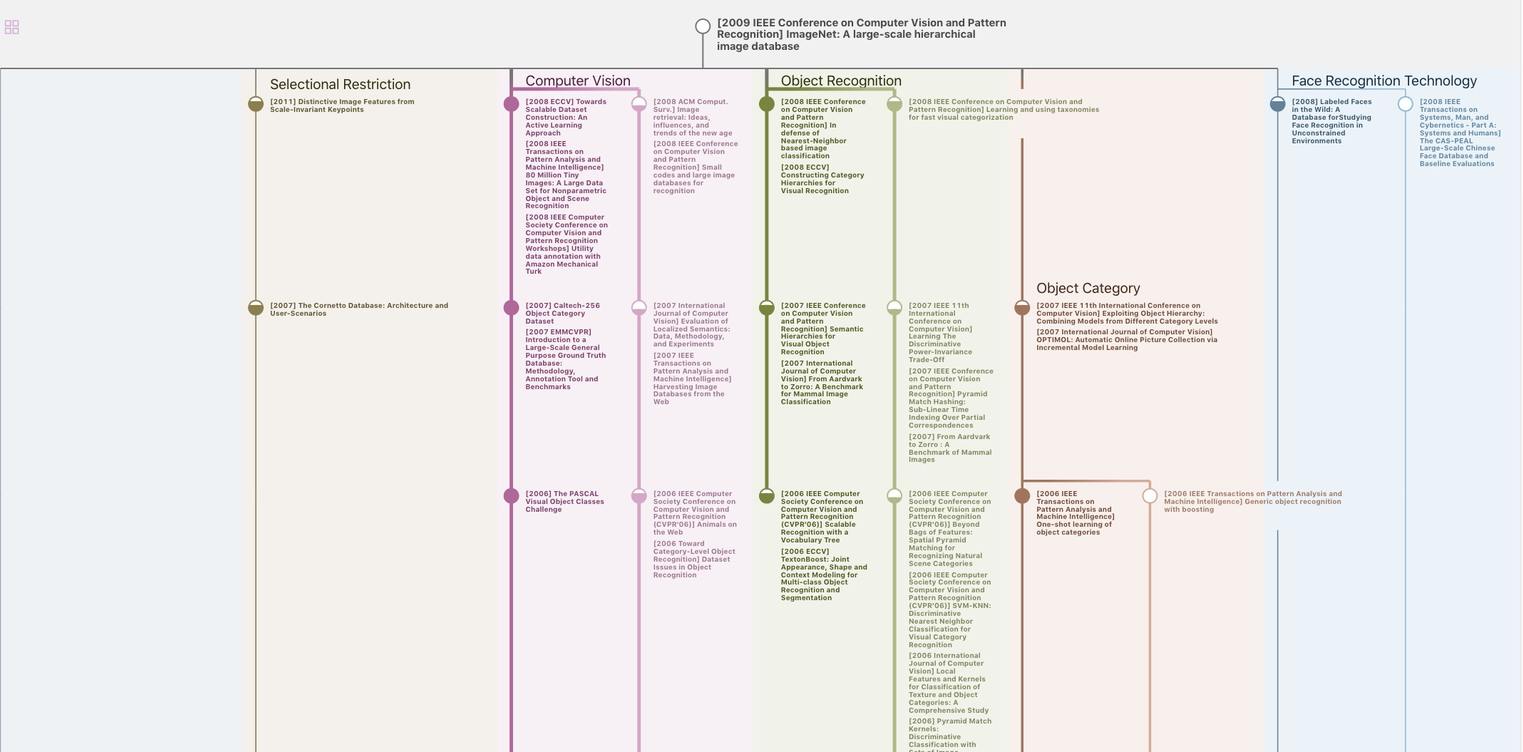
生成溯源树,研究论文发展脉络
Chat Paper
正在生成论文摘要